Predicting Hospitalization from Health Insurance Data
2022 IEEE International Conference on Systems, Man, and Cybernetics (SMC)(2022)
摘要
Hospitalizations represent an expressive part of total health costs and, therefore, reducing the number of hospitalizations, when possible, can generate both economic gains and enhanced quality of life of patients. Several works have been striving to use machine learning to create models for hospitalization predictions. Most of them require specialized knowledge in the health area, mainly in the stages of data preparation and selection of features. This feature engineering is not always perfect and may fail to select relevant features for the model training process. In this paper, to fill this gap, we explore three sources of information to extract features, i.e., medical specialty, event description, and the International Classification of Diseases. In addition, we introduce a dataset composed of 38,524 records of medical events from 34,930 patients. To assess and set a baseline for this new dataset, we have used two well-known ensemble methods (Random Forest and Gradient Boosting). The best results, AUC = 0.82, were achieved by combining the models generated from the three feature set tested and gradient boosting. We believe that researchers will find this dataset a valuable tool in their work on hospitalization prediction. It will also make future benchmarking and evaluation possible.
更多查看译文
关键词
Health Insurance,Hospitalization Prediction,Machine Learning,Gradient Boosting,Random Forest.
AI 理解论文
溯源树
样例
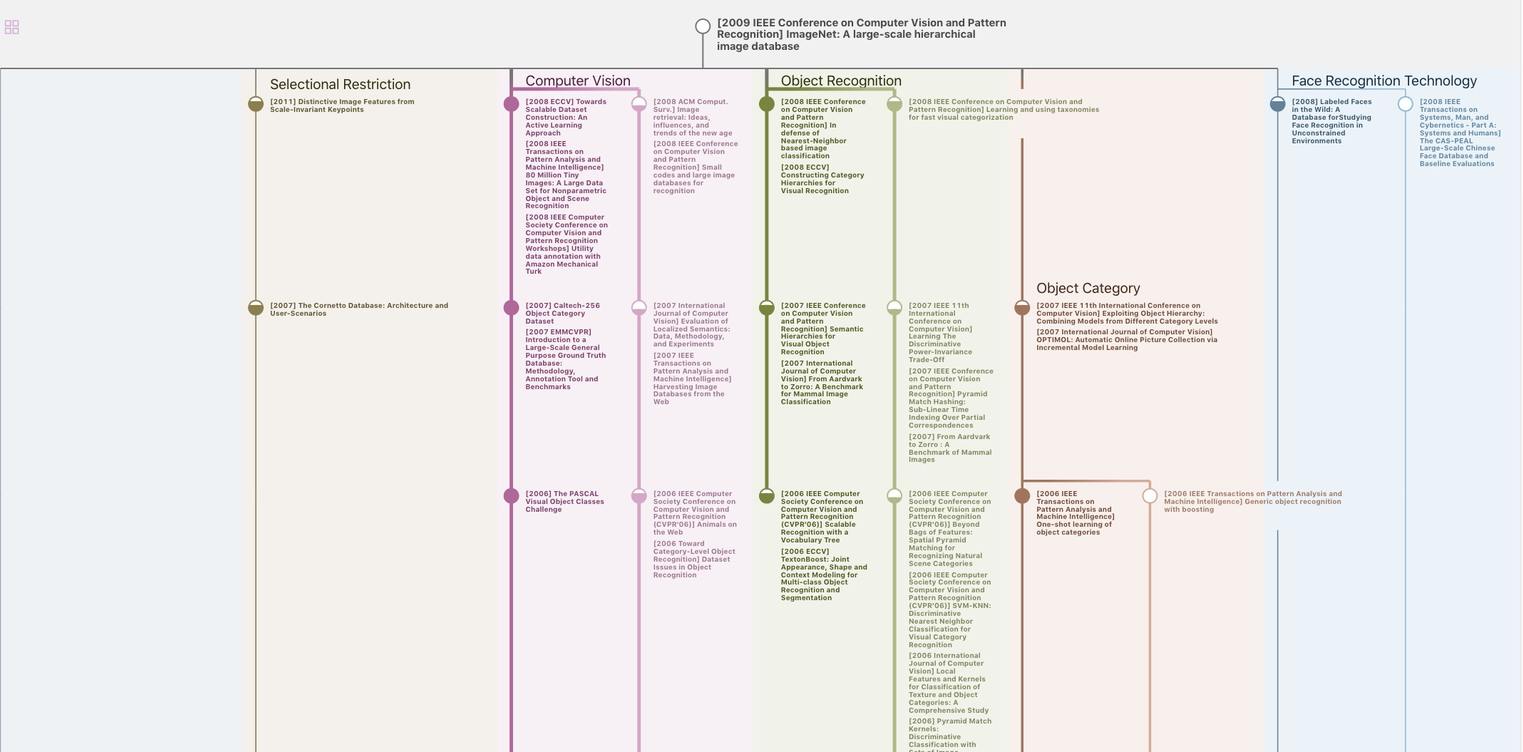
生成溯源树,研究论文发展脉络
Chat Paper
正在生成论文摘要