Scalable Multi-Agent Reinforcement Learning for Dynamic Coordinated Multipoint Clustering
IEEE Transactions on Communications(2023)
摘要
Reinforcement learning (RL) is a widely investigated intelligent algorithm and proved to be useful in the wireless communication area. However, for optimization problems in large-scale multi-cell networks whose dimension increases exponentially, it is unrealistic to employ a conventional centralized RL algorithm and make decisions for the entire network. Multi-agent RL, which allows distribute decision-making, is expected to solve the scalability problem but with performance issues due to the unknown global information, i.e., non-stationary environment. In this paper, we propose a parameter-sharing multi-agent RL for grouping decisions of coordinated multi-point in a large-scale network, where agents jointly serve users to enhance the cell-edge service. By sharing information via parameters, our theoretical and simulation results show that parameter sharing can largely benefit the multi-agent algorithm with convergence proof and convergence speed analysis. To reduce the effect of biased local heterogeneous experience, we also propose a transfer learning method for the parameter sharing process, whose performance of transfer learning algorithms is verified by the simulation results.
更多查看译文
关键词
Reinforcement learning,telecommunication,multi-agent systems,next generation networking
AI 理解论文
溯源树
样例
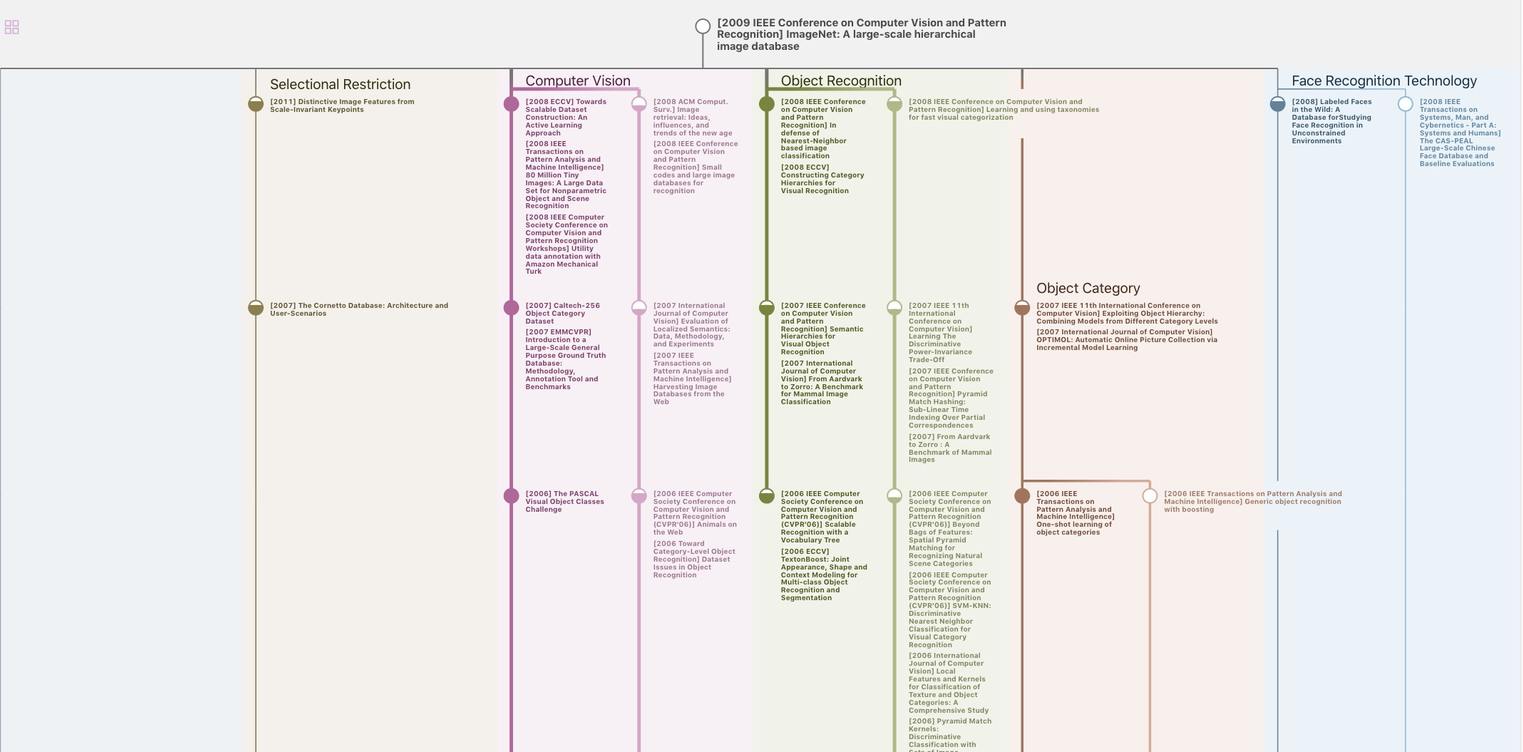
生成溯源树,研究论文发展脉络
Chat Paper
正在生成论文摘要