Automatic Spectral Representation With Improved Stacked Spectral Feature Space Patch (ISSFSP) for CNN-Based Hyperspectral Image Classification
IEEE TRANSACTIONS ON GEOSCIENCE AND REMOTE SENSING(2022)
摘要
Hyperspectral imaging (HSI) effectively supports land cover (LC) classification in understanding nature-human interactions. The LC classification quality depends heavily on the input features. Combining convolutional neural network (CNN) and stacked spectral feature space patch (SSFSP) has proved effective in simultaneously exploiting spatial and spectral features for accurate LC classification. SSFSP is a stack of gridded spectral feature space images (GSIs) where the spatial and intensity distributions of LCs are derived and directly learned by CNN. However, the GSI set was not fully optimized for HSI classification. In this article, we propose an improved SSFSP (ISSFSP) to tackle this problem, by integrating SSFSP with a novel feature selection (FS) method called band combination search (BCS). BCS first defines a novel metric to measure the ability of a GSI to distinguish various LCs, and then uses a coarse-to-fine strategy to efficiently identify the optimal GSI set. Furthermore, BCS provides a classifier-free method for determining the appropriate number of GSIs after trading off accuracy and efficiency. We conducted experiments using three HSI datasets, ISSFSP and five state-of-the-art FS methods, and six classification models to validate the effectiveness of ISSFSP. ISSFSP obtained consistently highest accuracy (over 98% overall accuracy, 97% average accuracy, and 97% Kappa) using fewer bands and samples, indicating that ISSFSP significantly boosts the performance of CNN while reducing the dependence on model structure and sample quality. In short, ISSFSP can automatically provide promising spectral features for CNN-based HSI classification regarding classification accuracy and model independency, showing great potential for practical applications.
更多查看译文
关键词
Feature extraction, Convolutional neural networks, Training, Measurement, Kernel, Data mining, Shape, Convolutional neural networks (CNNs), handcrafted feature, hyperspectral imaging (HSI) classification, improved stacked spectral feature space patch (ISSFSP), land cover (LC) classification
AI 理解论文
溯源树
样例
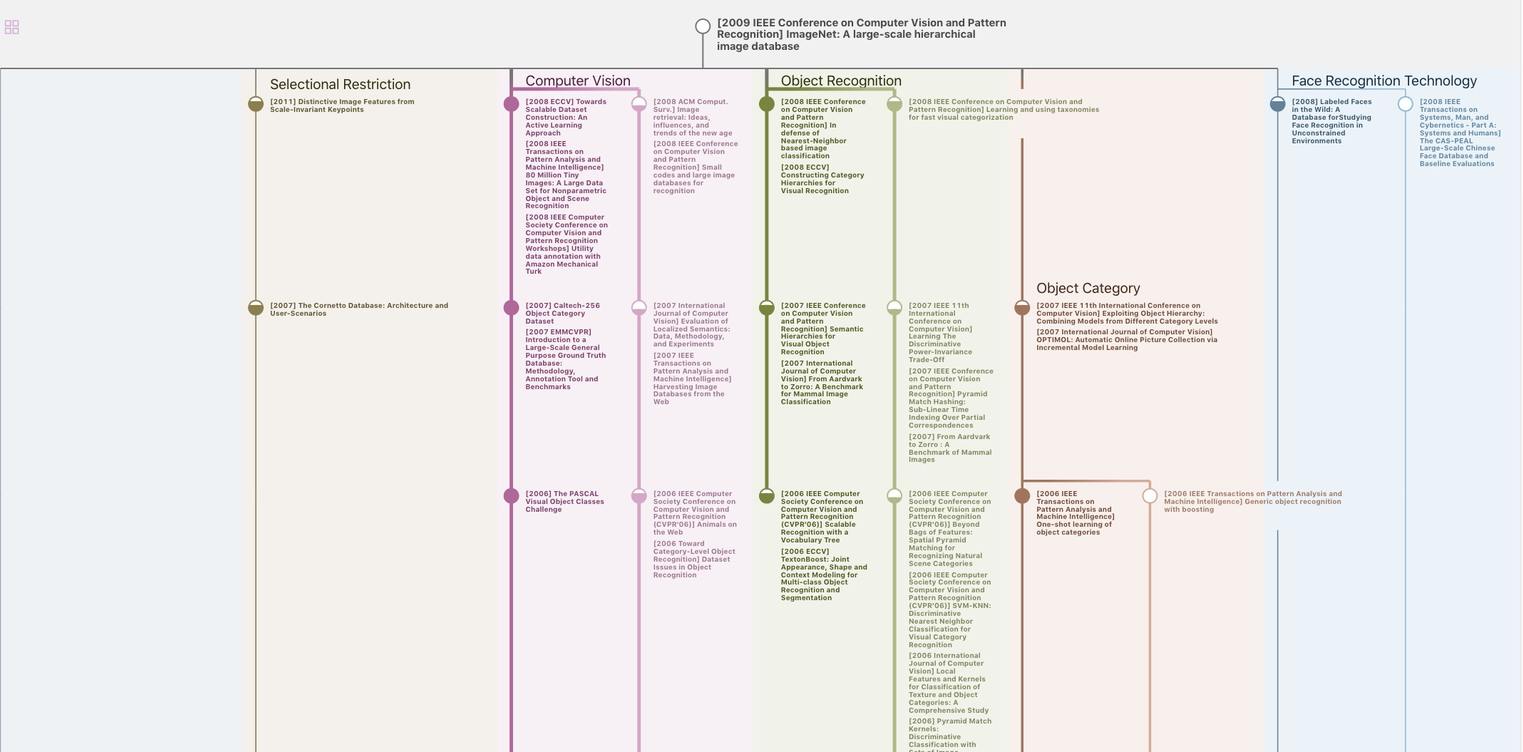
生成溯源树,研究论文发展脉络
Chat Paper
正在生成论文摘要