A novel hybrid method based on kernel-free support vector regression for stock indices and price forecasting
JOURNAL OF THE OPERATIONAL RESEARCH SOCIETY(2023)
摘要
Price forecasting in the financial market is one of the most important and challenging tasks in the field of time series forecasting since it is noisy, non-linear and non-stationary. In this paper, we first develop a kernel-free support vector regression model which not only has a strong flexibility to capture the nonlinear structure of the data but also maintains the high efficiency to avoid choosing a suitable kernel and its related parameters. Then a novel hybrid method is proposed combining empirical mode decomposition algorithm, quadratic surface support vector regression and autoregressive integrated moving average method for the stock indices and future price forecasting. This ensemble scheme fully takes the advantages of these individual methods to efficiently produce accurate time series forecasts. Finally, to compare our proposed method with other benchmark forecasting methods, three stock indices and three future prices are selected as the forecasting targets. The numerical results and statistical test strongly demonstrate the promising performance of our proposed hybrid method in terms of forecasting accuracy, efficiency and robustness.
更多查看译文
关键词
Autoregressive integrated moving average, empirical mode decomposition, financial time series forecasting, kernel-free support vector regression, quadratic surface
AI 理解论文
溯源树
样例
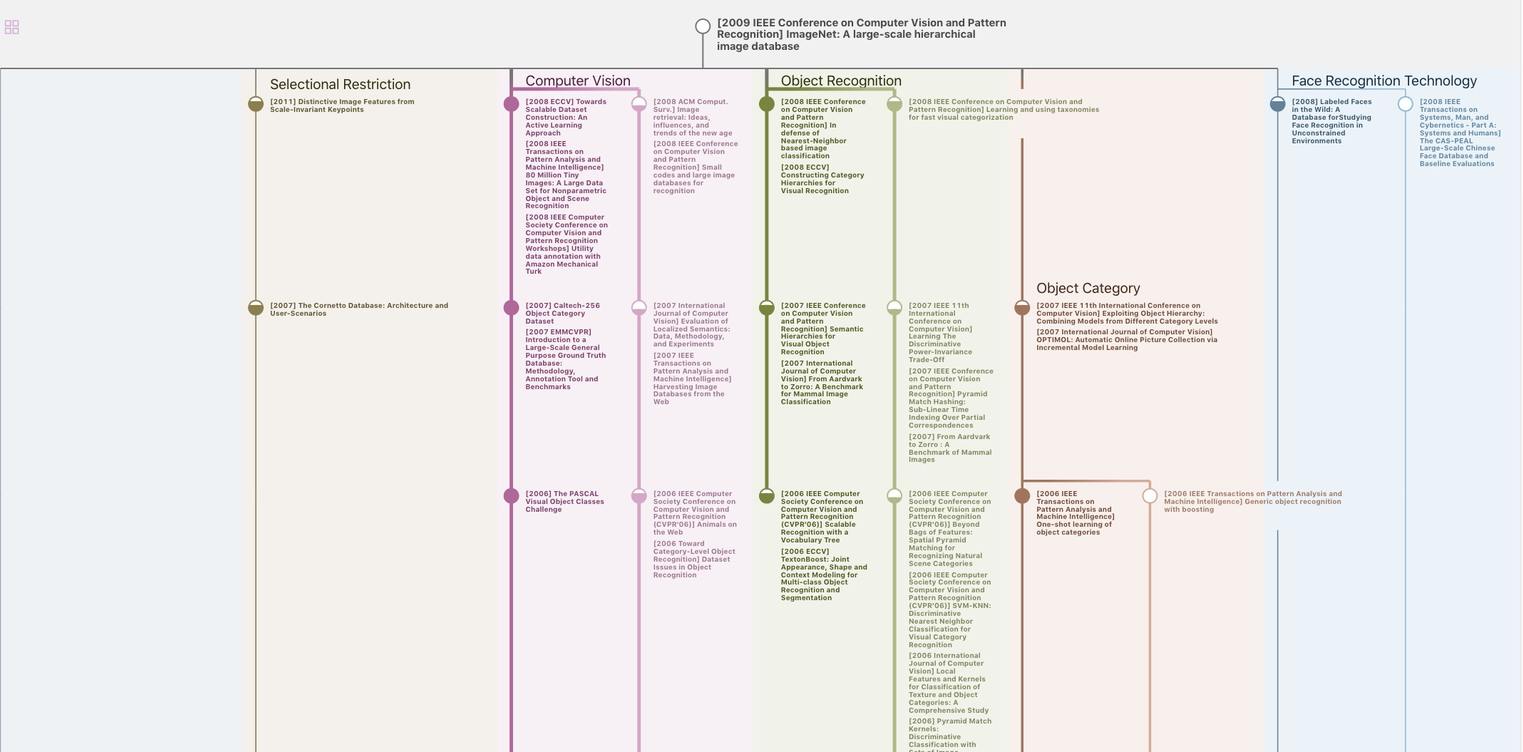
生成溯源树,研究论文发展脉络
Chat Paper
正在生成论文摘要