Multi-center anatomical segmentation with heterogeneous labels via landmark-based models
arxiv(2022)
摘要
Learning anatomical segmentation from heterogeneous labels in multi-center datasets is a common situation encountered in clinical scenarios, where certain anatomical structures are only annotated in images coming from particular medical centers, but not in the full database. Here we first show how state-of-the-art pixel-level segmentation models fail in naively learning this task due to domain memorization issues and conflicting labels. We then propose to adopt HybridGNet, a landmark-based segmentation model which learns the available anatomical structures using graph-based representations. By analyzing the latent space learned by both models, we show that HybridGNet naturally learns more domain-invariant feature representations, and provide empirical evidence in the context of chest X-ray multiclass segmentation. We hope these insights will shed light on the training of deep learning models with heterogeneous labels from public and multi-center datasets.
更多查看译文
关键词
anatomical segmentation, landmark-based models, missing annotations, graph neural networks
AI 理解论文
溯源树
样例
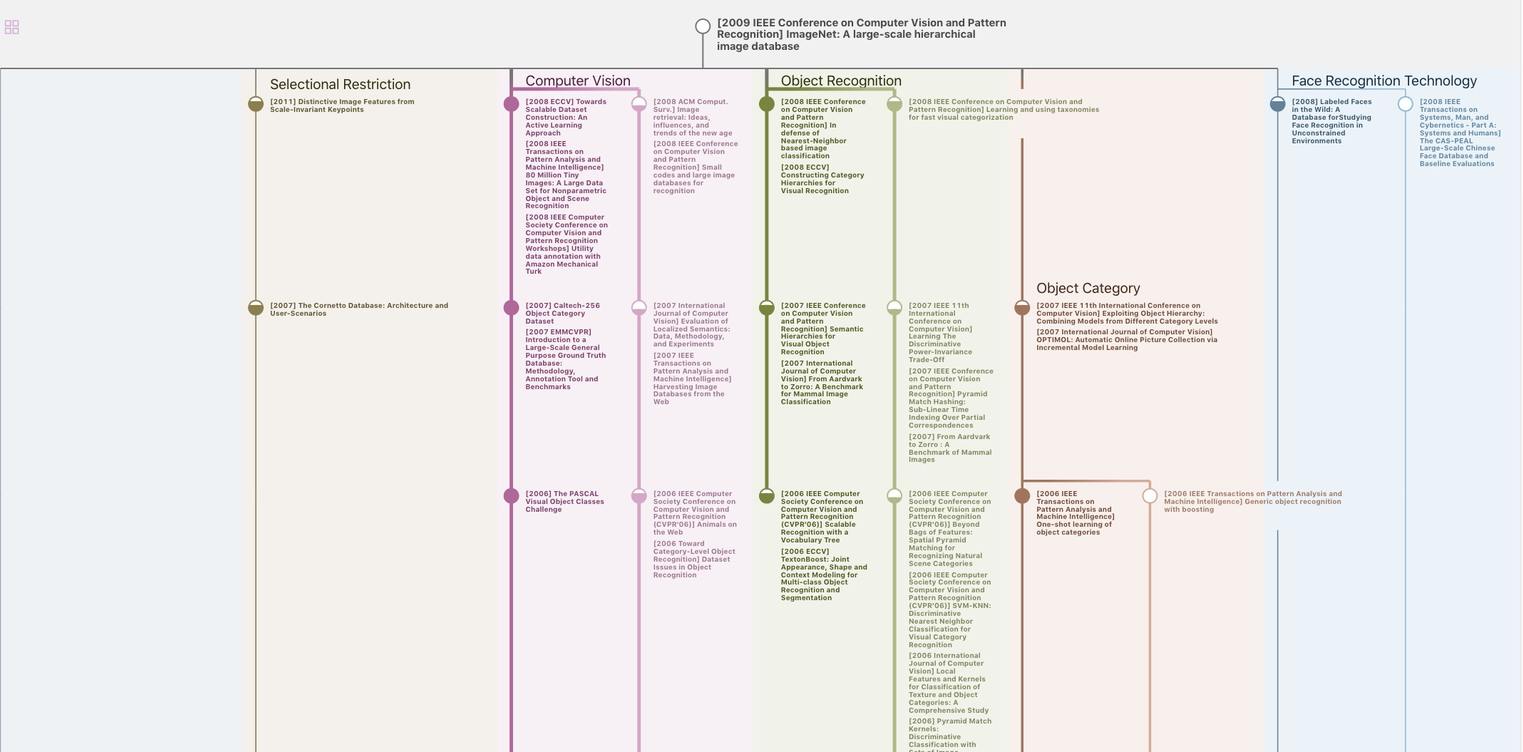
生成溯源树,研究论文发展脉络
Chat Paper
正在生成论文摘要