FDM-PINN: Physics-informed Neural Network Based on Fictitious Domain Method
International Journal of Computer Mathematics/International journal of computer mathematics(2022)
摘要
In this article, we present a physics-informed neural network combined with fictitious domain method (FDM-PINN) to study linear elliptic and parabolic problems with Robin boundary condition. Our goal here to develop a deep learning framework where one solves a variant of the original problem on the full Omega, followed by a well-chosen correction on small domain omega((omega) over bar subset of Omega), which is geometrically simple shaped domain. We study the applicability and accuracy of FDM-PINN for the elliptic and parabolic problems with fixed omega or moving omega. This method is of the virtual control type and relies on a well-designed neural network. Numerical results obtained by FDM-PINN for two-dimensional elliptic and parabolic problems are given, which are more accurate than the results obtained by least-squares/fictitious domain method in [R. Glowinski and Q. He, A least-squares/fictitious domain method for linear elliptic problems with robin boundary conditions, Commun. Comput. Phys. 9 (2011), pp. 587-606.].
更多查看译文
关键词
Fictitious domain method,neural network,Robin boundary condition,automatic differentiation,elliptic problem
AI 理解论文
溯源树
样例
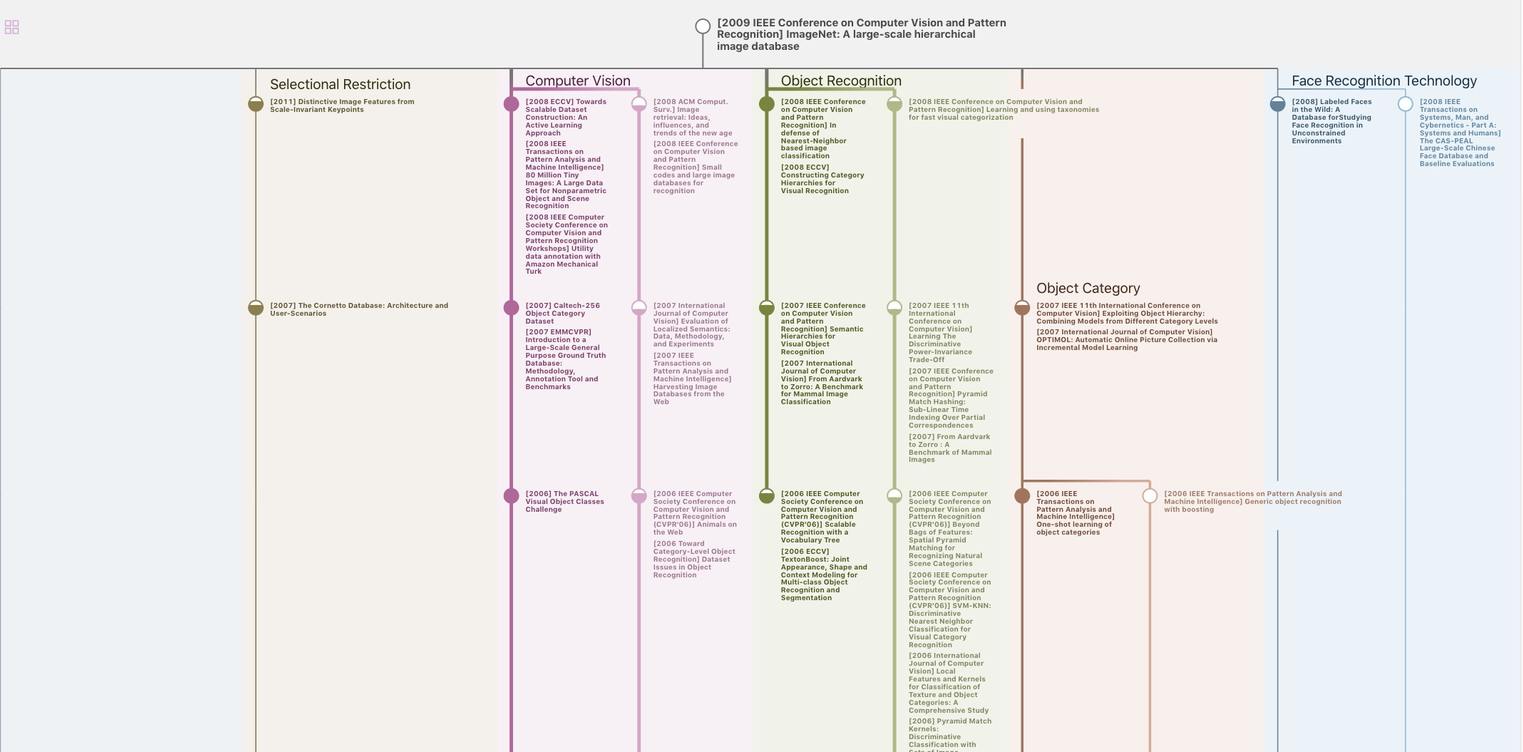
生成溯源树,研究论文发展脉络
Chat Paper
正在生成论文摘要