GoRela: Go Relative for Viewpoint-Invariant Motion Forecasting
arxiv(2022)
摘要
The task of motion forecasting is critical for self-driving vehicles (SDVs) to be able to plan a safe maneuver. Towards this goal, modern approaches reason about the map, the agents' past trajectories and their interactions in order to produce accurate forecasts. The predominant approach has been to encode the map and other agents in the reference frame of each target agent. However, this approach is computationally expensive for multi-agent prediction as inference needs to be run for each agent. To tackle the scaling challenge, the solution thus far has been to encode all agents and the map in a shared coordinate frame (e.g., the SDV frame). However, this is sample inefficient and vulnerable to domain shift (e.g., when the SDV visits uncommon states). In contrast, in this paper, we propose an efficient shared encoding for all agents and the map without sacrificing accuracy or generalization. Towards this goal, we leverage pair-wise relative positional encodings to represent geometric relationships between the agents and the map elements in a heterogeneous spatial graph. This parameterization allows us to be invariant to scene viewpoint, and save online computation by re-using map embeddings computed offline. Our decoder is also viewpoint agnostic, predicting agent goals on the lane graph to enable diverse and context-aware multimodal prediction. We demonstrate the effectiveness of our approach on the urban Argoverse 2 benchmark as well as a novel highway dataset.
更多查看译文
关键词
agent goals,agents,context-aware multimodal prediction,domain shift,driving vehicles,GoRela,heterogeneous spatial graph,leverage pair-wise relative positional encodings,map elements,map embeddings,modern approaches reason,multiagent prediction,online computation,predominant approach,reference frame,safe maneuver,scaling challenge,scene viewpoint,SDV frame,SDV visits uncommon states,shared coordinate frame,target agent,viewpoint agnostic,viewpoint-invariant motion forecasting
AI 理解论文
溯源树
样例
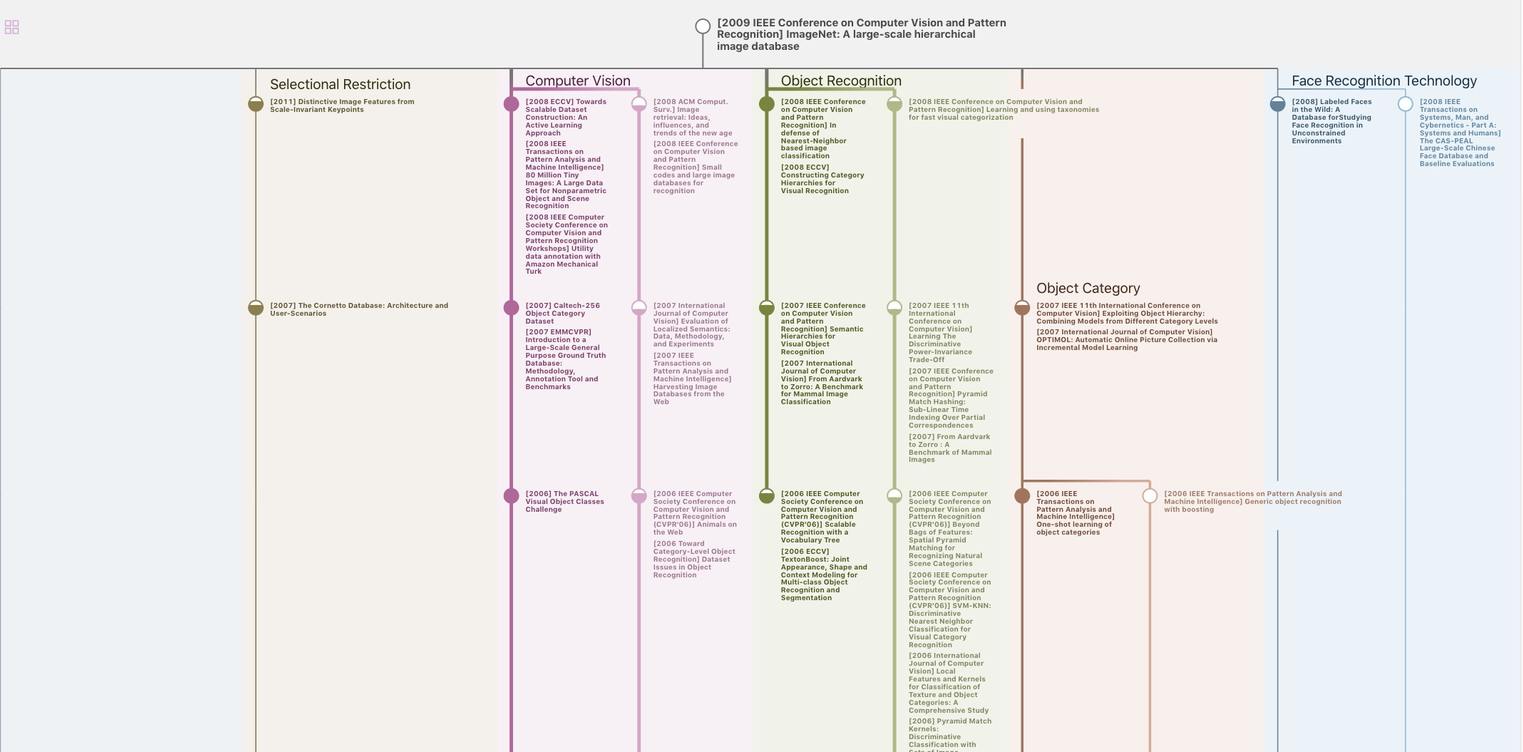
生成溯源树,研究论文发展脉络
Chat Paper
正在生成论文摘要