Estimation of surface roughness in selective laser sintering using computational models
INTERNATIONAL JOURNAL OF ADVANCED MANUFACTURING TECHNOLOGY(2022)
摘要
This study presents a comprehensive experimental dataset and a novel classification model based on Deep Neural Networks to estimate surface roughness for additive manufacturing. Many problems exist due to the very complex nature of the production process. Some focus on the production planning phase, including the nesting problem under many constraints. However, it is not possible to solve the main function without a clear understanding of the nature of the constraints. The purpose of this research is to present a method to automate the surface roughness estimation process in the production planning phase. The significance of this study is to implement a data-driven model for one of the most critical decision constraints in the nesting process. Solving this problem will automate a key decision constraint, and it might be implemented as an automated constraint module in solving the nesting problem. The proposed model focused on selective laser sintering (SLS) technology based on polyamide 12 powder applications. A comprehensive dataset is designed to simulate the behaviour of an industrial SLS manufacturing process based on a 3D positioning strategy. A set of samples with random positions are also created to test present the model’s robustness. The proposed classification model is based on Deep Neural Networks (DNN) with hyper-parameters designed for the problem. The dataset and the model provide a new user interface to estimate the surface roughness depending on the coordinates of a given product surface in an SLS production chamber and the production parameters employed in the production planning phase. The results show that the model can classify sample surfaces as “rough” or “smooth” with a very high percentage (95.8%) for the training set and with 100% for the test set. Benchmark results also show that the model outperforms other machine learning methods in classifying the surface roughness successfully on the test set.
更多查看译文
关键词
Advanced manufacturing,Additive manufacturing,Selective laser sintering,Surface roughness,Artificial Intelligence,Deep Neural Networks
AI 理解论文
溯源树
样例
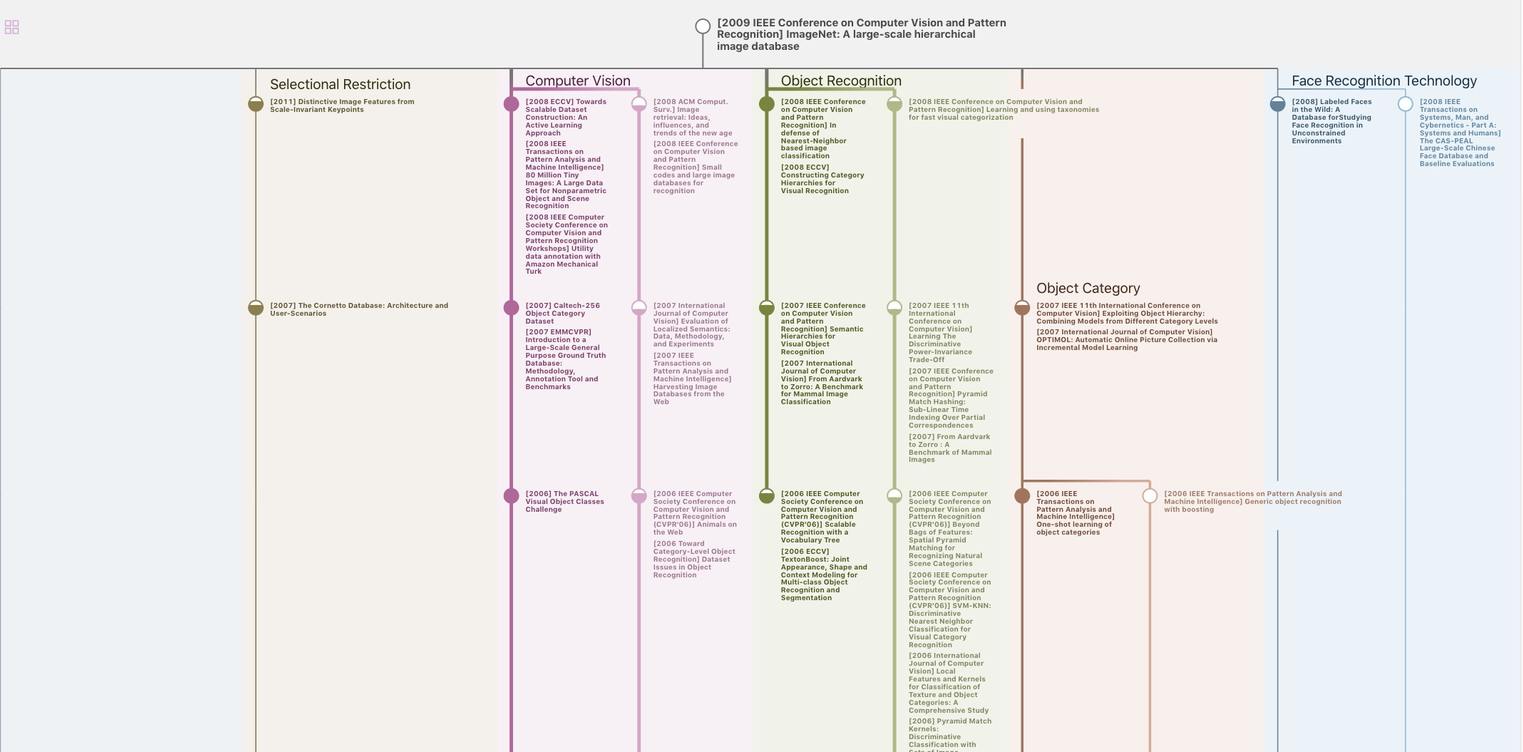
生成溯源树,研究论文发展脉络
Chat Paper
正在生成论文摘要