What Knowledge Is Needed? Towards Explainable Memory for kNN-MT Domain Adaptation
conf_acl(2022)
摘要
kNN-MT presents a new paradigm for domain adaptation by building an external datastore, which usually saves all target language token occurrences in the parallel corpus. As a result, the constructed datastore is usually large and possibly redundant. In this paper, we investigate the interpretability issue of this approach: what knowledge does the NMT model need? We propose the notion of local correctness (LAC) as a new angle, which describes the potential translation correctness for a single entry and for a given neighborhood. Empirical study shows that our investigation successfully finds the conditions where the NMT model could easily fail and need related knowledge. Experiments on six diverse target domains and two language-pairs show that pruning according to local correctness brings a light and more explainable memory for kNN-MT domain adaptation.
更多查看译文
关键词
adaptation,explainable memory,knowledge
AI 理解论文
溯源树
样例
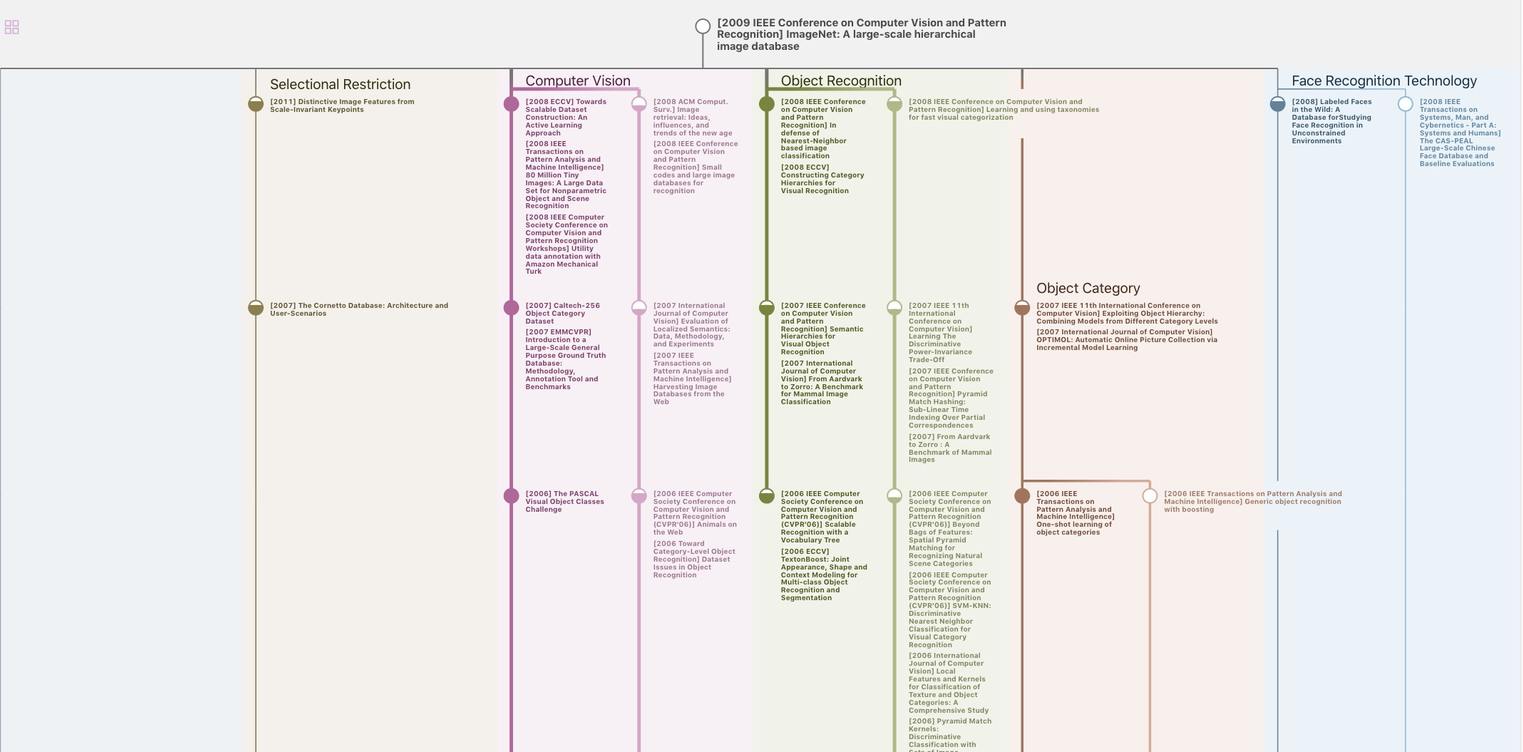
生成溯源树,研究论文发展脉络
Chat Paper
正在生成论文摘要