Transfer and Continual Supervised Learning for Robotic Grasping Through Grasping Features
CONTINUAL SEMI-SUPERVISED LEARNING, CSSL 2021(2022)
摘要
We present a Transfer and Continual Learning method for robotic grasping tasks, based on small vision-depth (RGBD) datasets and realized through the use of Grasping Features. Given a network architecture composed by a CNN (Convolutional Neural Network) followed by a FCC (Fully Connected Cascade Neural Network), we exploit high-level features specific of the grasping tasks, as extracted by the convolutional network from RGBD images. These features are more descriptive of a grasping task than just visual ones, and thus more efficient for transfer learning purposes. Being datasets for visual grasping less common than those for image recognition, we also propose an efficient way to generate these data using only simple geometric structures. This reduces the computational burden of the FCC and allows to obtain a better performance with the same amount of data. Simulation results using the collaborative UR-10 robot and a jaw gripper are reported to show the quality of the proposed method.
更多查看译文
关键词
Transfer Learning, Continual Learning, Robotic grasping
AI 理解论文
溯源树
样例
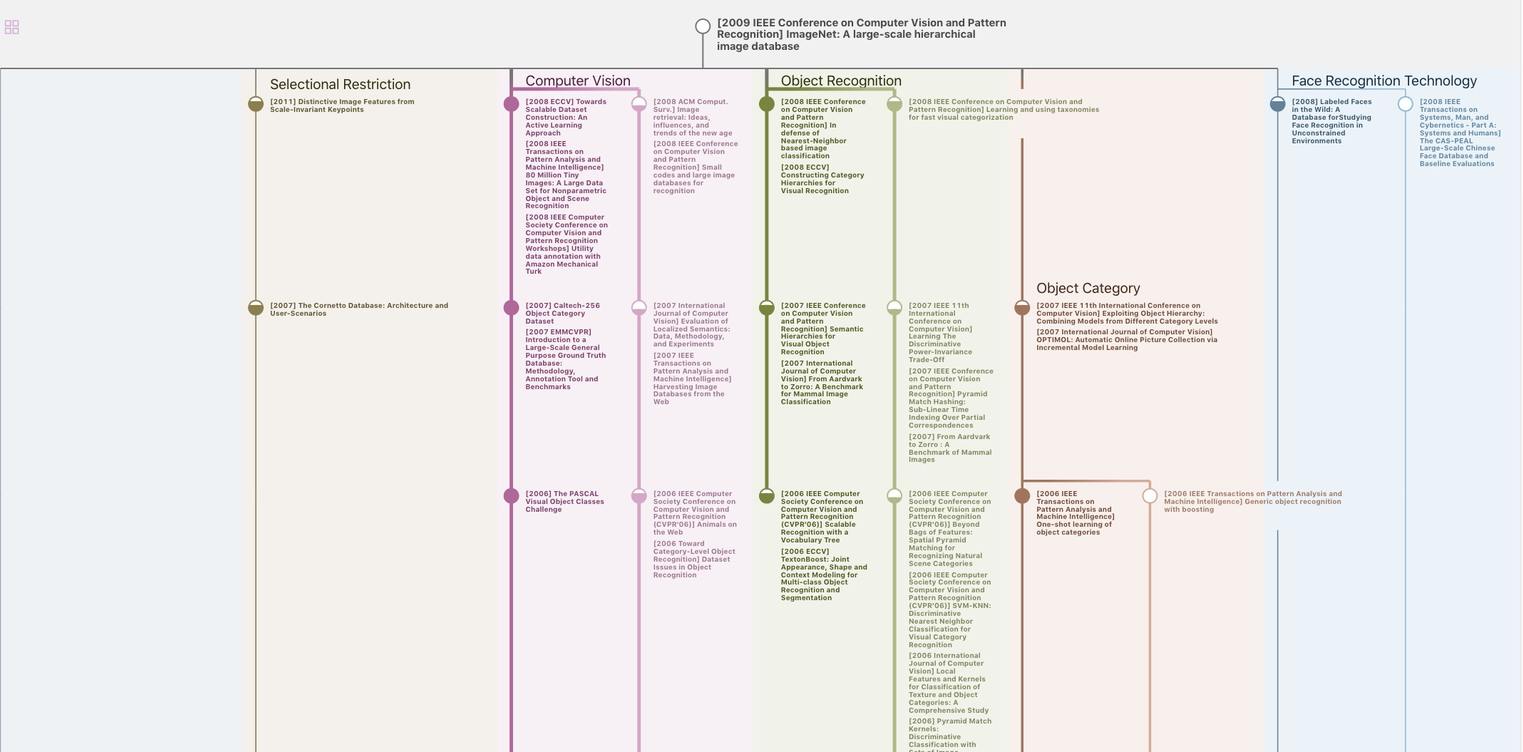
生成溯源树,研究论文发展脉络
Chat Paper
正在生成论文摘要