Query Expansion, Argument Mining and Document Scoring for an Efficient Question Answering System
EXPERIMENTAL IR MEETS MULTILINGUALITY, MULTIMODALITY, AND INTERACTION (CLEF 2022)(2022)
摘要
In the current world, individuals are faced with decision making problems and opinion formation processes on a daily basis. Nevertheless, answering a comparative question by retrieving documents based only on traditional measures (such as TF-IDF and BM25) does not always satisfy the need. In this paper, we propose a multi-layer architecture to answer comparative questions based on arguments. Our approach consists of a pipeline of query expansion, argument mining model, and sorting of the documents by a combination of different ranking criteria. Given the crucial role of the argument mining step, we examined two models: Disti1BERT and an ensemble learning approach using stacking of SVM and Disti1BERT. We compare the results of both models using two argumentation corpora on the level of argument identification task, and further using the dataset of CLEF 2021 Touche Lab shared task 2 on the level of answering comparative questions.
更多查看译文
关键词
Comparative Question Answering, Computational Argumentation, Argument Search
AI 理解论文
溯源树
样例
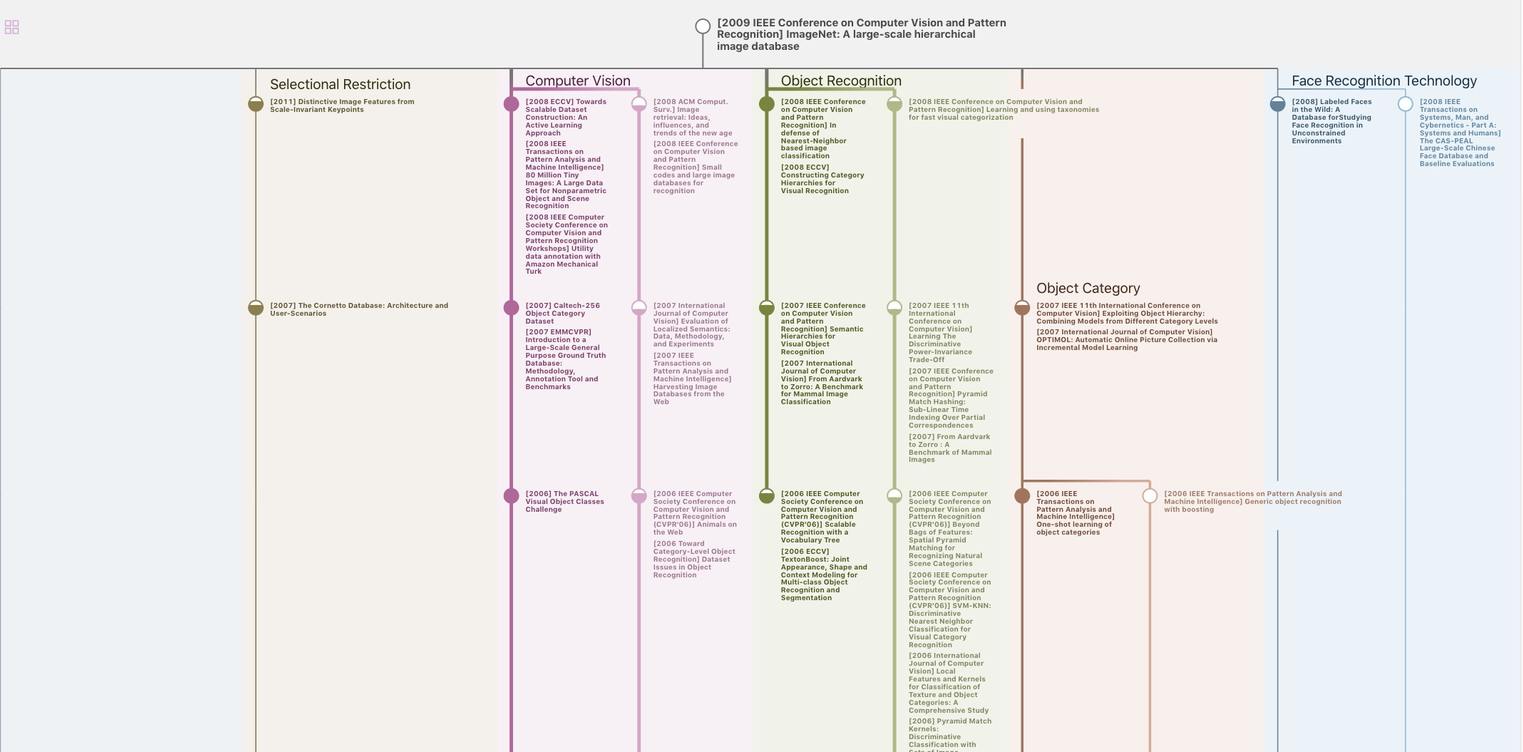
生成溯源树,研究论文发展脉络
Chat Paper
正在生成论文摘要