Can neural networks extrapolate? Discussion of a theorem by Pedro Domingos
arxiv(2023)
摘要
Neural networks trained on large datasets by minimizing a loss have become the state-of-the-art approach for resolving data science problems, particularly in computer vision, image processing and natural language processing. In spite of their striking results, our theoretical understanding about how neural networks operate is limited. In particular, what are the extrapolation capabilities of trained neural networks if any? In this paper we discuss a theorem of Domingos stating that “every machine learned by continuous gradient descent is approximately a kernel machine”. According to Domingos, this fact leads to conclude that all machines trained on data are mere kernel machines. We first extend Domingo’s result in the discrete case and to networks with vector-valued output. We then study its relevance and significance on simple examples. We find that in simple cases, the “neural tangent kernel” arising in Domingos’ theorem does provide understanding of the networks’ predictions. When the task given to the network grows in complexity, the interpolation capability of the network can be effectively explained by Domingos’ theorem, and no extrapolation capability of the network beyond its learning domain is found, even when the network’s structure would allow for it. We illustrate this fact on a classic perception theory problem: recovering a shape from its boundary.
更多查看译文
关键词
Neural networks,Neural tangent kernel,Kernel machine,Gradient descent,Machine learning,Planar topology
AI 理解论文
溯源树
样例
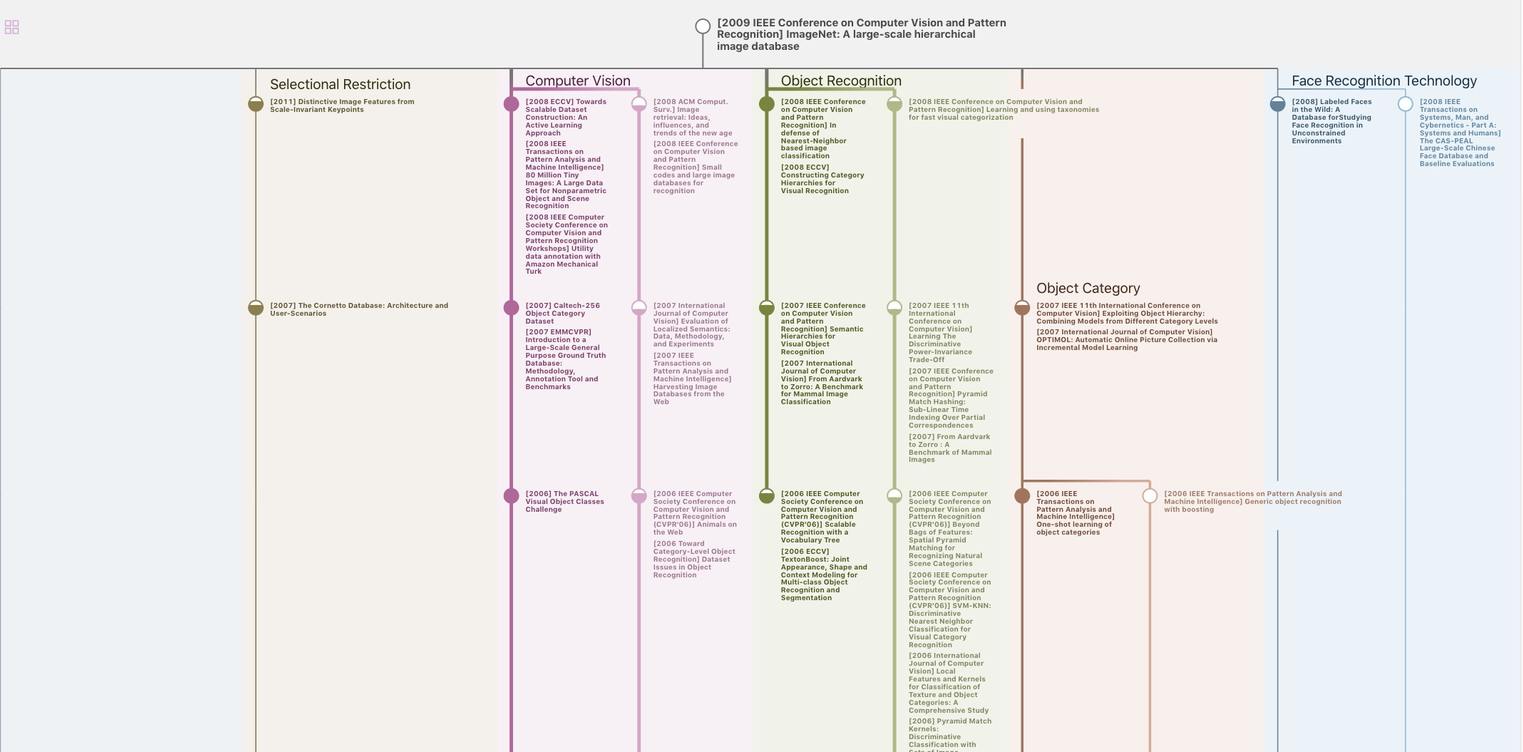
生成溯源树,研究论文发展脉络
Chat Paper
正在生成论文摘要