Deep Odometry Systems on Edge with EKF-LoRa Backend for Real-Time Indoor Positioning
2022 Workshop on Cyber Physical Systems for Emergency Response (CPS-ER)(2022)
摘要
Ubiquitous positioning for pedestrians in adverse environments has been a long standing challenge. Despite dramatic progress made by Deep Learning, multi-sensor deep odometry systems still pose a high computational cost and suffer from cumulative drifting errors over time. Thanks to the increasing computational power of edge devices, we propose a novel ubiquitous positioning solution by integrating state-of-the-art deep odometry models on edge with an EKF (Extended Kalman Filter)-LoRa backend. We carefully select and compare three sensor modalities, i.e., an Inertial Measurement Unit (IMU), a millimetre-wave (mmWave) radar, and a thermal infrared camera, and implement their deep odometry inference engines to run in real-time. A pipeline for deploying deep odometry on edge platforms with different resource constraints is proposed. We design a LoRa link for positional data backhaul and project aggregated positions of deep odometry into the global frame. We find that a simple EKF backend is sufficient for generic odometry calibration with over 34% accuracy gains against any standalone deep odometry system. Extensive tests in different environments validate the efficiency and efficacy of our proposed positioning system.
更多查看译文
关键词
Deep Learning, edge computing, ubiquitous positioning
AI 理解论文
溯源树
样例
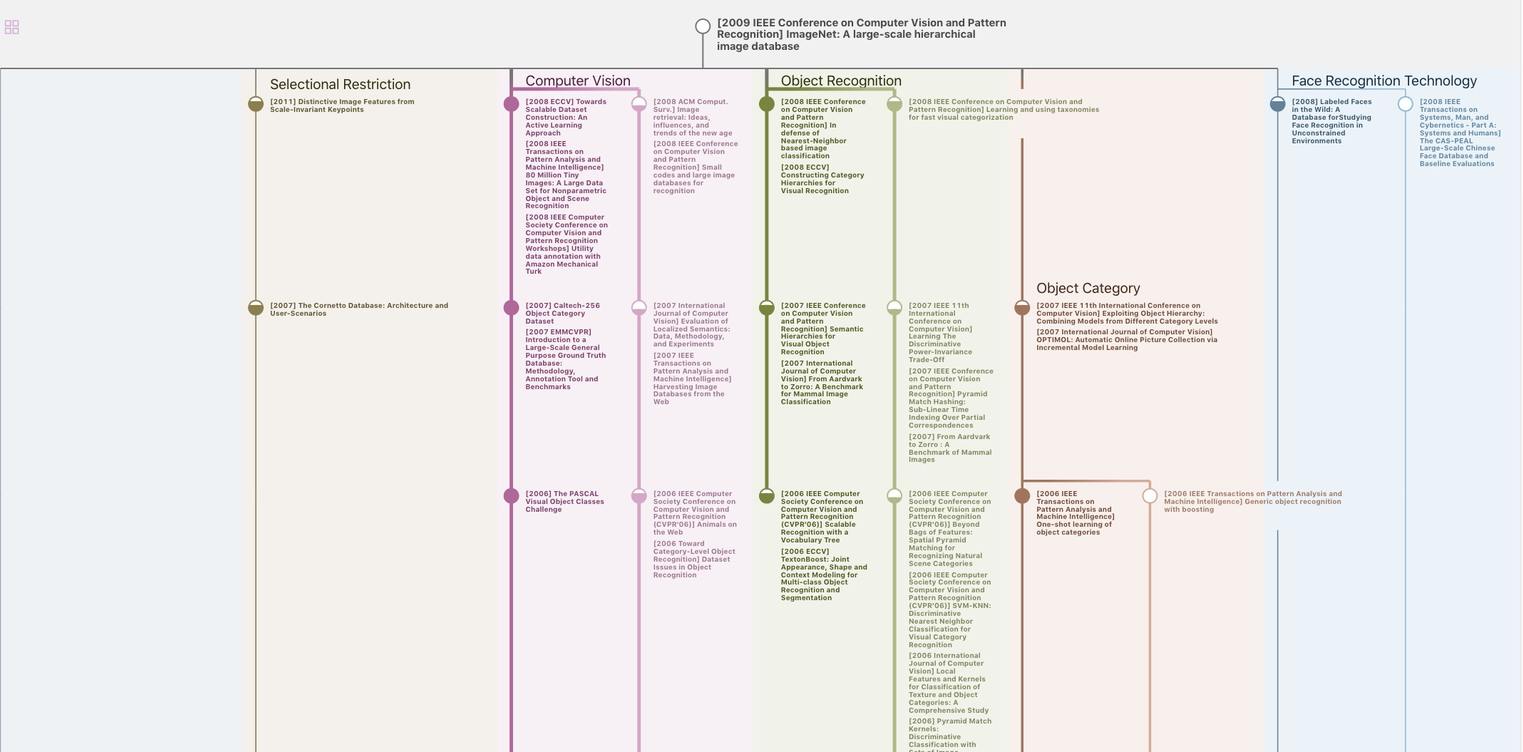
生成溯源树,研究论文发展脉络
Chat Paper
正在生成论文摘要