Adapting to Environment Changes Through Neuromodulation of Reinforcement Learning
FROM ANIMALS TO ANIMATS 16(2022)
摘要
Reinforcement learning (RL) enables agents to learn actions that can give maximum reward in an interactive environment. The environment is normally described as a predefined Markov Decision Process (MDP) which is assumed to remain unchanged throughout the life of RL agents. However, RL faces challenges when the environment changes. First, the agent needs to be able to detect the environment change rapidly when it occurs. Second, the agent needs to retain the knowledge learned before the environmental change. When facing an environment that was interacted with before, the learned knowledge should be recalled and utilized. To overcome these two challenges, we developed a biologically-inspired neuromodulation system that enables RL agents to quickly detect and adapt to environment changes. Our neuromodulation system is inspired by the effects of the cholinergic (ACh) and noradrenergic (NE) neuromodulatory systems on tracking uncertainties in the environment. We conducted experiments in the Gridworld environment and on a simulated MuJoCo robot to demonstrate the efficacy of our approach.
更多查看译文
关键词
Neuromodulation, Reinforcement learning, Uncertainty
AI 理解论文
溯源树
样例
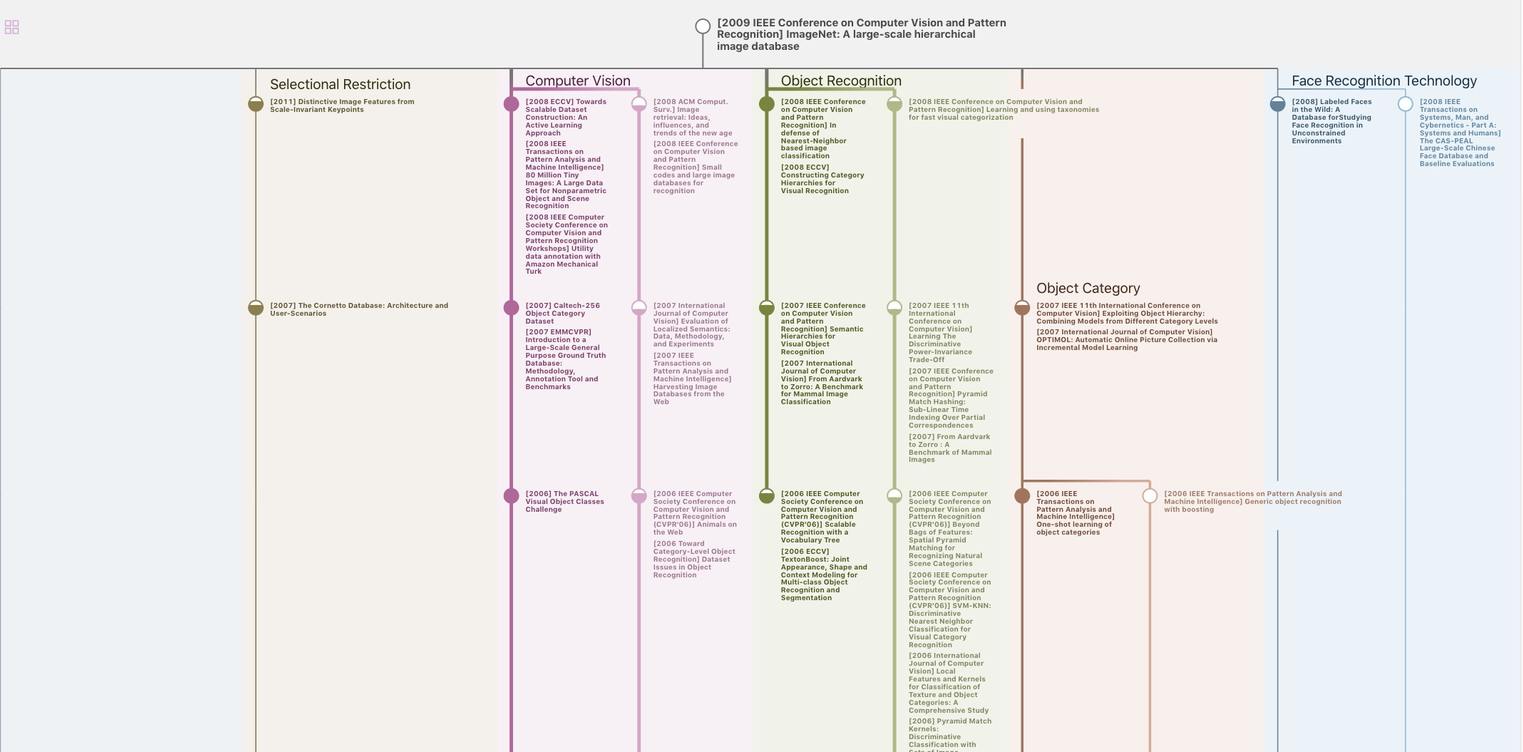
生成溯源树,研究论文发展脉络
Chat Paper
正在生成论文摘要