SNR-aware Automatic Modulation Recognition based on Modified Deep Residual Networks
2022 IEEE 95TH VEHICULAR TECHNOLOGY CONFERENCE (VTC2022-SPRING)(2022)
摘要
Recently, automatic modulation recognition (AMR), i.e., identifying the modulation modes of signals using deep learning (DL) has received much attention. This paper proposes an AMR method based on DL (i.e., SG-NET), including a novel DL architecture (i.e., GuResNet) based on the deep residual network (ResNet) and a SNR-aware mechanism, which can effectively extract signal characteristics to achieve a better recognition accuracy. Specifically, we design a deep residual network model that mainly consists of six novel Residual Units to abstract effective signal features and prevent over-fitting. Then, to further improve the recognition performance in low SNR scenarios (i.e., the SNR is lower than 0dB), we train different parameters in the GuResNets based on the aware SNR. That is, we firstly use original signals with high and low SNRs to train a general GusResNet. When SNR is larger than OdB, we directly adopt the general GuResNet for AMR. While, in low SNR scenarios, we exploit the Legendre method to extract signal features and then re-train the parameters in the GuResNets under different SNR conditions to improve the recognition accuracy further. The simulations demonstrate that our proposed SG-NET can obtain nearly 30% accuracy gain when the SNR is lower than OdB, and 10% improvement when SNR is larger than 0dB compared with existing schemes.
更多查看译文
关键词
Automatic modulation recognition (AMR), deep learning (DL), residual network (ResNet), SNR-aware mechanism
AI 理解论文
溯源树
样例
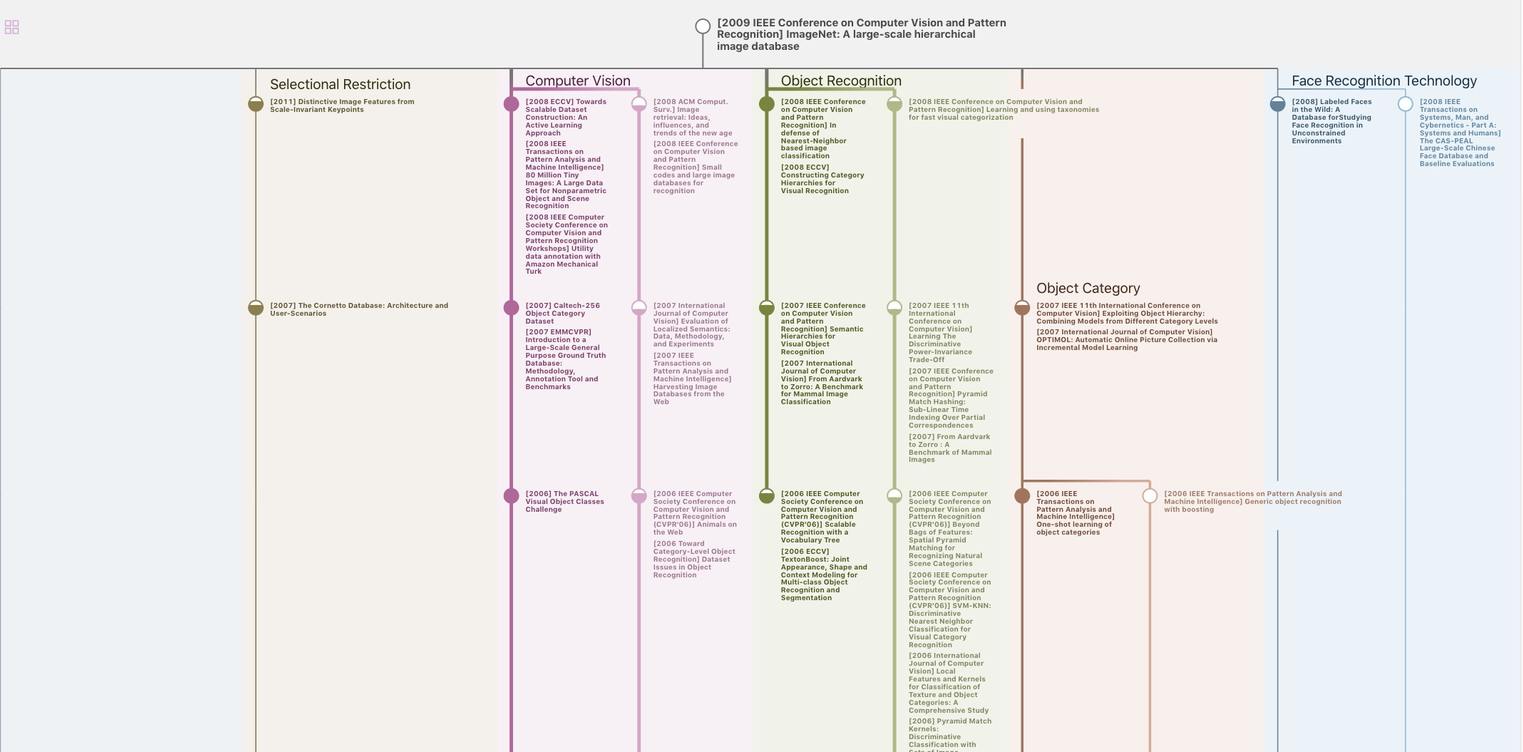
生成溯源树,研究论文发展脉络
Chat Paper
正在生成论文摘要