Markovian Segmentation of Non-stationary Data Corrupted by Non-stationary Noise
ADVANCES IN COMPUTING SYSTEMS AND APPLICATIONS(2022)
摘要
Hidden Markov models have been widely applied for data processing, mainly for image segmentation. However, when applied to non-stationary data, the results obtained may be poor due to mismatch between the models and reality. To this, such models have been extended to triplet Markov models which exhibit better performances while keeping similar computational complexity. In this paper, we introduce two novel models, based on triplet chain and field, able to handle simultaneously noise and classes non-stationarity, through a pairwise markovian auxiliary process. The performances of our models were assessed against regular hidden Markov models on simulated and synthetic images segmentation. The results obtained confirm the interest of the models proposed.
更多查看译文
关键词
Segmentation, Markov models, Non-stationarity
AI 理解论文
溯源树
样例
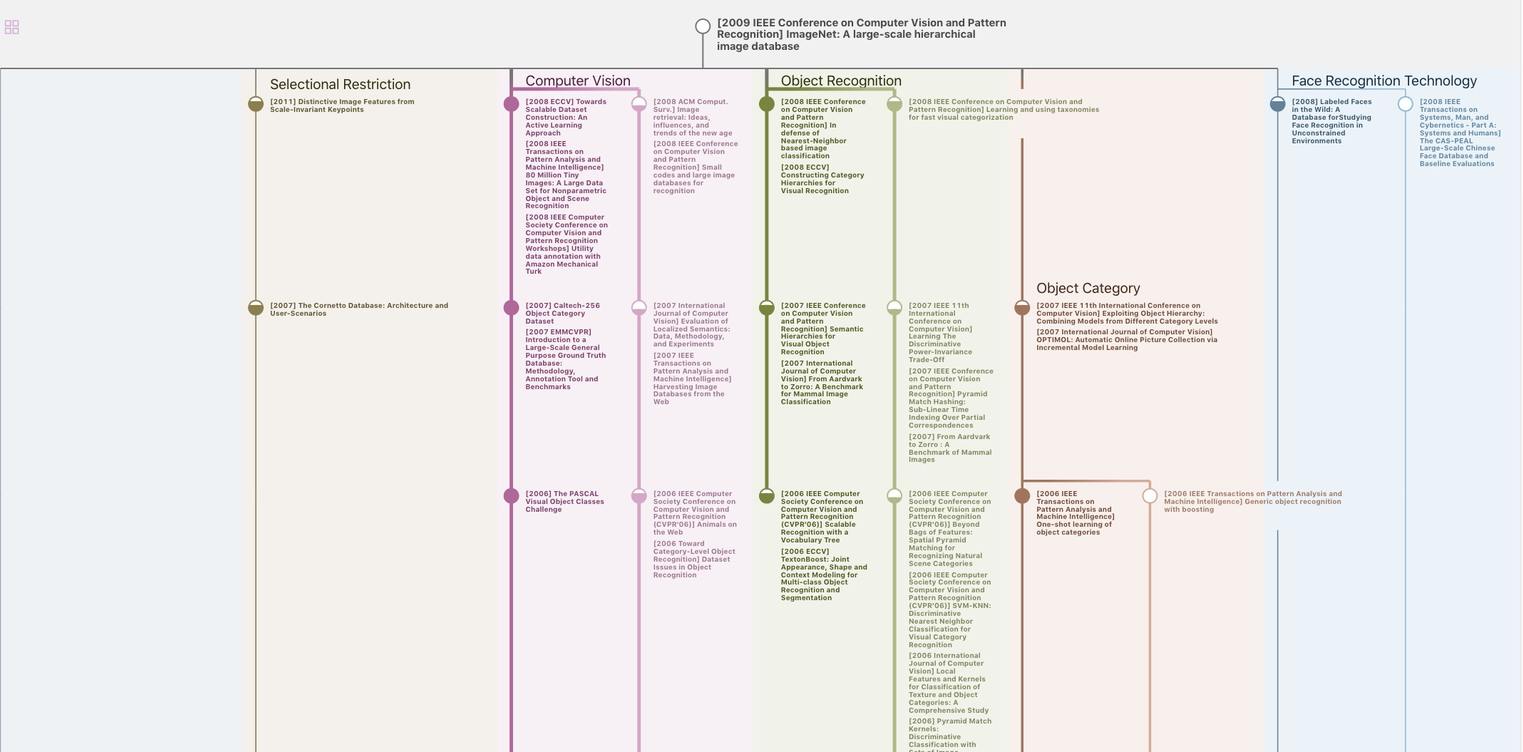
生成溯源树,研究论文发展脉络
Chat Paper
正在生成论文摘要