MelHuBERT: A simplified HuBERT on Mel spectrogram
arxiv(2022)
摘要
Self-supervised models have had great success in learning speech representations that can generalize to various downstream tasks. HuBERT, in particular, achieves strong performance while being relatively simple in training compared to others. The original experimental setting is computationally extensive, hindering the reproducibility of the models. It is also unclear why certain design decisions are made, such as the ad-hoc loss function, and whether these decisions have an impact on the learned representations. We propose MelHuBERT, a simplified version of HuBERT that takes Mel spectrograms as input, significantly reducing computation and memory consumption. We study several aspects of training, including the loss function, multi-stage training, and streaming options. Our result is a efficient yet performant model that can be trained on a single GPU.
更多查看译文
关键词
Self-Supervised Learning,Speech Representations,Automatic Speech Recognition,Speaker Recognition
AI 理解论文
溯源树
样例
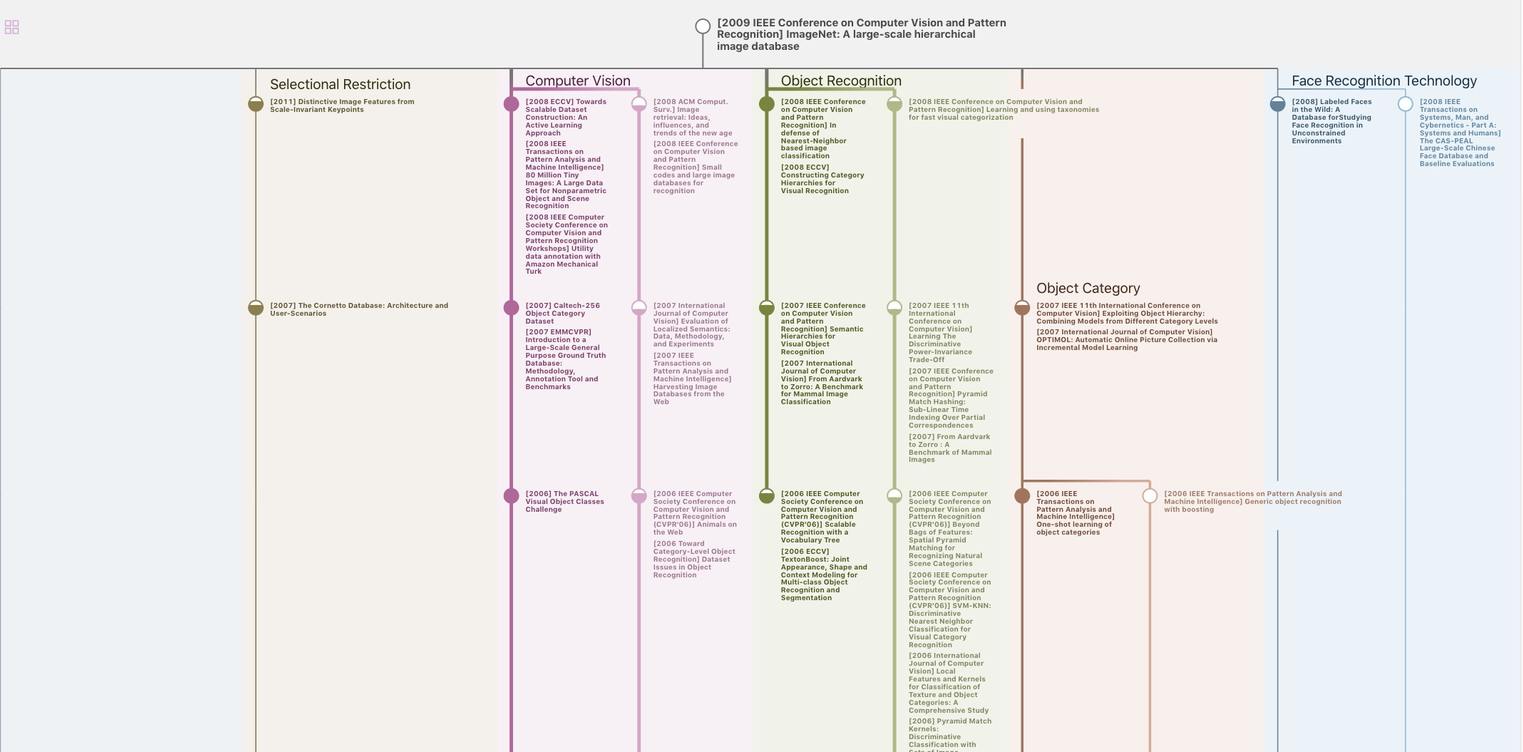
生成溯源树,研究论文发展脉络
Chat Paper
正在生成论文摘要