Exploring the Confounding Factors of Academic Career Success: An Empirical Study with Deep Predictive Modeling
2023 23RD IEEE INTERNATIONAL CONFERENCE ON DATA MINING WORKSHOPS, ICDMW 2023(2023)
摘要
Understanding determinants of success in academic careers is critically important to both scholars and their employing organizations. While considerable research efforts have been made in this direction, there is still a lack of a quantitative approach to modeling the academic careers of scholars due to the massive confounding factors. To this end, in this paper, we try to mine the key factors of academic career success through an empirical and predictive modeling perspective, with a focus on two typical career honors, i.e. IEEE Fellow and ACM Fellow. Specifically, we first analyze the candidate's nomination carefully and extract the probable factors of being a Fellow, e.g., scholarly productivity, scientific impact, gender, etc. Then for a scholar, we define the "scholarly distance" to measure the ability that he/she can recommend appropriate endorsers from existing Fellows as his/her nominators. Third, we propose both classification and regression models based on the same underlying neural network structure with self-attention mechanism, called Cls-Fellow and Reg-Fellow, to predict whether a candidate will be elected as a Fellow at current year and how many additional years it will take to be a Fellow. These two models could be helpful for scholars' self-assessment. Extensive experiments on two Fellow datasets (IEEE Fellow and ACM Fellow) show that our proposed models can achieve great performance. Finally, we analyze the importance of different factors quantitatively, and obtain some insightful findings, such as the evolution of co-author networks between candidates and Fellows, the inequality of gender. We hope these derived factors and findings can help the scholars to improve their competitiveness and develop well in their academic career.
更多查看译文
关键词
fellow election,scholarly productivity evaluation,coauthorship networks
AI 理解论文
溯源树
样例
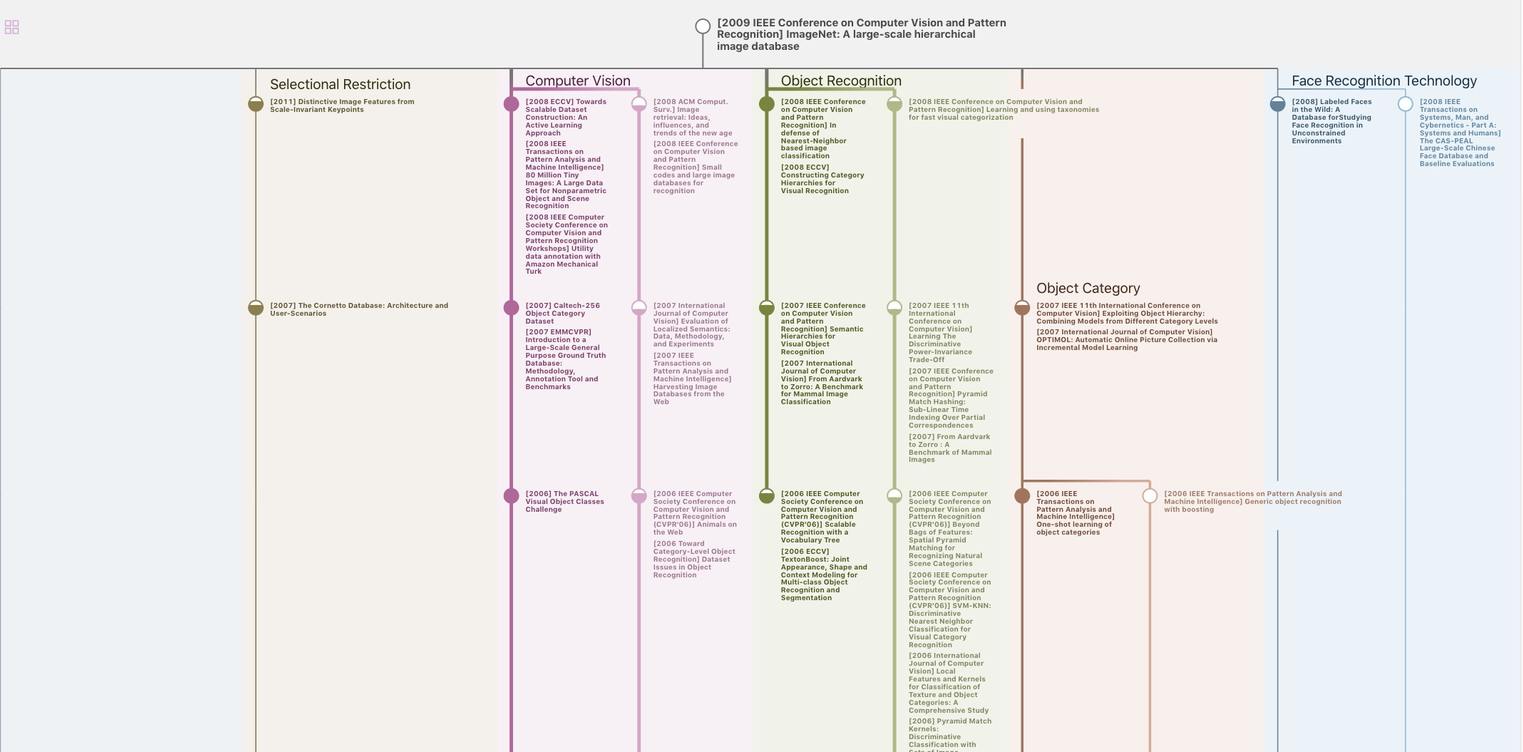
生成溯源树,研究论文发展脉络
Chat Paper
正在生成论文摘要