Efficient Compressed Ratio Estimation Using Online Sequential Learning for Edge Computing
2023 IEEE 34TH ANNUAL INTERNATIONAL SYMPOSIUM ON PERSONAL, INDOOR AND MOBILE RADIO COMMUNICATIONS, PIMRC(2023)
摘要
Owing to the widespread adoption of the Internet of Things, a vast amount of sensor information is being acquired in real time. Accordingly, the communication cost of data from edge devices is increasing. Compressed sensing (CS), a data compression method that can be used on edge devices, has been attracting attention as a method to reduce communication costs. In CS, estimating the appropriate compression ratio is important. There is a method to adaptively estimate the compression ratio for the acquired data using reinforcement learning (RL). However, the computational costs associated with existing RL methods that can be utilized on edges are often high. In this study, we developed an efficient RL method for edge devices, referred to as the actor-critic online sequential extreme learning machine (AC-OSELM), and a system to compress data by estimating an appropriate compression ratio on the edge using AC-OSELM. The performance of the proposed method in estimating the compression ratio is evaluated by comparing it with other RL methods for edge devices. The experimental results indicate that AC-OSELM demonstrated the same or better compression performance and faster compression ratio estimation than the existing methods.
更多查看译文
关键词
edge computing,data compression,IoT
AI 理解论文
溯源树
样例
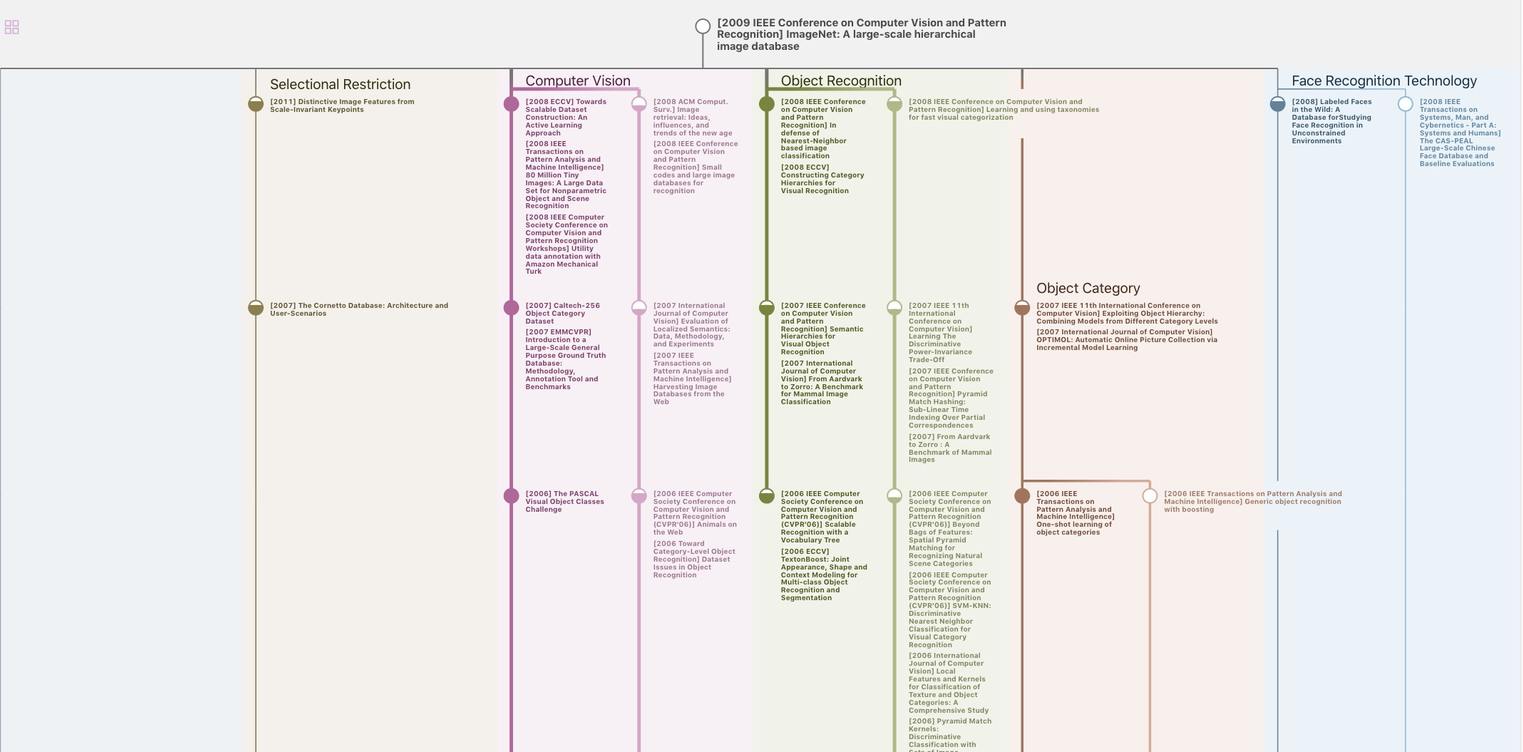
生成溯源树,研究论文发展脉络
Chat Paper
正在生成论文摘要