Large-Scale Bidirectional Training for Zero-Shot Image Captioning
arxiv(2022)
摘要
When trained on large-scale datasets, image captioning models can understand the content of images from a general domain but often fail to generate accurate, detailed captions. To improve performance, pretraining-and-finetuning has been a key strategy for image captioning. However, we find that large-scale bidirectional training between image and text enables zero-shot image captioning. In this paper, we introduce Bidirectional Image Text Training in largER Scale, BITTERS, an efficient training and inference framework for zero-shot image captioning. We also propose a new evaluation benchmark which comprises of high quality datasets and an extensive set of metrics to properly evaluate zero-shot captioning accuracy and societal bias. We additionally provide an efficient finetuning approach for keyword extraction. We show that careful selection of large-scale training set and model architecture is the key to achieving zero-shot image captioning.
更多查看译文
关键词
large-scale,zero-shot
AI 理解论文
溯源树
样例
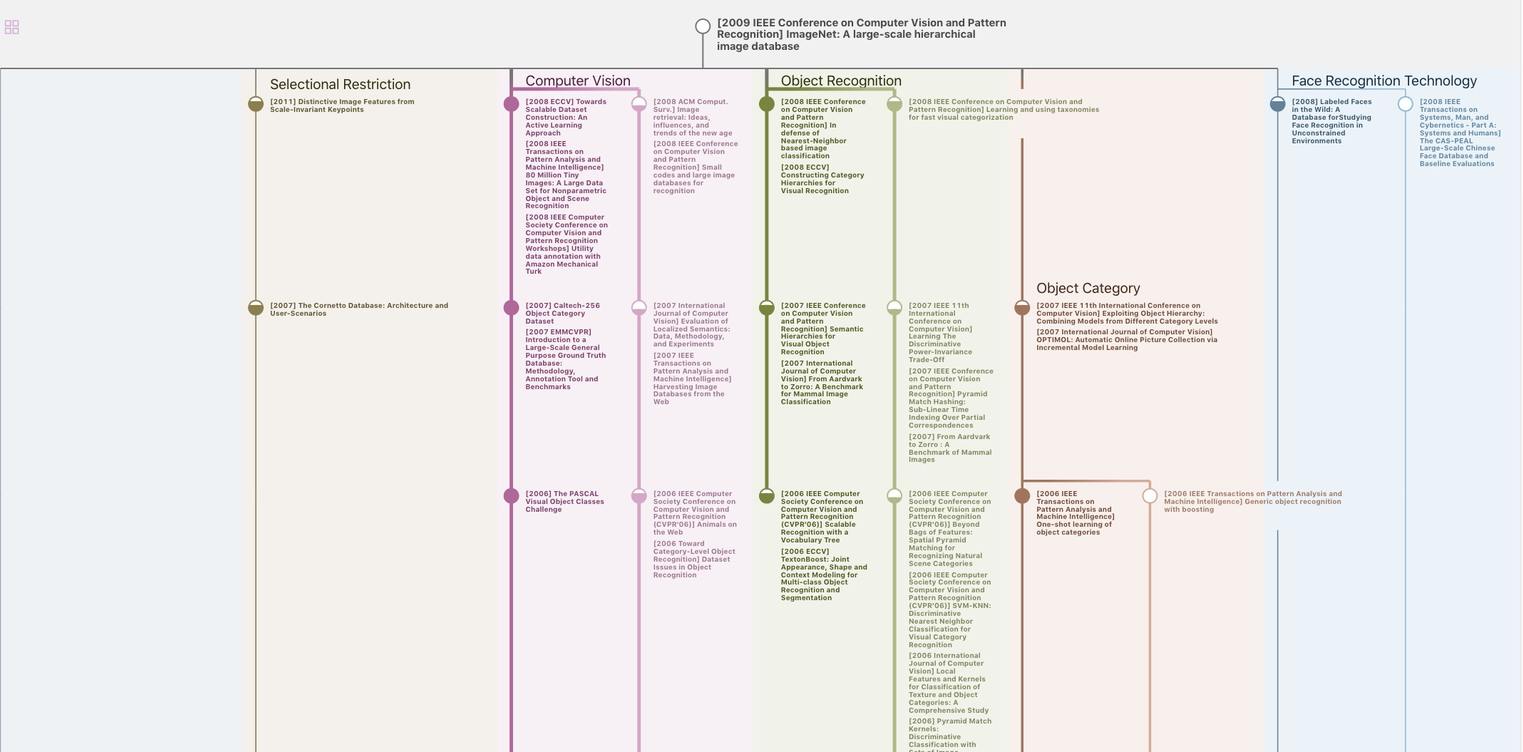
生成溯源树,研究论文发展脉络
Chat Paper
正在生成论文摘要