ATEAM: Knowledge Integration from Federated Datasets for Vehicle Feature Extraction using Annotation Team of Experts
arxiv(2022)
摘要
The vehicle recognition area, including vehicle make-model recognition (VMMR), re-id, tracking, and parts-detection, has made significant progress in recent years, driven by several large-scale datasets for each task. These datasets are often non-overlapping, with different label schemas for each task: VMMR focuses on make and model, while re-id focuses on vehicle ID. It is promising to combine these datasets to take advantage of knowledge across datasets as well as increased training data; however, dataset integration is challenging due to the domain gap problem. This paper proposes ATEAM, an annotation team-of-experts to perform cross-dataset labeling and integration of disjoint annotation schemas. ATEAM uses diverse experts, each trained on datasets that contain an annotation schema, to transfer knowledge to datasets without that annotation. Using ATEAM, we integrated several common vehicle recognition datasets into a Knowledge Integrated Dataset (KID). We evaluate ATEAM and KID for vehicle recognition problems and show that our integrated dataset can help off-the-shelf models achieve excellent accuracy on VMMR and vehicle re-id with no changes to model architectures. We achieve mAP of 0.83 on VeRi, and accuracy of 0.97 on CompCars. We have released both the dataset and the ATEAM framework for public use.
更多查看译文
关键词
federated datasets,vehicle feature
AI 理解论文
溯源树
样例
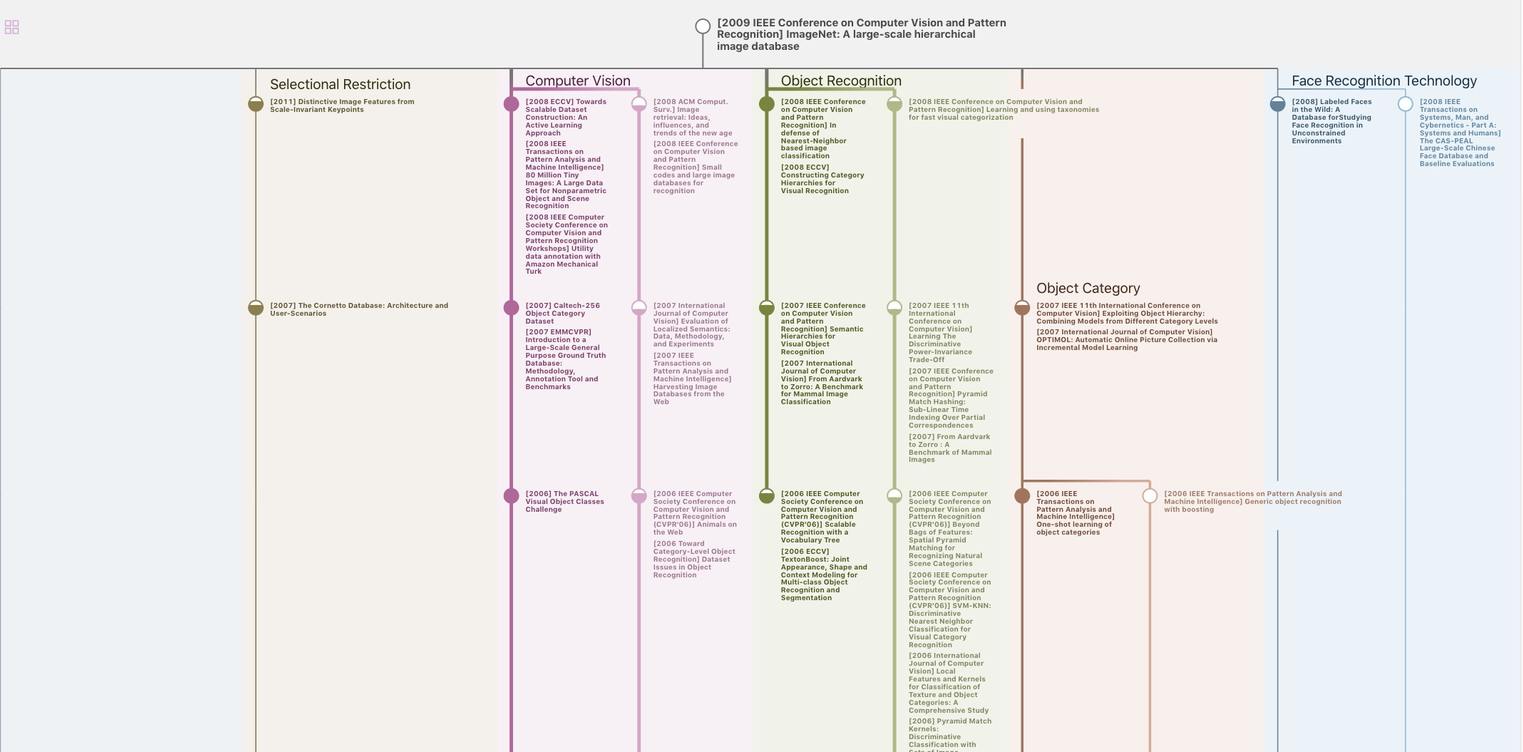
生成溯源树,研究论文发展脉络
Chat Paper
正在生成论文摘要