Exploiting In-Constraint Energy in Constrained Variational Quantum Optimization
2022 IEEE/ACM Third International Workshop on Quantum Computing Software (QCS)(2022)
摘要
A central challenge of applying near-term quantum optimization algorithms to industrially relevant problems is the need to incorporate complex constraints. In general, such constraints cannot be easily encoded in the circuit, and the quantum circuit measurement outcomes are not guaranteed to respect the constraints. Therefore, the optimization must trade off the in-constraint probability and the quality of the inconstraint solution by adding a penalty for constraint violation into the objective. We propose a new approach for solving constrained optimization problems with unconstrained, easy-to-implement quantum ansatze. Our method leverages the inconstraint energy as the objective and adds a lower-bound constraint on the in-constraint probability to the optimizer. We demonstrate significant gains in solution quality over directly optimizing the penalized energy. We implement our method in QVoice, a Python package that interfaces with Qiskit for quick prototyping in simulators and on quantum hardware.
更多查看译文
关键词
quantum approximate optimization algorithm,variational quantum eigensolver,constrained combinatorial optimization
AI 理解论文
溯源树
样例
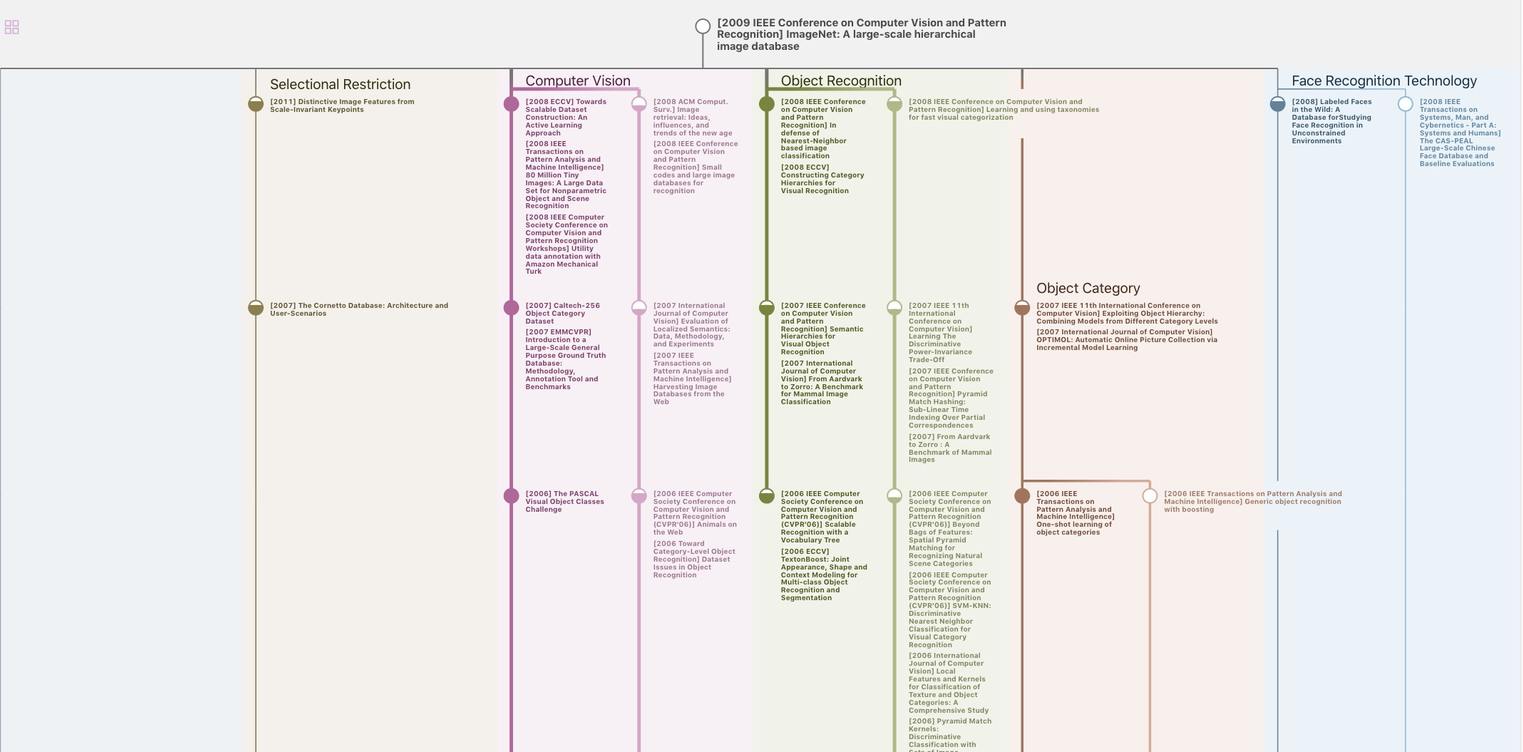
生成溯源树,研究论文发展脉络
Chat Paper
正在生成论文摘要