Efficient data acquisition and training of collisional-radiative model artificial neural network surrogates through adaptive parameter space sampling
MACHINE LEARNING-SCIENCE AND TECHNOLOGY(2022)
摘要
Effective plasma transport modeling of magnetically confined fusion devices relies on having an accurate understanding of the ion composition and radiative power losses of the plasma. Generally, these quantities can be obtained from solutions of a collisional-radiative (CR) model at each time step within a plasma transport simulation. However, even compact, approximate CR models can be computationally onerous to evaluate, and in-situ evaluation of these models within a larger plasma transport code can lead to a rigid bottleneck. As a way to bypass this bottleneck, we propose deploying artificial neural network (ANN) surrogates to allow rapid evaluation of the necessary plasma quantities. However, one issue with training an accurate ANN surrogate is the reliance on a sufficiently large and representative training and validation data set, which can be time-consuming to generate. In this work we explore a data-driven active learning and training routine to allow autonomous adaptive sampling of the problem parameter space to ensure a sufficiently large and meaningful set of training data is assembled for the network training. As a result, we can demonstrate approximately order-of-magnitude savings in required training data samples to produce an accurate surrogate.
更多查看译文
关键词
collisional-radiative, plasma model, artificial neural network, adaptive sampling, active learning, surrogate model, exploration versus exploitation
AI 理解论文
溯源树
样例
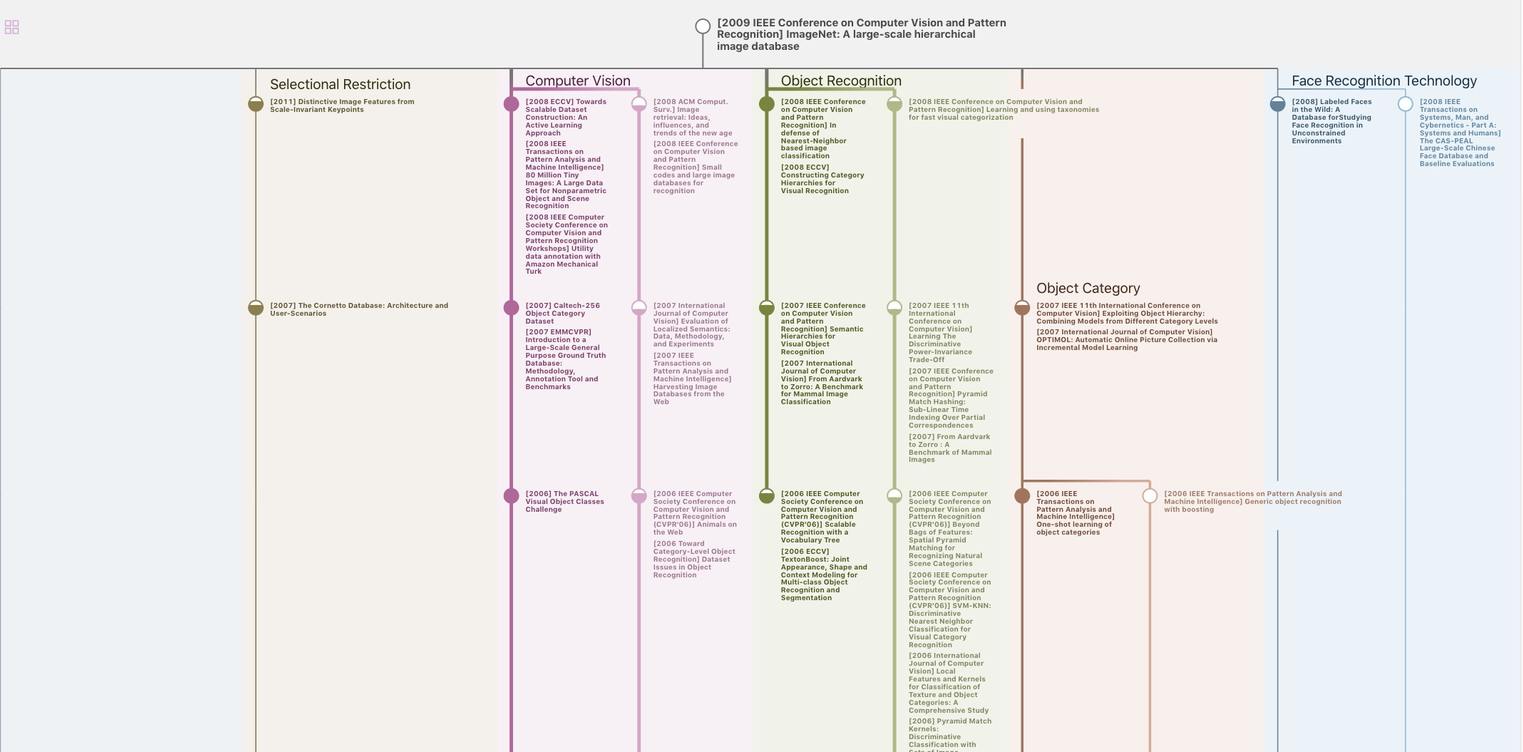
生成溯源树,研究论文发展脉络
Chat Paper
正在生成论文摘要