Learning Regularized Positional Encoding for Molecular Prediction
arxiv(2022)
摘要
Machine learning has become a promising approach for molecular modeling. Positional quantities, such as interatomic distances and bond angles, play a crucial role in molecule physics. The existing works rely on careful manual design of their representation. To model the complex nonlinearity in predicting molecular properties in an more end-to-end approach, we propose to encode the positional quantities with a learnable embedding that is continuous and differentiable. A regularization technique is employed to encourage embedding smoothness along the physical dimension. We experiment with a variety of molecular property and force field prediction tasks. Improved performance is observed for three different model architectures after plugging in the proposed positional encoding method. In addition, the learned positional encoding allows easier physics-based interpretation. We observe that tasks of similar physics have the similar learned positional encoding.
更多查看译文
关键词
positional encoding,molecular,prediction,learning
AI 理解论文
溯源树
样例
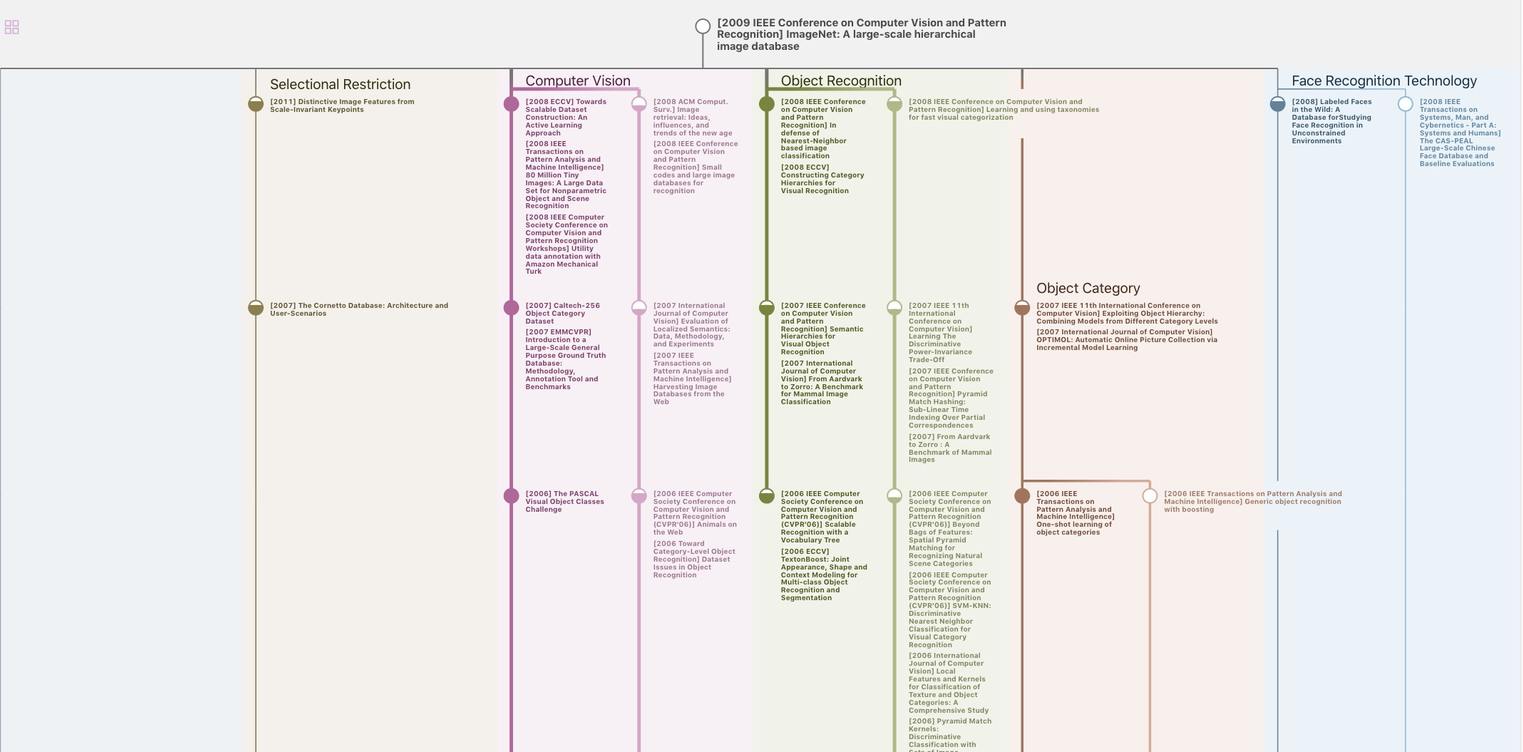
生成溯源树,研究论文发展脉络
Chat Paper
正在生成论文摘要