Using Random Forest Distances for Outlier Detection
IMAGE ANALYSIS AND PROCESSING, ICIAP 2022, PT III(2022)
摘要
In recent years, a great variety of outlier detectors have been proposed in the literature, many of which are based on pairwise distances or derived concepts. However, in such methods, most of the efforts have been devoted to the outlier detection mechanisms, not paying attention to the distance measure - in most cases the basic Euclidean distance is used. Instead, in the clustering field, data-dependent measures have shown to be very useful, especially those based on Random Forests: actually, Random Forests are partitioners of the space able to naturally encode the relation between two objects. In the outlier detection field, these informative distances have received scarce attention. This manuscript is aimed at filling this gap, studying the suitability of these measures in the identification of outliers. In our scheme, we build an unsupervised Random Forest model, from which we extract pairwise distances; these distances are then input to an outlier detector. In particular, we study the impact of several Random Forest-based distances, including advanced and recent ones, on different outlier detectors. We evaluate thoroughly our methodology on nine benchmark datasets for outlier detection, focusing on different aspects of the pipeline, such as the parametrization of the forest, the type of distance-based outlier detector, and most importantly, the impact of the adopted distance.
更多查看译文
关键词
Outlier detection, Random forest distances, Data-dependent distances
AI 理解论文
溯源树
样例
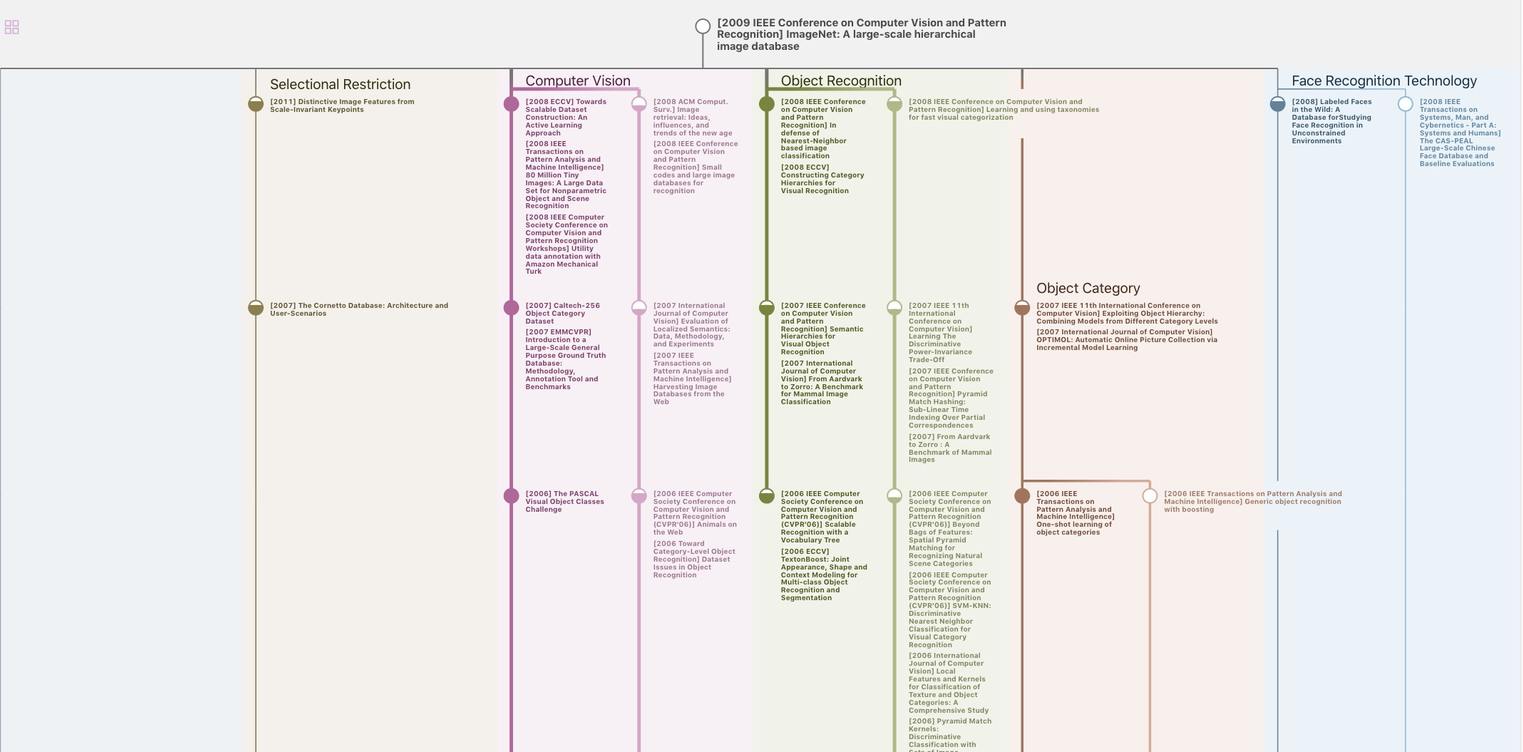
生成溯源树,研究论文发展脉络
Chat Paper
正在生成论文摘要