Exploring reinforcement learning approaches for drafting in collectible card games
Entertainment Computing(2023)
摘要
Collectible card games (CCGs), such as Magic: the Gathering and Hearthstone, are played by tens of millions worldwide and are known for their usually large and intricate rules. From an artificial intelligence (AI) standpoint, playing CCGs consists of two interdependent tasks: deck-building and battling. This paper presents three deep reinforcement learning approaches for deck-building in the arena mode of CCGs. Our approaches are trained in self-play and differ in the handling of past information when drafting new cards for a deck. We formulate the problem in a game-agnostic manner and perform experiments on Legends of Code and Magic, a CCG designed for AI research. Considering the win rate of the decks when used by fixed battling agents, the results show that our trained drafting agents outperform the best ones available for the game and do so by building very different decks. We also reenact the Strategy Card Game AI competition and show that our best drafting strategy improves the win rate of a baseline competitor by 14.9 and 12.7 percentage points in the 2019 and 2020 editions.
更多查看译文
关键词
Reinforcement learning,Collectible card game,Deck-building
AI 理解论文
溯源树
样例
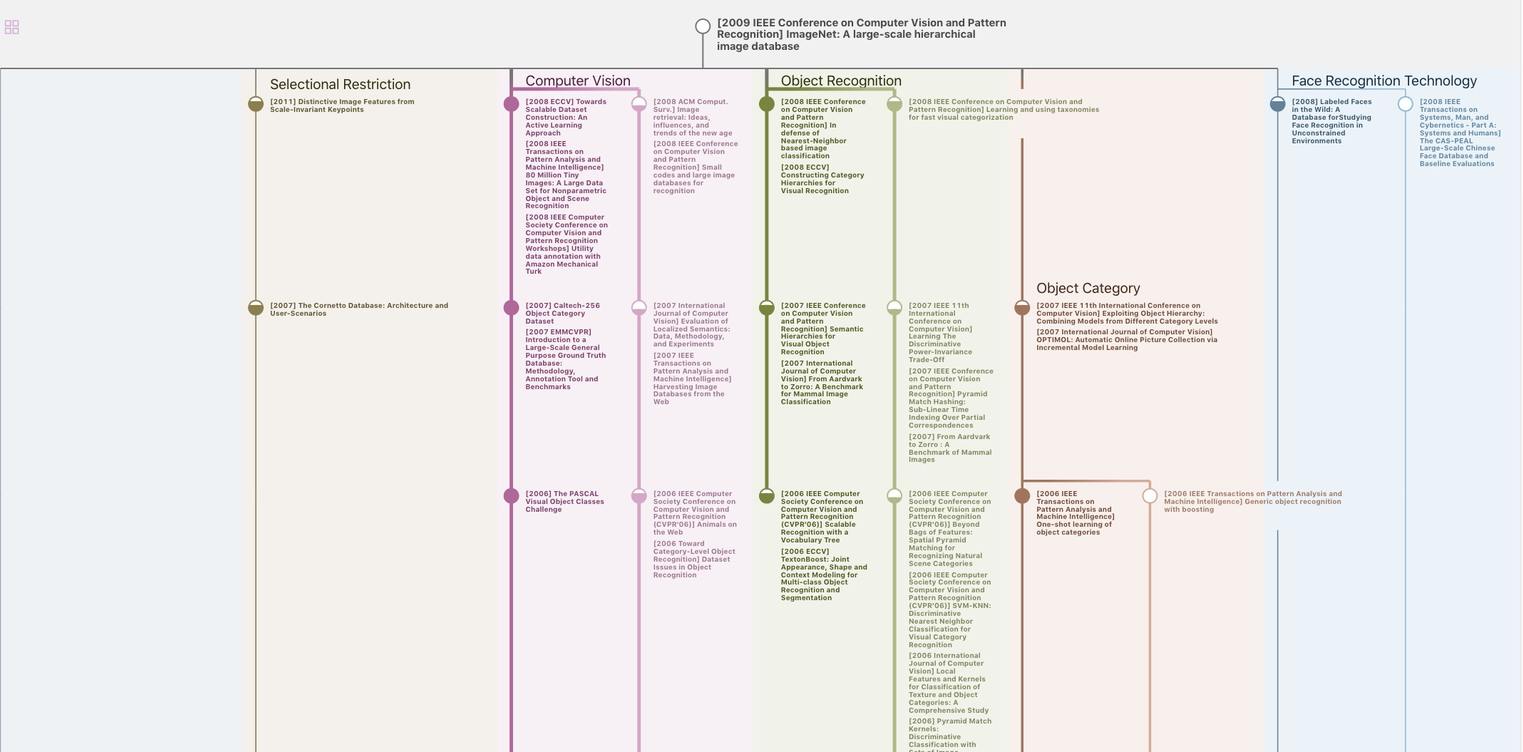
生成溯源树,研究论文发展脉络
Chat Paper
正在生成论文摘要