Universal Quantum Perceptrons for Quantum Machine Learning
arxiv(2022)
摘要
Quantum neuromorphic computing (QNC) is a subfield of quantum machine learning (QML) that capitalizes on inherent system dynamics. As a result, QNC can run on contemporary, noisy quantum hardware and is poised to realize challenging algorithms in the near term. One key element yet to be added to QNC is the characterization of the requisite dynamics for universal quantum neuromorphic computation. We address this issue by proposing a quantum equivalent to the classical perceptron, a simple mathematical model for a neuron that is the building block of various machine learning architectures. We introduce a quantum perceptron (QP) based on the analog dynamics of interacting qubits with tunable coupling constants. By adding tunable single-qubit rotations to the QP, we demonstrate that a QP can realize universal quantum computation, which contrasts sharply with the limited computational complexity of a single classical perceptron. We show that QPs are analogous to variational quantum algorithms (VQAs) familiar to the quantum machine learning community. We derive the quantum neural tangent kernel of a QP and compare the QP's trainability to the trainability of other VQAs. We discuss the advantages and drawbacks of kernel formalism. Finally, we demonstrate the effectiveness of QPs by applying them to numerous QML problems, including calculating the inner products between quantum states, entanglement witnessing, and quantum metrology.
更多查看译文
关键词
quantum,machine learning
AI 理解论文
溯源树
样例
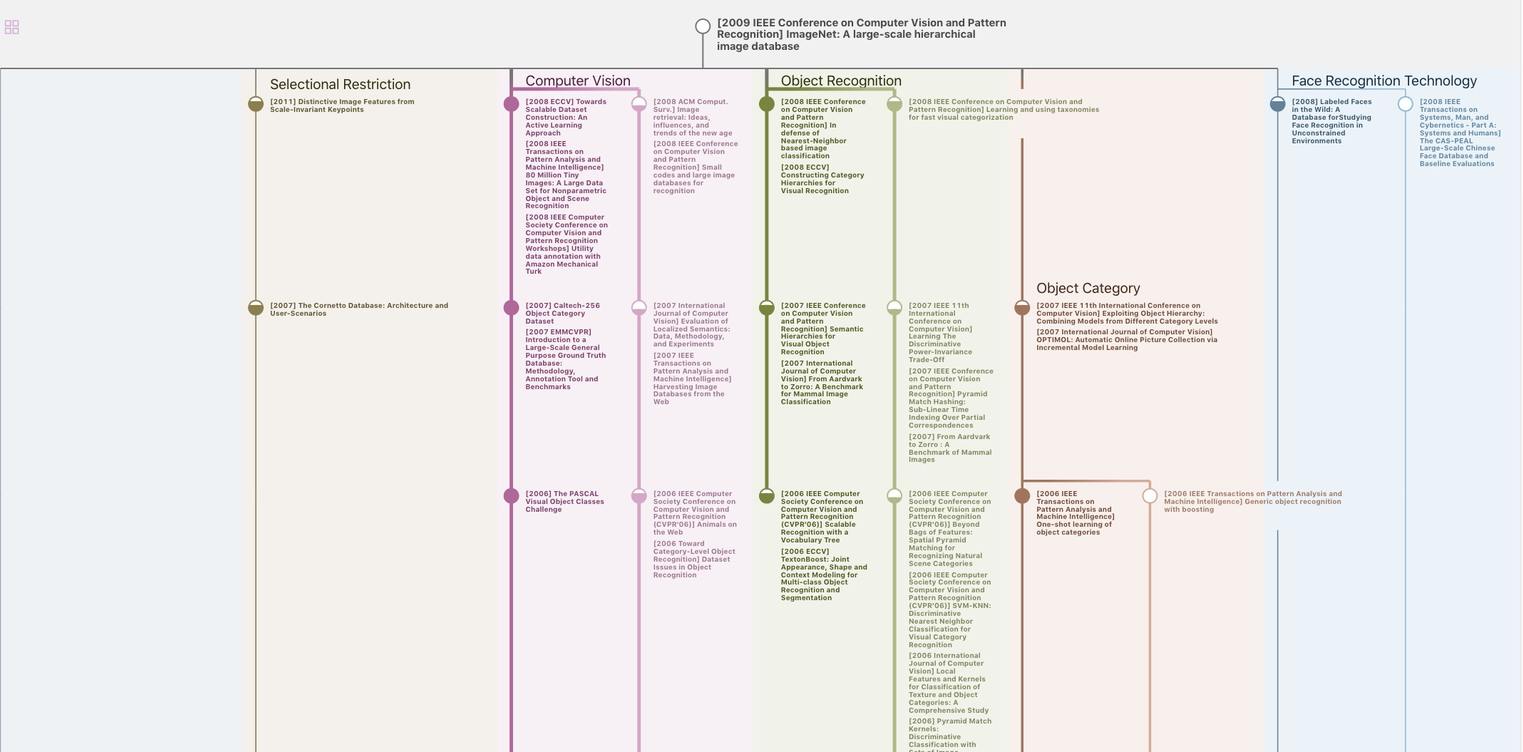
生成溯源树,研究论文发展脉络
Chat Paper
正在生成论文摘要