Machine Learning Based Tuner for Frequency-Selective PAPR Reduction
IEEE Transactions on Vehicular Technology(2023)
摘要
Frequency-selective peak-to-average power ratio (PAPR) reduction is essential in networks such as 5G New Radio (NR) that support frequency-domain multiplexing of users and services. However, stemming from the frequency-selective shaping of the involved clipping noise, the relation between the intended PAPR target and the actually realized PAPR is known to be heavily nonlinear, which complicates the PAPR reduction. In this article, a novel machine learning (ML)-based solution, called PAPRer, is proposed to automatically and accurately tune the optimal PAPR target for frequency-selective PAPR reduction. This is achieved by utilizing the features related to the used clipping noise filter and minimization of the defined loss function, through supervised learning, which quantifies the PAPR target estimation accuracy. An analytical clipping noise power-based method is also devised for reference purposes. Extensive numerical evaluations in 5G NR context are provided and analyzed, showing that PAPRer can very accurately predict and tune the optimal PAPR target. These results, together with the provided complexity assessment, demonstrate that the proposed PAPRer offers a favorable performance-complexity tradeoff in choosing the optimal PAPR target for frequency-selective PAPR reduction.
更多查看译文
关键词
Peak to average power ratio,OFDM,Indexes,Frequency-domain analysis,Frequency division multiplexing,Filtering,Time-domain analysis,5G NR,clipping and filtering,machine learning,PAPR,power-efficiency,supervised learning
AI 理解论文
溯源树
样例
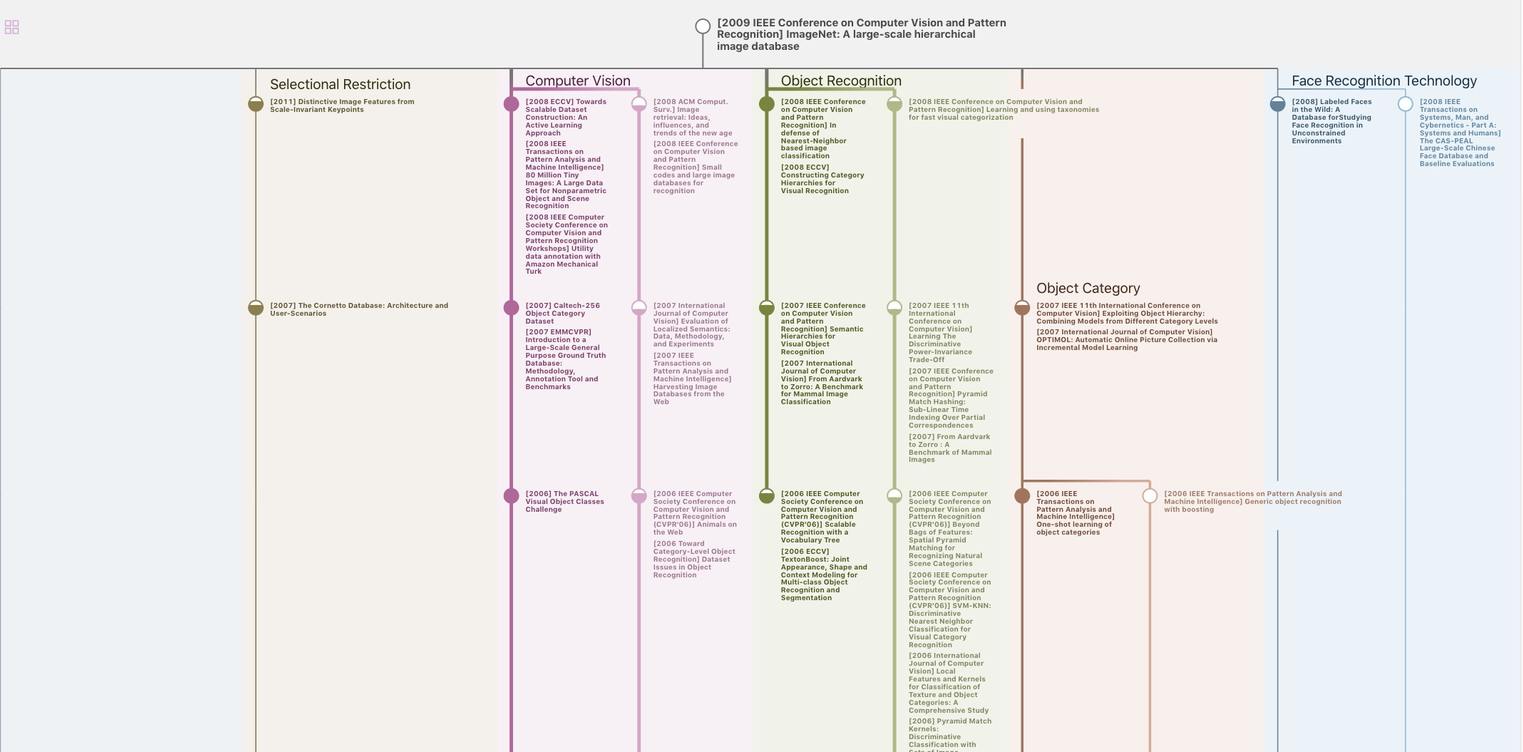
生成溯源树,研究论文发展脉络
Chat Paper
正在生成论文摘要