Including Transfer Learning and Synthetic Data in a Training Process of a 2D Object Detector for Autonomous Driving
ROBOT2022: Fifth Iberian Robotics Conference(2022)
摘要
Nowadays the use of deep learning (DL) based systems is widely extended in several areas such as facial recognition, voice and audio processing or perception systems. The training process that must be performed for proper functionality requires a large amount of data with the required characteristics needed for the task to be executed. The process of obtaining new adequate training data is complex and tedious, therefore multiple techniques such as data augmentation or transfer learning have been developed in order to have a greater amount of knowledge in the network without the need to search for new data sources. The aim of this paper is to study the effect of the inclusion of knowledge from transfer learning in a 2D image detector trained with real world and synthetic data from multiple sources. The detector that is going to be trained will be focused on autonomous driving tasks, therefore we decide to use KITTI as the real world data source and our AD PerDevkit (based on CARLA) and Virtual-KITTI as the synthetic sources.
更多查看译文
关键词
Deep learning, Transfer learning, 2D object detection, Simulation, KITTI, CARLA
AI 理解论文
溯源树
样例
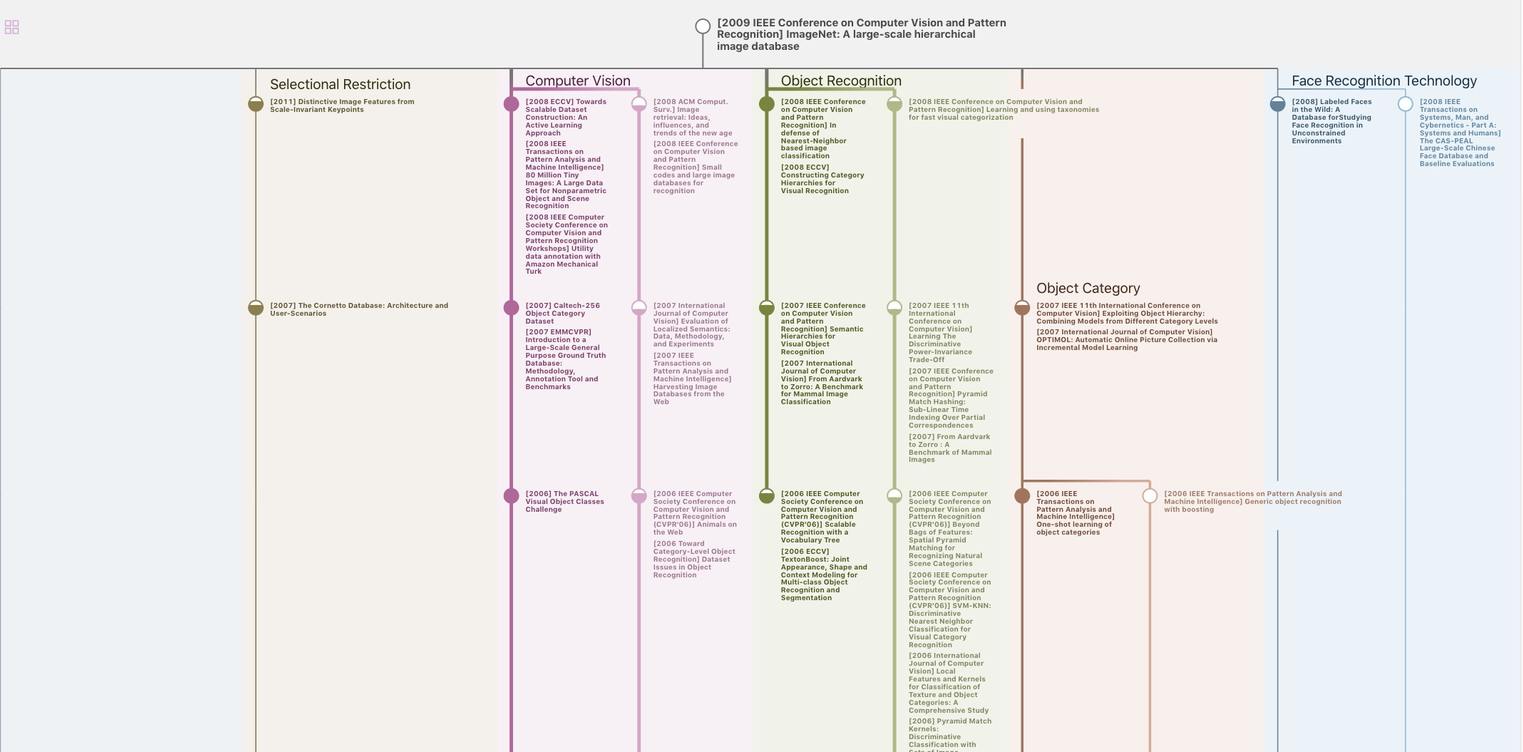
生成溯源树,研究论文发展脉络
Chat Paper
正在生成论文摘要