Distance-Based Random Forest Clustering with Missing Data
IMAGE ANALYSIS AND PROCESSING, ICIAP 2022, PT III(2022)
摘要
In recent years there has been an increased interest in clustering methods based on Random Forests, due to their flexibility and their capability in describing data. One problem of current RF-clustering approaches is that they are not able to directly deal with missing data, a common scenario in many application fields (e.g. Bioinformatics): the usual solution in this case is to pre-impute incomplete data before running standard clustering methods. In this paper we present the first Random Forest clustering approach able to directly deal with missing data. We start from the very recent RatioRF distance for clustering [3], which has shown to outperform all other distance-based RF clustering schemes, extending the framework in two directions, which allow the integration of missing data mechanisms directly inside the clustering pipeline. Experimental results, based on 6 standard UCI ML datasets, are promising, also in comparison with some literature alternatives.
更多查看译文
关键词
Random Forest clustering, Missing data, Ratio RF distance
AI 理解论文
溯源树
样例
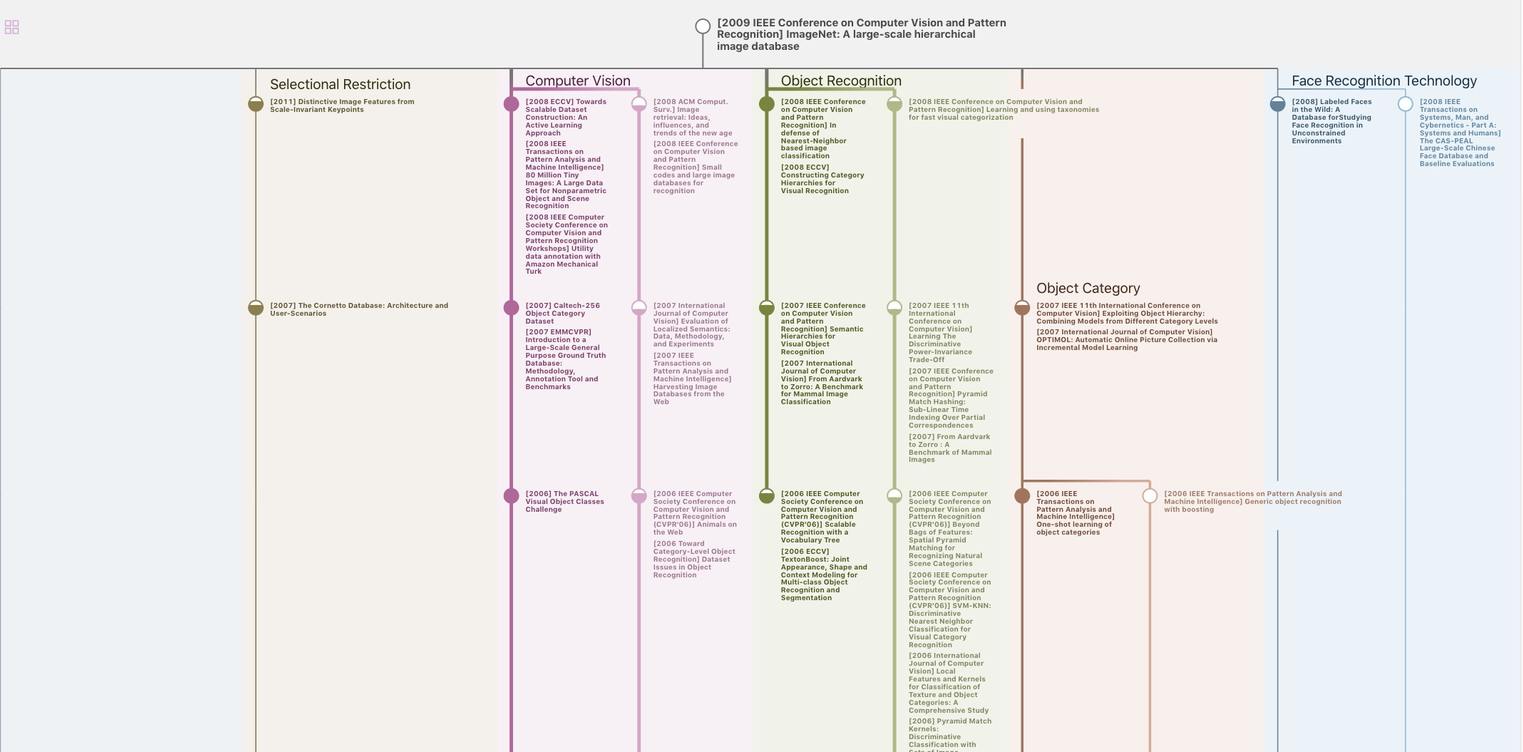
生成溯源树,研究论文发展脉络
Chat Paper
正在生成论文摘要