Learning Global Optimization by Deep Reinforcement Learning.
BRACIS (2)(2022)
摘要
Learning to Optimize (L2O) is a growing field that employs a variety of machine learning (ML) methods to learn optimization algorithms automatically from data instead of developing hand-engineered algorithms that usually require hyperparameter tuning and problem-specific design. However, there are some barriers to adopting those learned optimizers in practice. For instance, they exhibit instability during training, poor generalization to problems outside the distribution, and lack scalability. Current research efforts suggest either improving L2O models or improving training techniques to overcome such hardships. We focus on the latter and propose to train a Deep Reinforcement Learning (Deep RL) agent to learn an optimization algorithm from training in a diverse set of benchmark functions. To this end, we propose a general framework for learning to optimize by reinforcement learning, which adapts training strategies used in other L2O approaches, such as curriculum learning and input normalization. We investigate the importance of these strategies through an ablation study and show that even though Deep RL, to the best of our knowledge, is not a well-explored theme in L2O, it provides a direct framework to learn an optimizer able to deal with the exploration-exploitation dilemma and that the applied techniques improved stability and generalization.
更多查看译文
关键词
global optimization,learning
AI 理解论文
溯源树
样例
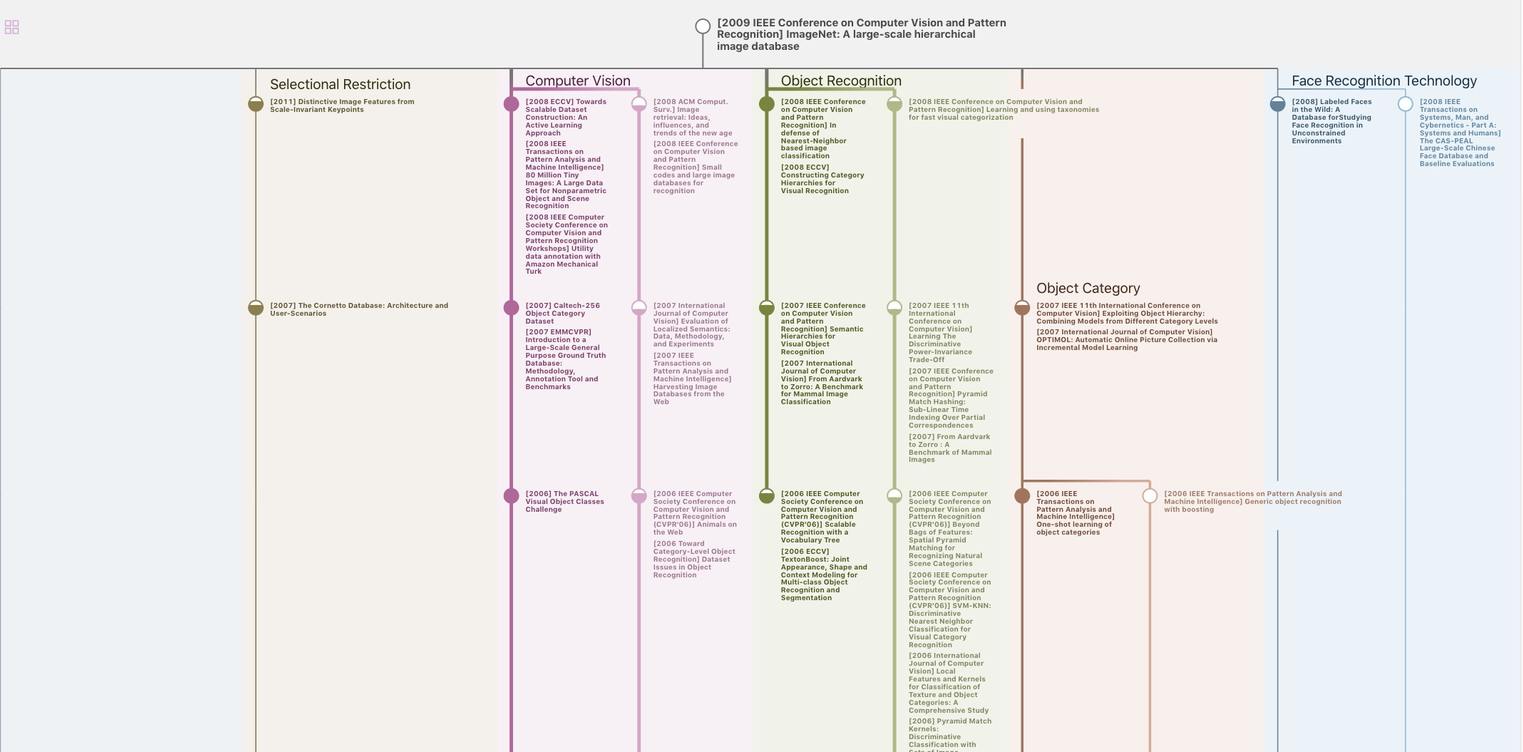
生成溯源树,研究论文发展脉络
Chat Paper
正在生成论文摘要