MUSCLE: Multi-task Self-supervised Continual Learning to Pre-train Deep Models for X-Ray Images of Multiple Body Parts
MEDICAL IMAGE COMPUTING AND COMPUTER ASSISTED INTERVENTION, MICCAI 2022, PT VIII(2022)
摘要
While self-supervised learning (SSL) algorithms have been widely used to pre-train deep models, few efforts [11] have been done to improve representation learning of X-ray image analysis with SSL pre-trained models. In this work, we study a novel self-supervised pre-training pipeline, namely Multi-task Self-super-vised Continual Learning (MUSCLE), for multiple medical imaging tasks, such as classification and segmentation, using X-ray images collected from multiple body parts, including heads, lungs, and bones. Specifically, MUSCLE aggregates X-rays collected from multiple body parts for MoCo-based representation learning, and adopts a well-designed continual learning (CL) procedure to further pre-train the backbone subject various X-ray analysis tasks jointly. Certain strategies for image pre-processing, learning schedules, and regularization have been used to solve data heterogeneity, overfitting, and catastrophic forgetting problems for multi-task/dataset learning in MUSCLE. We evaluate MUSCLE using 9 real-world X-ray datasets with various tasks, including pneumonia classification, skeletal abnormality classification, lung segmentation, and tuberculosis (TB) detection. Comparisons against other pre-trained models [7] confirm the proof-of-concept that self-supervised multi-task/dataset continual pre-training could boost the performance of X-ray image analysis.
更多查看译文
关键词
X-ray images (X-ray), Self-supervised learning
AI 理解论文
溯源树
样例
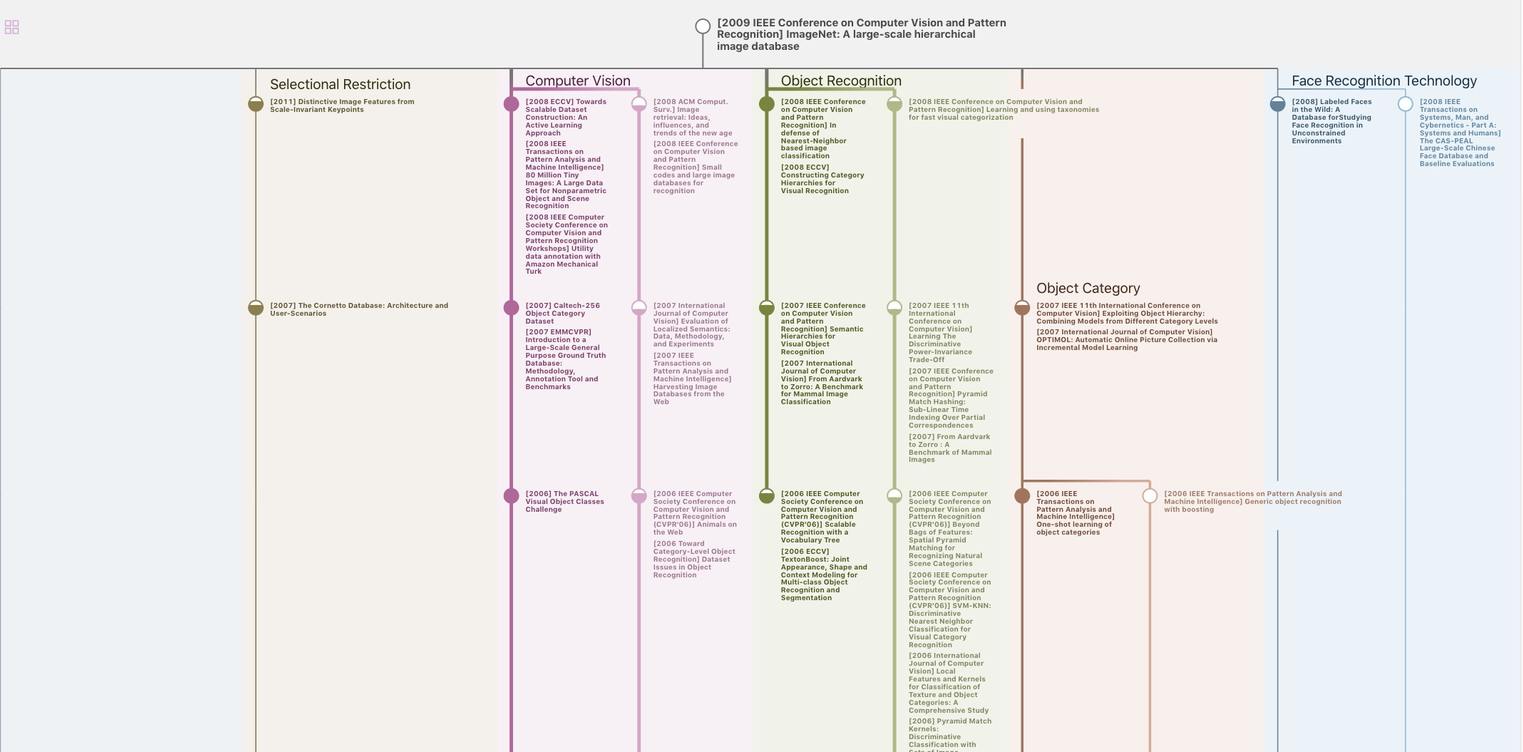
生成溯源树,研究论文发展脉络
Chat Paper
正在生成论文摘要