A Riemannian ADMM
arxiv(2022)
摘要
We consider a class of Riemannian optimization problems where the objective is the sum of a smooth function and a nonsmooth function, considered in the ambient space. This class of problems finds important applications in machine learning and statistics such as the sparse principal component analysis, sparse spectral clustering, and orthogonal dictionary learning. We propose a Riemannian alternating direction method of multipliers (ADMM) to solve this class of problems. Our algorithm adopts easily computable steps in each iteration. The iteration complexity of the proposed algorithm for obtaining an $\epsilon$-stationary point is analyzed under mild assumptions. To the best of our knowledge, this is the first Riemannian ADMM with provable convergence guarantee for solving Riemannian optimization problem with nonsmooth objective. Numerical experiments are conducted to demonstrate the advantage of the proposed method.
更多查看译文
AI 理解论文
溯源树
样例
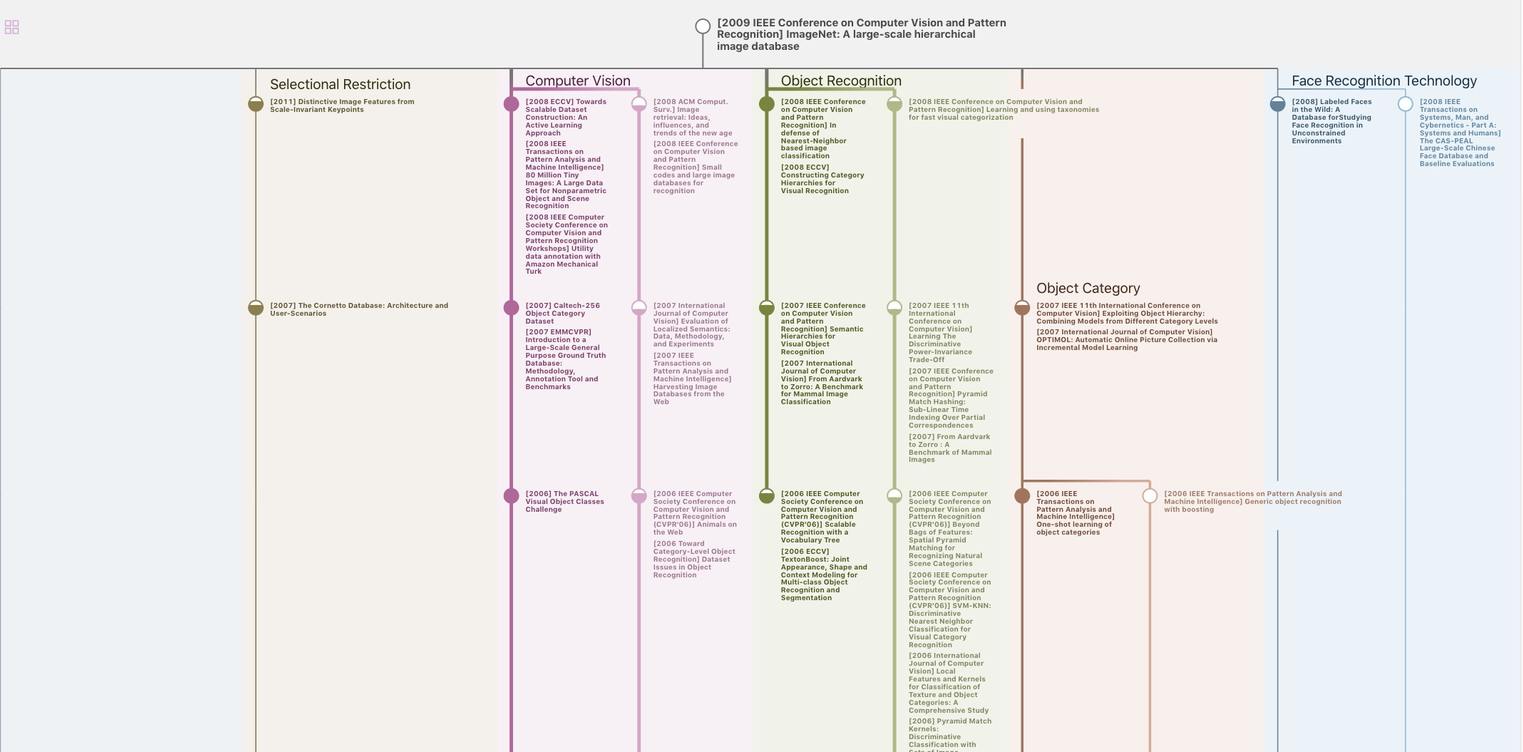
生成溯源树,研究论文发展脉络
Chat Paper
正在生成论文摘要