Learning-Based Diagnostics for Fault Detection and Isolation in Linear Stochastic Systems
2022 30TH MEDITERRANEAN CONFERENCE ON CONTROL AND AUTOMATION (MED)(2022)
摘要
AI-enabled mechanisms are deployed to guard controlled systems against sensor anomalies. We explore a two-level architecture design in which a low-level feedback controller of a linear system uses measurements from one or more potentially unreliable sensors. These observations are prone both to sensor noise but unknown additive faults. Our proposed, high-level, guard mechanism consists of a Reinforcement Learning (RL) agent that monitors available vitals of the system. In the event of a fault on the sensor components, the RL agent automatically detects, estimates the fault, localizes and takes action to cancel the fault. In addition, we develop design methodologies for efficient training of the RL agent that take advantage of system dynamics and sensor fusion schemes. We show that the associated training cost functions can be designed so that their optimal policy achieves efficient of arbitrary constant or piece-wise constant sensor faults. To illustrate our theoretical results, we consider a linearized version of a chemical process with multiple sensors, controlled by a Linear Quadratic Gaussian (LQG) Servo-Controller with Integral Action. Our simulations show that the RL-agent is successful in localizing the faulty sensors and mitigating the effects of faults in an online fashion.
更多查看译文
关键词
sensor anomalies,two-level architecture design,low-level feedback controller,linear system,potentially unreliable sensors,unknown additive faults,guard mechanism,monitors available vitals,sensor components,RL agent,design methodologies,system dynamics,sensor fusion schemes,associated training cost functions,arbitrary constant piece-wise,linearized version,multiple sensors,Linear Quadratic Gaussian Servo-Controller,RL-agent,faulty sensors,fault detection,Linear stochastic systems,AI-enabled mechanisms,controlled systems
AI 理解论文
溯源树
样例
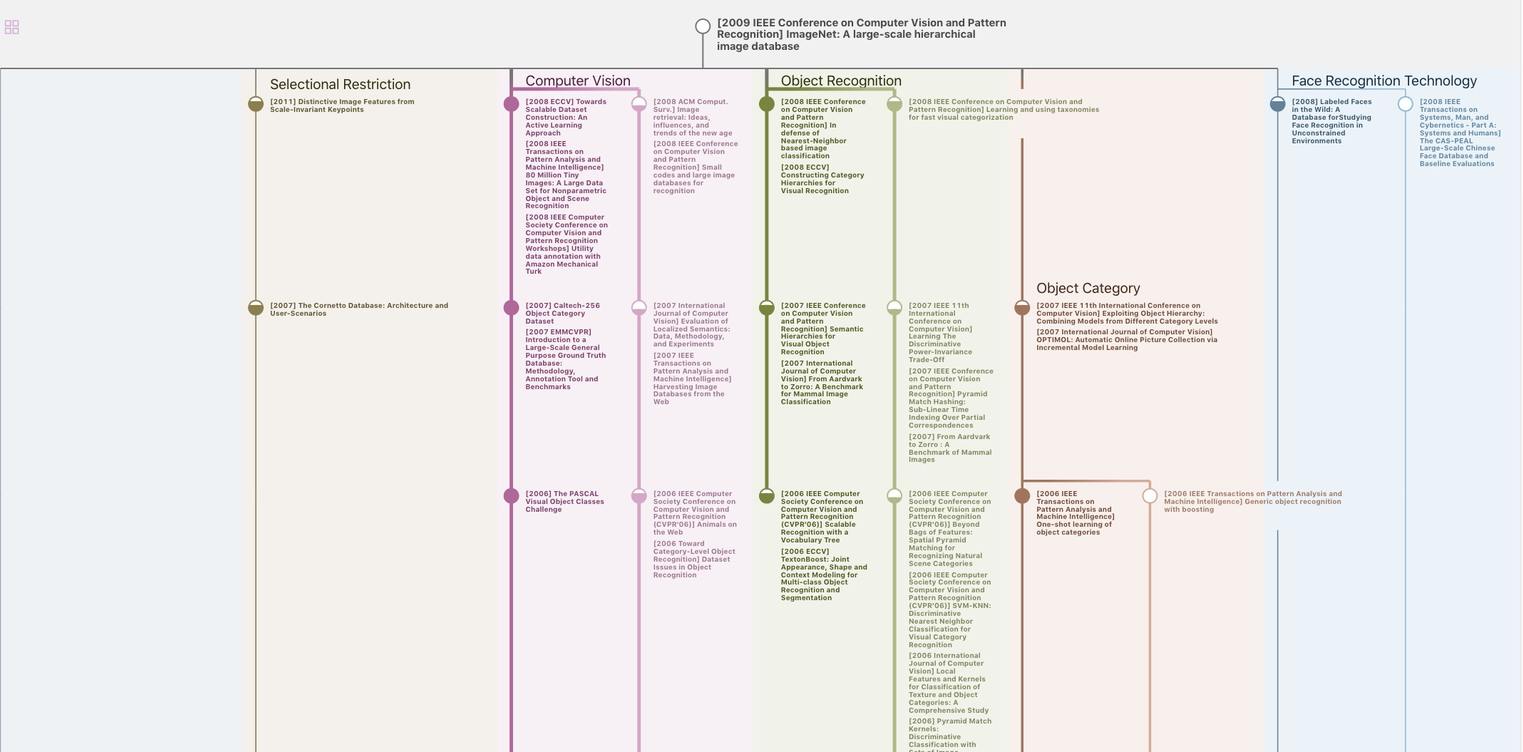
生成溯源树,研究论文发展脉络
Chat Paper
正在生成论文摘要