DuDoTrans: Dual-Domain Transformer for Sparse-View CT Reconstruction
MACHINE LEARNING FOR MEDICAL IMAGE RECONSTRUCTION (MLMIR 2022)(2022)
摘要
While Computed Tomography (CT) is necessary for clinical diagnosis, ionizing radiation in the imaging process induces irreversible injury, thereby driving researchers to study sparse-view CT reconstruction. Iterative models are proposed to alleviate the appeared artifacts in sparse-view CT images, but their computational cost is expensive. Deep-learning-based methods have gained prevalence due to the excellent reconstruction performances and computation efficiency. However, these methods ignore the mismatch between the CNN's local feature extraction capability and the sinogram's global characteristics. To overcome the problem, we propose Dual-Domain Transformer (DuDoTrans) to simultaneously restore informative sinograms via the long-range dependency modeling capability of Transformer and reconstruct CT image with both the enhanced and raw sinograms. With such a novel design, DuDoTrans even with fewer involved parameters is more effective and generalizes better than competing methods, which is confirmed by reconstruction performances on the NIH-AAPM and COVID-19 datasets. Finally, experiments also demonstrate its robustness to noise.
更多查看译文
关键词
CT Reconstruction, Sparse view, Sinogram restoration
AI 理解论文
溯源树
样例
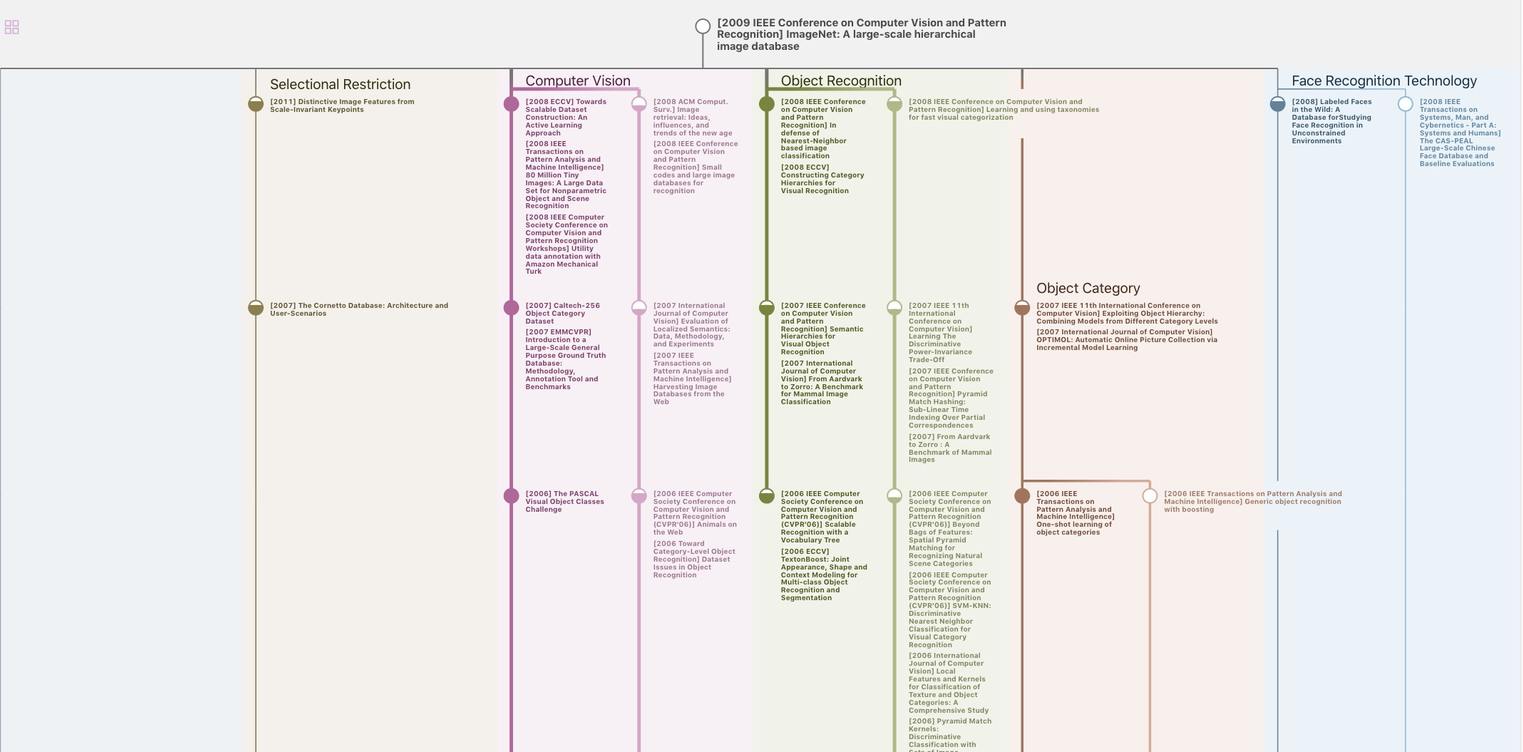
生成溯源树,研究论文发展脉络
Chat Paper
正在生成论文摘要