Experimental assessment of ground-truth faults in a typical single-duct dual-fan air-handling unit under Mediterranean climatic conditions: Impact scenarios of sensors? offset and fans? failure
ENERGY AND BUILDINGS(2022)
摘要
Data-driven Automated Fault Detection and Diagnosis (AFDD) is the automated process of detecting devi-ations (faults) from normal operation and diagnosing the type of problem and/or its location based on the exploitation of data collected under normal and faulty conditions. The performance of a typical single -duct dual-fan constant air volume air-handling unit (AHU) are investigated through a number of exper-iments performed during Italian cooling and heating seasons under both fault free and faulty scenarios. The AHU operation is analysed while artificially introducing six typical faults: 1) positive offset (+3 degrees C) of the return air temperature sensor; 2) negative offset (-3 degrees C) of the return air temperature sensor; 3) pos-itive offset (+15 %) of the return air relative humidity sensor; 4) negative offset (-15 %) of the return air relative humidity sensor; 5) complete failure of the return air fan; 6) complete failure of the supply air fan. The faulty tests are compared with the fault free experiments performed under the same boundary conditions to assess the impacts of the faults on both thermal/hygrometric indoor comfort and patterns of key operating parameters with the aim of supporting the studies focusing on new and accurate data -driven AFDD methods for AHUs. (c) 2022 Elsevier B.V. All rights reserved.
更多查看译文
关键词
Air -handling unit, Fan failure, Sensor offset, Experimental faulty data, Fault impact scenario, Fault detection and diagnosis, Energy management and efficiency
AI 理解论文
溯源树
样例
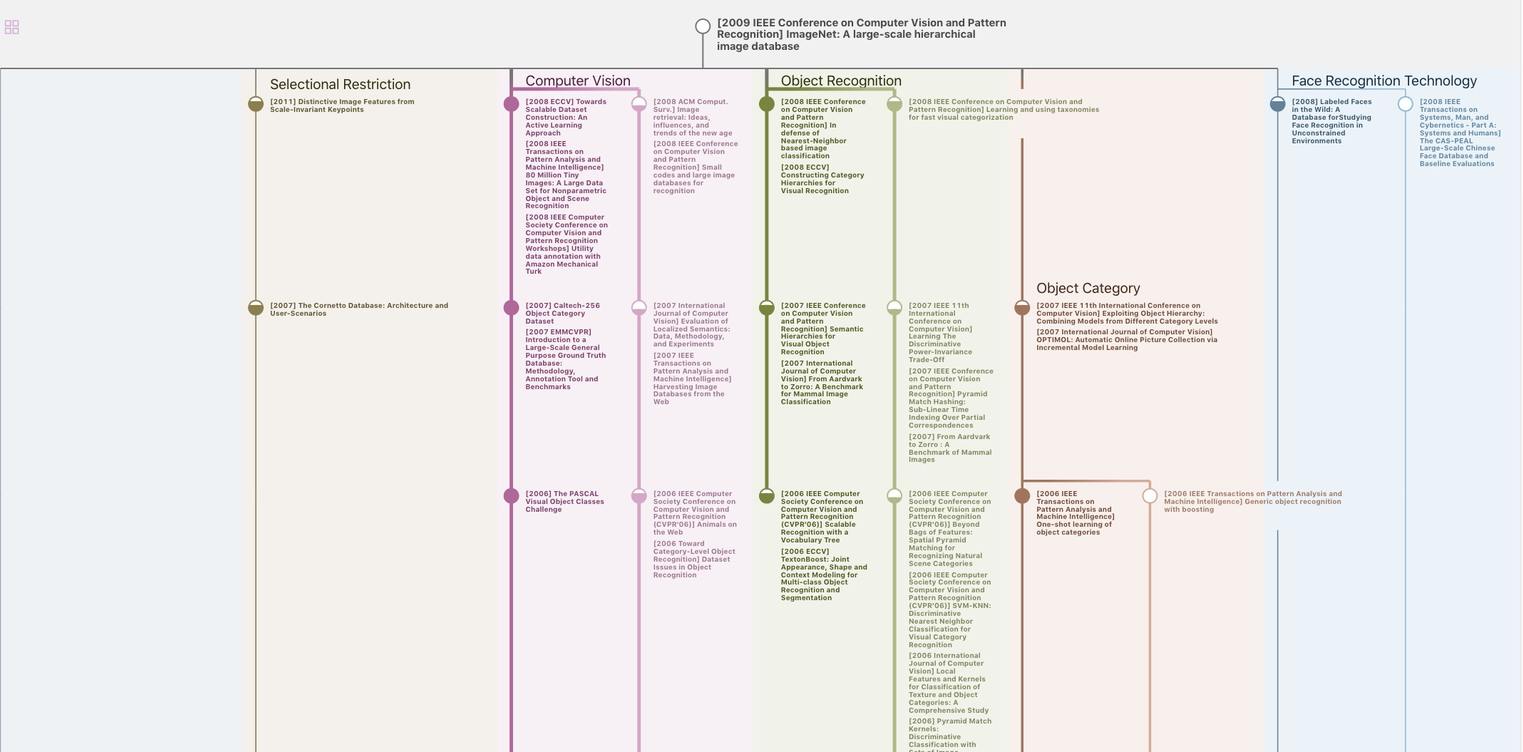
生成溯源树,研究论文发展脉络
Chat Paper
正在生成论文摘要