DeepG2P: Fusing Multi-Modal Data to Improve Crop Production
arxiv(2022)
摘要
Agriculture is at the heart of the solution to achieve sustainability in feeding the world population, but advancing our understanding on how agricultural output responds to climatic variability is still needed. Precision Agriculture (PA), which is a management strategy that uses technology such as remote sensing, Geographical Information System (GIS), and machine learning for decision making in the field, has emerged as a promising approach to enhance crop production, increase yield, and reduce water and nutrient losses and environmental impacts. In this context, multiple models to predict agricultural phenotypes, such as crop yield, from genomics (G), environment (E), weather and soil, and field management practices (M) have been developed. These models have traditionally been based on mechanistic or statistical approaches. However, AI approaches are intrinsically well-suited to model complex interactions and have more recently been developed, outperforming classical methods. Here, we present a Natural Language Processing (NLP)-based neural network architecture to process the G, E and M inputs and their interactions. We show that by modeling DNA as natural language, our approach performs better than previous approaches when tested for new environments and similarly to other approaches for unseen seed varieties.
更多查看译文
关键词
crop,multi-modal
AI 理解论文
溯源树
样例
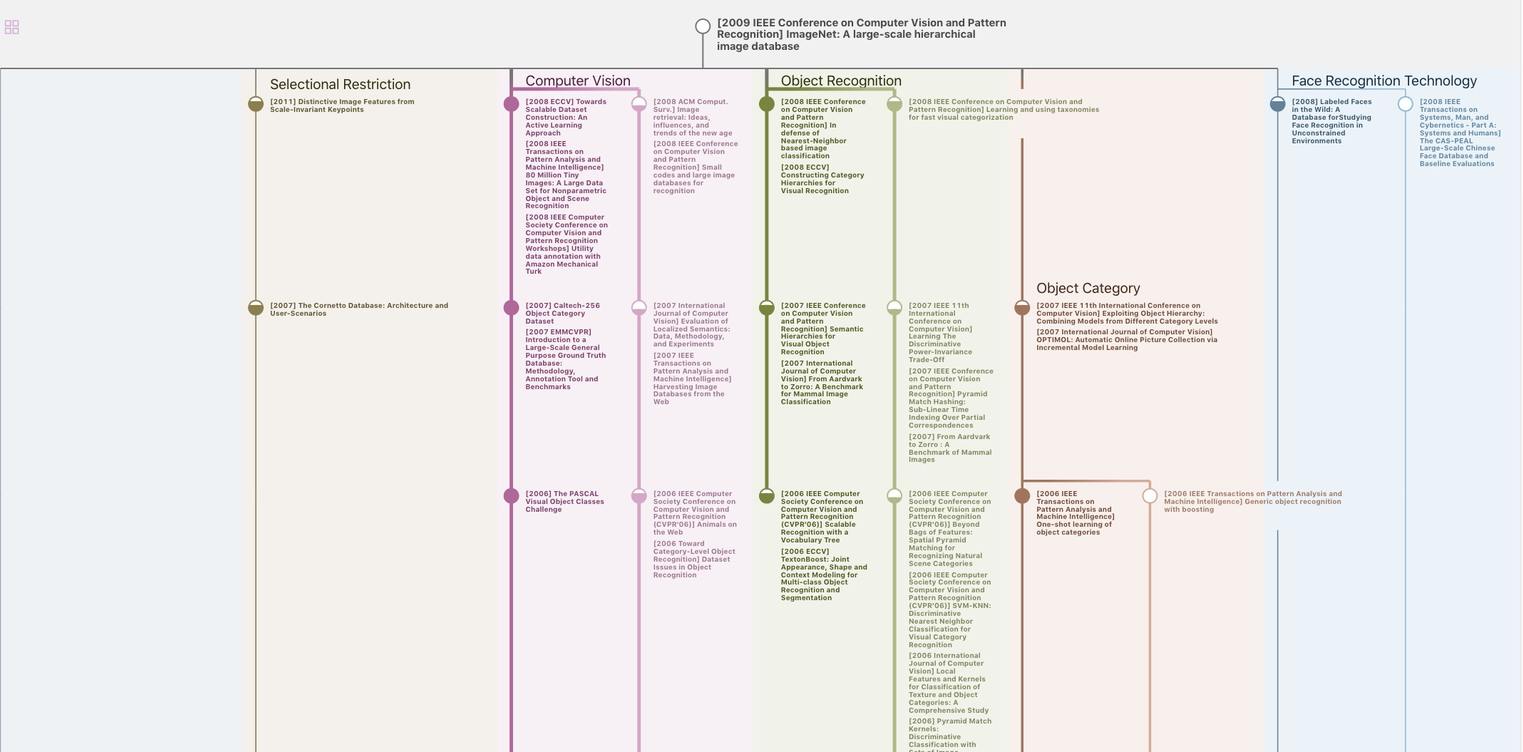
生成溯源树,研究论文发展脉络
Chat Paper
正在生成论文摘要