WACPN: A Neural Network for Pneumonia Diagnosis
COMPUTER SYSTEMS SCIENCE AND ENGINEERING(2023)
摘要
Community-acquired pneumonia (CAP) is considered a sort of pneu-monia developed outside hospitals and clinics. To diagnose community-acquired pneumonia (CAP) more efficiently, we proposed a novel neural network model. We introduce the 2-dimensional wavelet entropy (2d-WE) layer and an adaptive chaotic particle swarm optimization (ACP) algorithm to train the feed-forward neural network. The ACP uses adaptive inertia weight factor (AIWF) and Rossler attractor (RA) to improve the performance of standard particle swarm optimiza-tion. The final combined model is named WE-layer ACP-based network (WACPN), which attains a sensitivity of 91.87 +/- 1.37%, a specificity of 90.70 +/- 1.19%, a precision of 91.01 +/- 1.12%, an accuracy of 91.29 +/- 1.09%, F1 score of 91.43 +/- 1.09%, an MCC of 82.59 +/- 2.19%, and an FMI of 91.44 +/- 1.09%. The AUC of this WACPN model is 0.9577. We find that the max-imum deposition level chosen as four can obtain the best result. Experiments demonstrate the effectiveness of both AIWF and RA. Finally, this proposed WACPN is efficient in diagnosing CAP and superior to six state-of-the-art mod-els. Our model will be distributed to the cloud computing environment.
更多查看译文
关键词
Wavelet entropy, community-acquired pneumonia, neural network, adaptive inertia weight factor, rossler attractor, particle swarm optimization
AI 理解论文
溯源树
样例
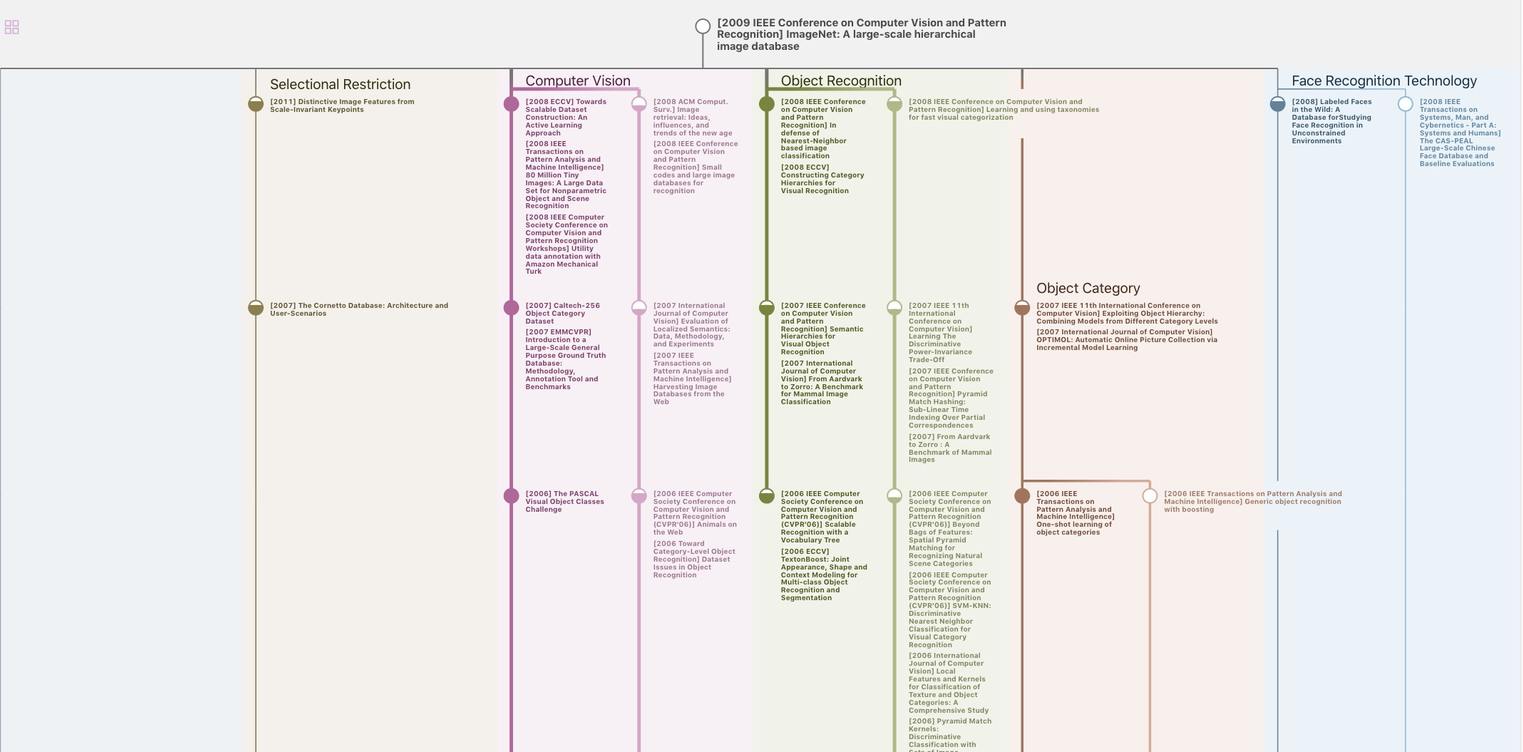
生成溯源树,研究论文发展脉络
Chat Paper
正在生成论文摘要