An online convex optimization-based framework for convex bilevel optimization
MATHEMATICAL PROGRAMMING(2022)
摘要
We propose a new framework for solving the convex bilevel optimization problem, where one optimizes a convex objective over the optimal solutions of another convex optimization problem. As a key step of our framework, we form an online convex optimization (OCO) problem in which the objective function remains fixed while the domain changes over time. We note that the structure of our OCO problem is different from the classical OCO problem that has been intensively studied in the literature. We first develop two OCO algorithms that work under different assumptions and provide their theoretical convergence rates. Our first algorithm works under minimal convexity assumptions, while our second algorithm is equipped to exploit structural information on the objective function, including smoothness, lack of first-order smoothness, and strong convexity. We then carefully translate our OCO results into their counterparts for solving the convex bilevel problem. In the context of convex bilevel optimization, our results lead to rates of convergence in terms of both inner and outer objective functions simultaneously, and in particular without assuming strong convexity in the outer objective function. Specifically, after T iterations, our first algorithm achieves O(T^-1/3) error bound in both levels, and this is further improved to O(T^-1/2) by our second algorithm. We illustrate the numerical efficacy of our algorithms on standard linear inverse problems and a large-scale text classification problem.
更多查看译文
关键词
optimization-based
AI 理解论文
溯源树
样例
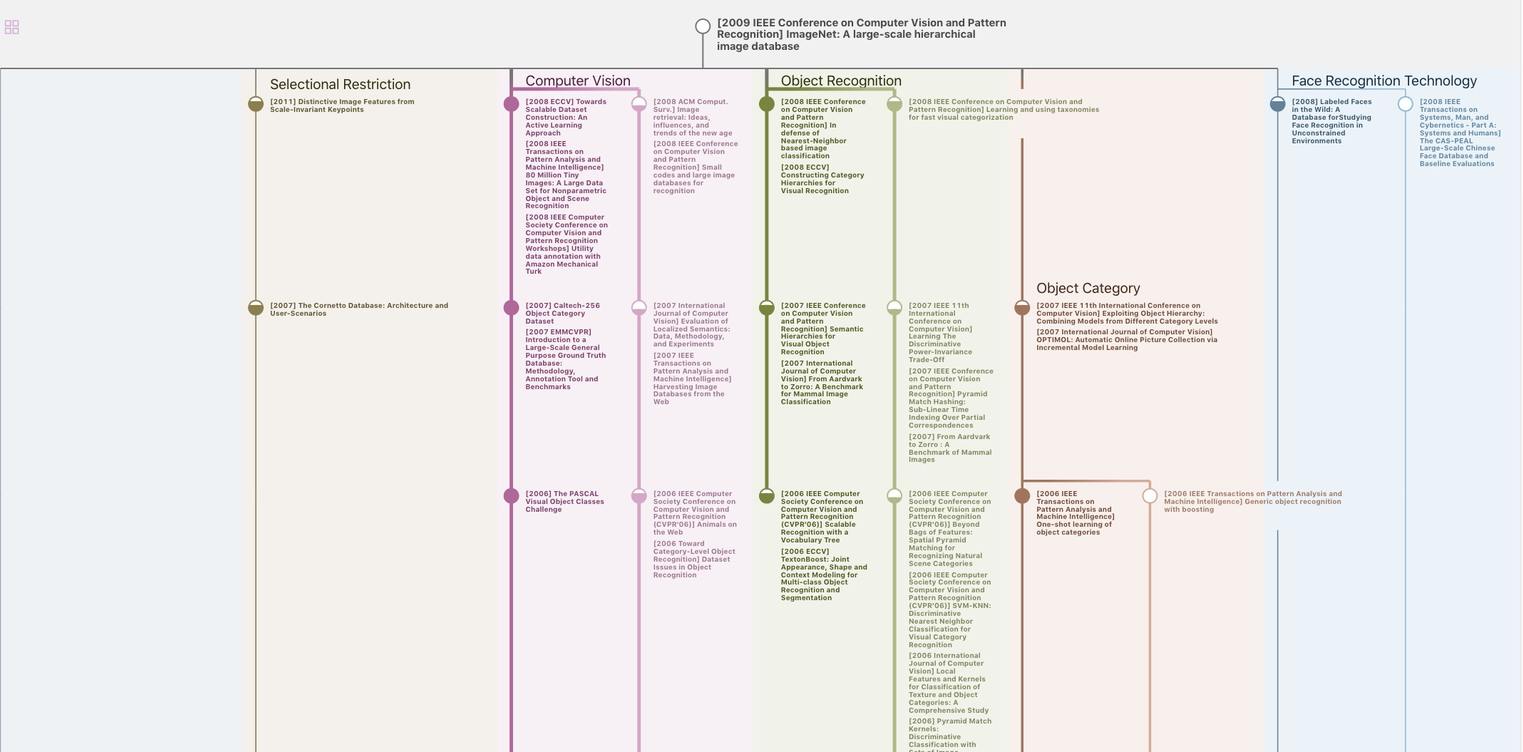
生成溯源树,研究论文发展脉络
Chat Paper
正在生成论文摘要