Neural Regression For Scale-Varying Targets
arxiv(2023)
摘要
In this work, we demonstrate that a major limitation of regression using a mean-squared error loss is its sensitivity to the scale of its targets. This makes learning settings consisting of target's whose values take on varying scales challenging. A recently-proposed alternative loss function, known as histogram loss, avoids this issue. However, its computational cost grows linearly with the number of buckets in the histogram, which renders prediction with real-valued targets intractable. To address this issue, we propose a novel approach to training deep learning models on real-valued regression targets, autoregressive regression, which learns a high-fidelity distribution by utilizing an autoregressive target decomposition. We demonstrate that this training objective allows us to solve regression tasks involving targets with different scales.
更多查看译文
关键词
neural regression,targets,scale-varying
AI 理解论文
溯源树
样例
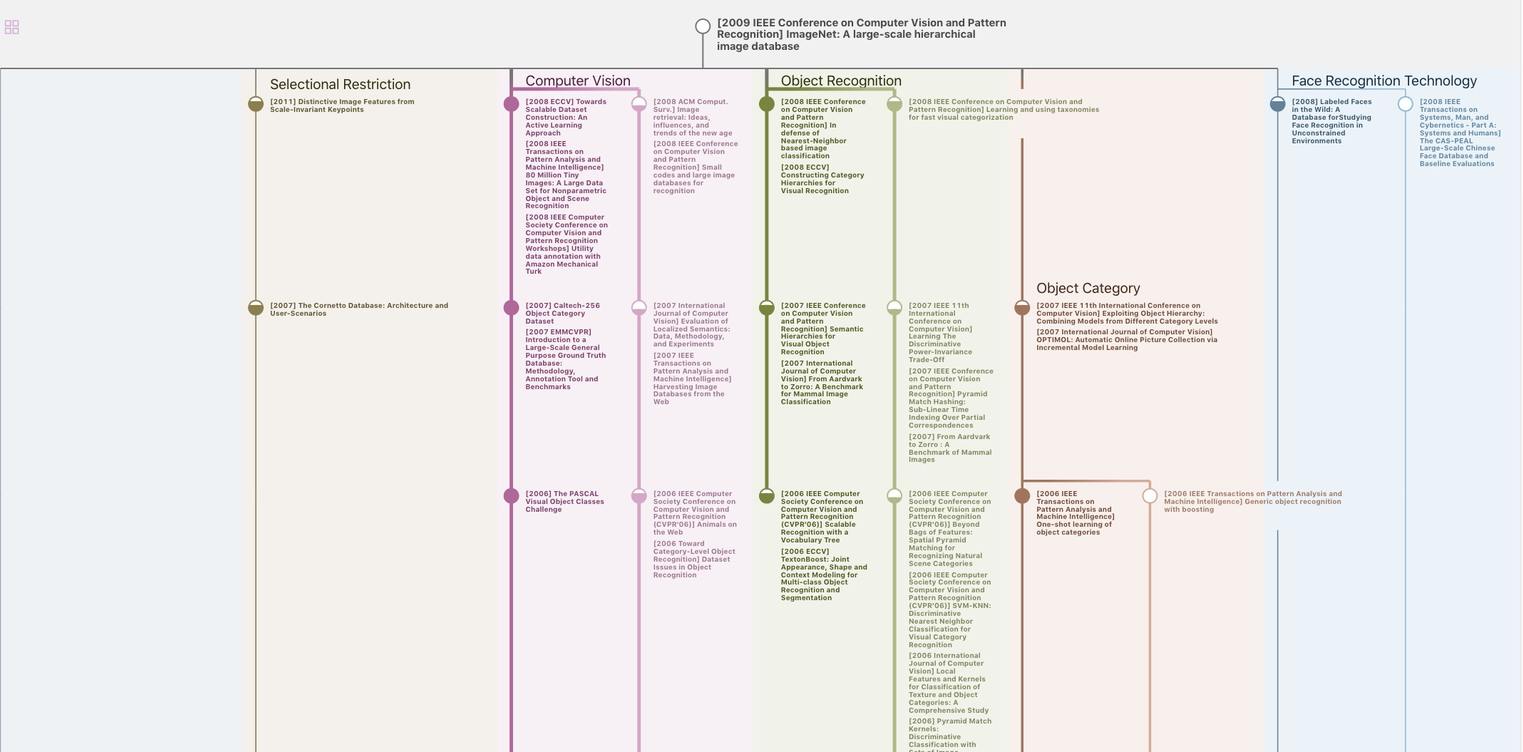
生成溯源树,研究论文发展脉络
Chat Paper
正在生成论文摘要