Low-Rank Tensor Tucker Decomposition for Hyperspectral Images Super-Resolution
INTELLIGENT ROBOTICS AND APPLICATIONS (ICIRA 2022), PT II(2022)
摘要
Super-resolution is an important way to improve the spatial resolution of Hyperspectral images (HSIs). In this paper, we propose a super-resolution method based on low-rank tensor Tucker Decomposition and weighted 3D total variation (TV) for HSIs. Global tensor Tucker decomposition and weighted 3D TV regularization are combined to exploit the prior knowledge of data low-rank information and local smoothness. Meanwhile, we use log-sum norm for tensor Tucker Decomposition to approximate the low-rank tensor. Extensive experiments show that our method outperforms some state-of-the-art methods on public HSI dataset.
更多查看译文
关键词
Low-rank tensor, Tucker decomposition, Hyperspectral images, Super-resolution
AI 理解论文
溯源树
样例
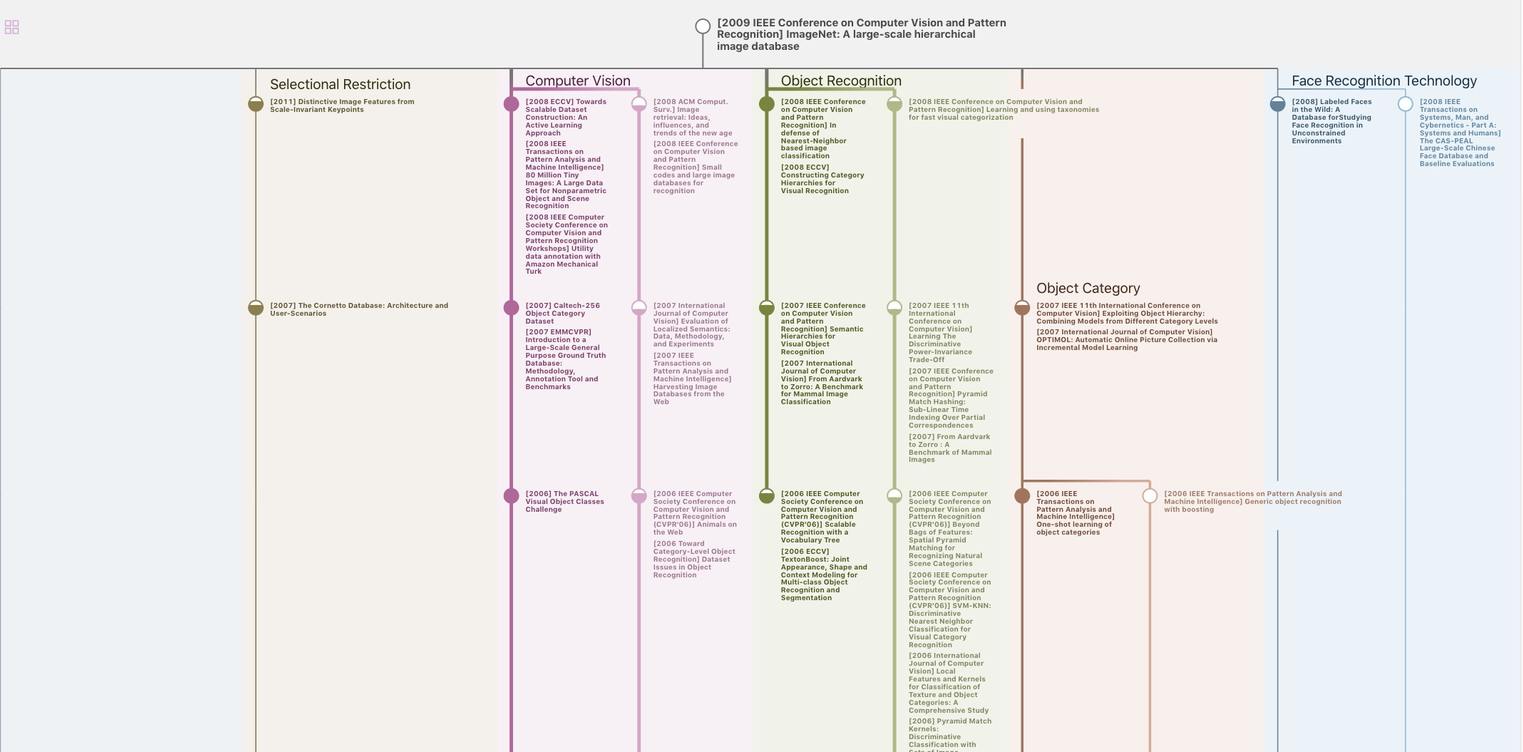
生成溯源树,研究论文发展脉络
Chat Paper
正在生成论文摘要