Domain-decomposed Bayesian inversion based on local Karhunen-Loève expansions
arxiv(2024)
摘要
In many Bayesian inverse problems the goal is to recover a spatially varying random field. Such problems are often computationally challenging especially when the forward model is governed by complex partial differential equations (PDEs). The challenge is particularly severe when the spatial domain is large and the unknown random field needs to be represented by a high-dimensional parameter. In this paper, we present a domain-decomposed method to attack the dimensionality issue and the method decomposes the spatial domain and the parameter domain simultaneously. On each subdomain, a local Karhunen-Loève (KL) expansion is constructed, and a local inversion problem is solved independently in a parallel manner, and more importantly, in a lower-dimensional space. After local posterior samples are generated through conducting Markov chain Monte Carlo (MCMC) simulations on subdomains, a novel projection procedure is developed to effectively reconstruct the global field. In addition, the domain decomposition interface conditions are dealt with an adaptive Gaussian process-based fitting strategy. Numerical examples are provided to demonstrate the performance of the proposed method.
更多查看译文
关键词
Bayesian inference,Markov chain Monte Carlo,Domain decomposition,Local KL expansions
AI 理解论文
溯源树
样例
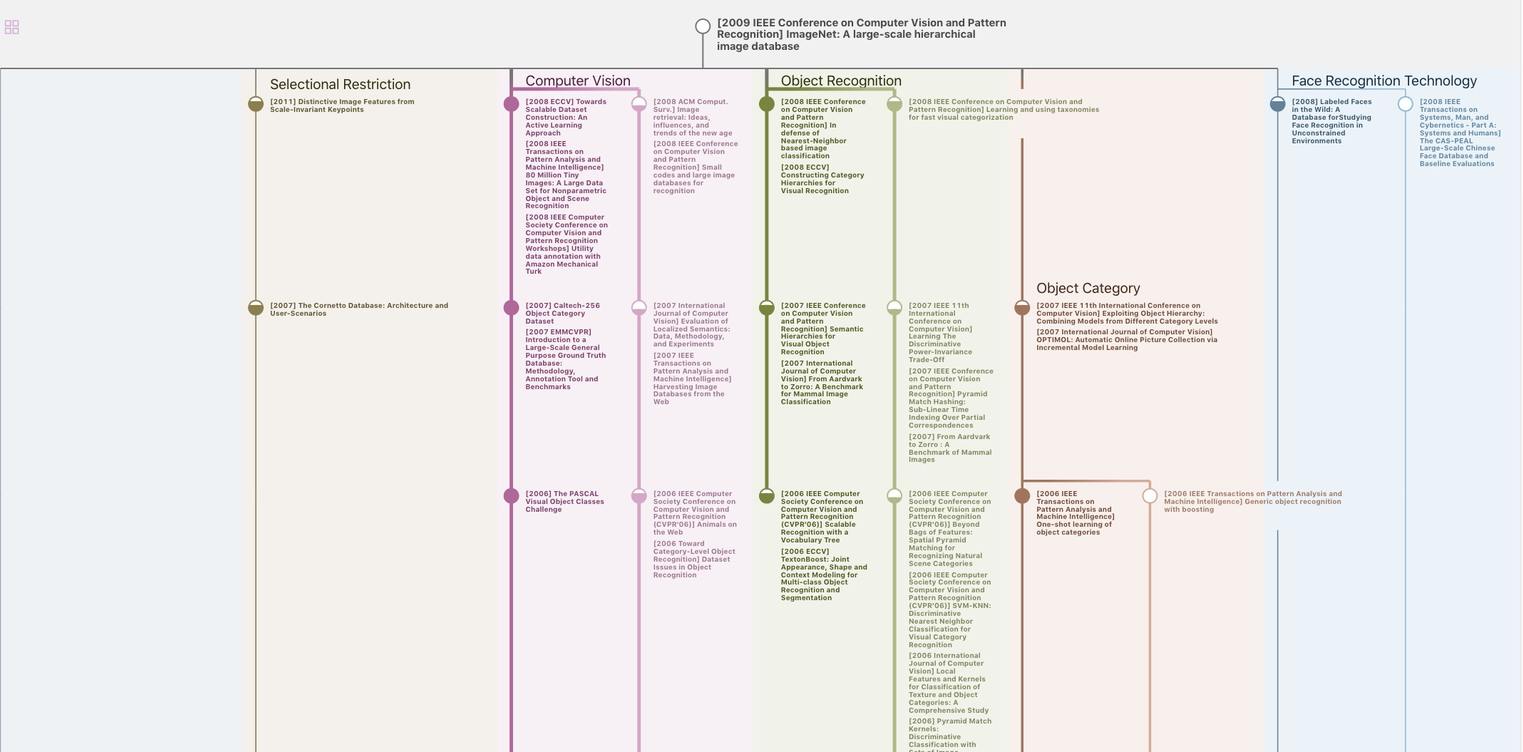
生成溯源树,研究论文发展脉络
Chat Paper
正在生成论文摘要