A Stratified Cascaded Approach for Brain Tumor Segmentation with the Aid of Multi-modal Synthetic Data
DATA AUGMENTATION, LABELLING, AND IMPERFECTIONS (DALI 2022)(2022)
摘要
Gliomas are one of the most widespread and aggressive forms of brain tumors. Accurate brain tumor segmentation is crucial for evaluation, monitoring and treatment of gliomas. Recent advances in deep learning methods have made a significant step towards a robust and automated brain tumor segmentation. However, due to the variation in shape and location of gliomas, as well as their appearance across different tumor grades, obtaining an accurate and generalizable segmentation model is still a challenge. To alleviate this, we propose a cascaded segmentation pipeline, aimed at introducing more robustness to segmentation performance through data stratification. In other words, we train separate models per tumor grade, aided with synthetic brain tumor images generated through conditional generative adversarial networks. To handle the variety in size, shape and location of tumors, we utilize a localization module, focusing the training and inference in the vicinity of the tumor. Finally, to identify which tumor grade segmentation model to utilize at inference time, we train a dense, attention-based 3D classification model. The obtained results suggest that both stratification and the addition of synthetic data to training significantly improve the segmentation performance, whereby up to 55% of test cases exhibit a performance improvement by more than 5% and up to 40% of test cases exhibit an improvement by more than 10% in Dice score.
更多查看译文
关键词
Brain tumor segmentation, Synthesis, Data stratification
AI 理解论文
溯源树
样例
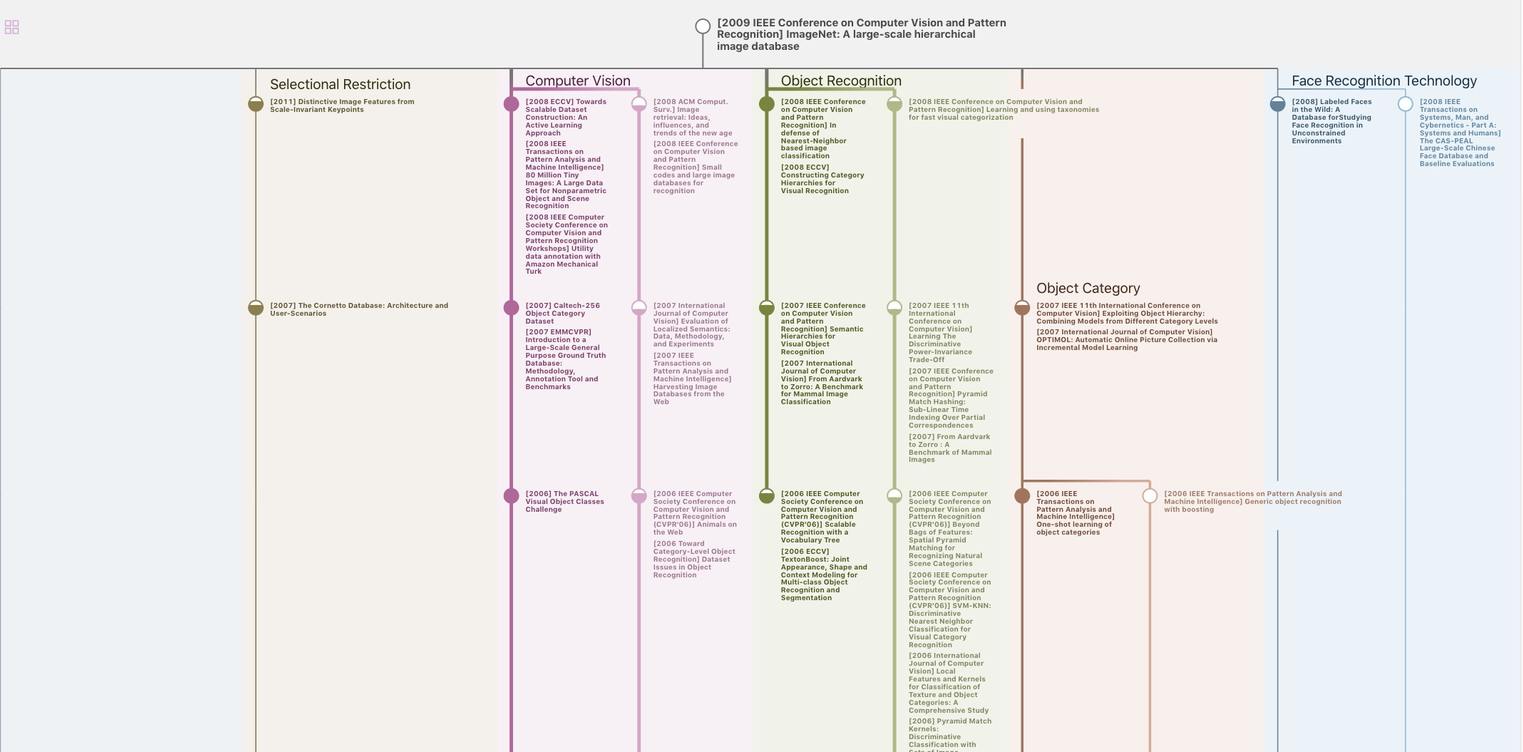
生成溯源树,研究论文发展脉络
Chat Paper
正在生成论文摘要