A Tale of Two Cities: Data and Configuration Variances in Robust Deep Learning
IEEE INTERNET COMPUTING(2023)
摘要
Deep neural networks (DNNs) have widespread applications in industries such as image recognition, supply chain, medical diagnosis, and autonomous driving. However, previous work has shown that the high accuracy of a DNN model does not imply high robustness (i.e., consistent performances on new and future datasets) because the input data and external environment (e.g., software and model configurations) for a deployed model are constantly changing. Therefore, ensuring robustness is crucial to enhance business and consumer confidence. Previous research focuses mostly on the data aspect of model variance. This article takes a holistic view of DNN robustness by summarizing the issues related to both data and software configuration variances. We also present a predictive framework using search-based optimization to generate representative variances for robust learning, considering data and configurations.
更多查看译文
关键词
Robustness,Data models,Perturbation methods,Training,Mathematical models,Forecasting,Predictive models
AI 理解论文
溯源树
样例
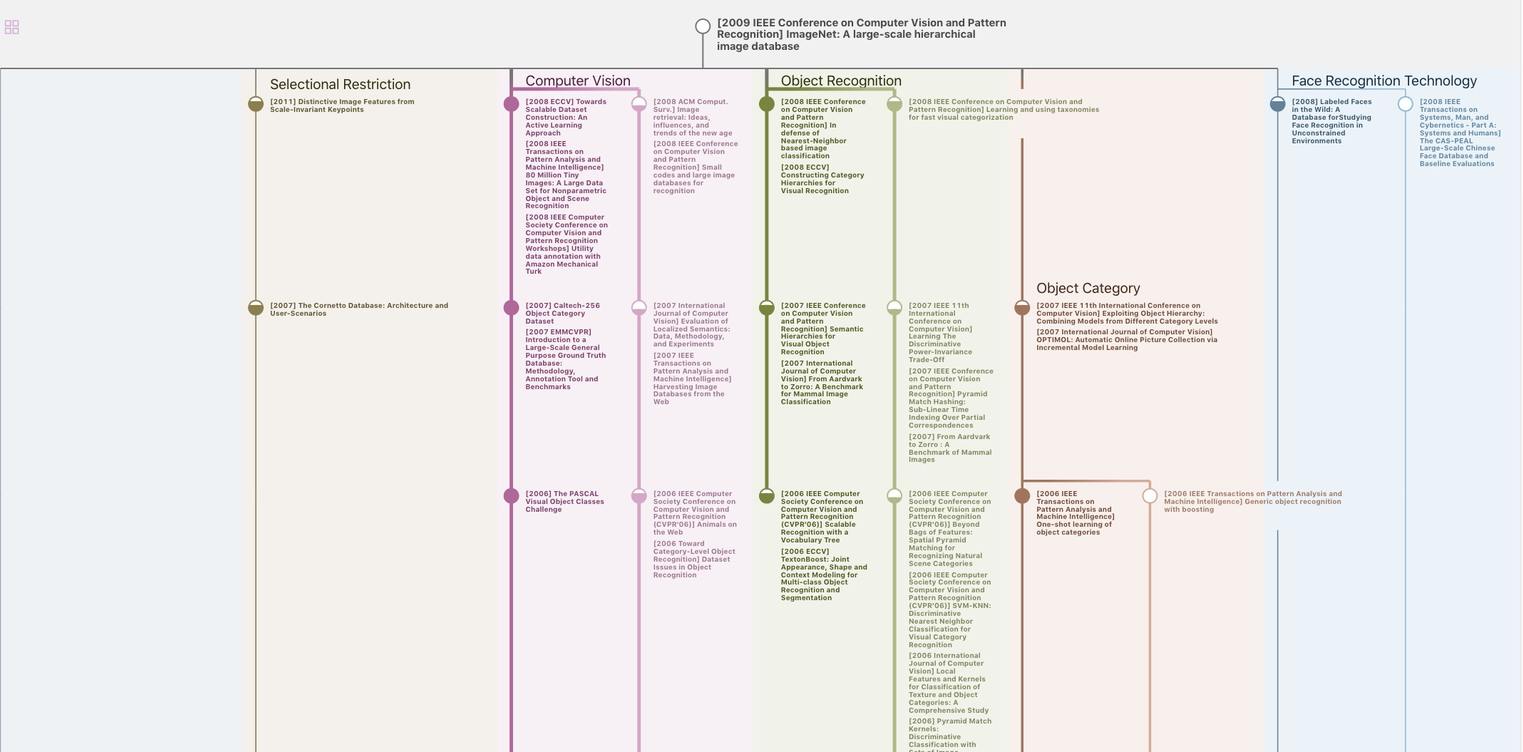
生成溯源树,研究论文发展脉络
Chat Paper
正在生成论文摘要