G2 DA: Geometry-guided dual-alignment learning for RGB-infrared person re-identification
Pattern Recognition(2023)
摘要
RGB-Infrared (IR) person re-identification aims to retrieve person-of-interest from heterogeneous cameras, easily suffering from large image modality discrepancy caused by different sensing wavelength ranges. Existing works usually minimize such discrepancy by aligning modality distribution of global features, while neglecting deep semantics and high-order structural relations within each class. This might render the misalignment between heterogeneous samples. In this paper, we propose Geometry-Guided Dual-Alignment (G2DA) learning, which yields better sample-level modality alignment for RGB-IR ReID by solving a graph-enabled distribution matching task that maximizes agreement between multi-modality node representations considering edge topology. Specifically, we covert RGB/IR images into semantic-aligned graphs, in which whole-part features and their similarities are represented by nodes and associated edges, respectively. To simultaneously implement node- and edge-wise alignment (Dual Alignment), we introduce Optimal Transport (OT) as a metric to calculate cross-modality human body matching scores. By minimizing the displacement cost across RGB-IR graphs, G2DA could learn not just modality-invariant but structurally consistent cross-modality representations. Furthermore, we advance a Message Fusion Attention (MFA) mechanism to adaptively smooth the node representations within each RGB/IR graph, effectively alleviating occlusions caused by other individuals and/or objects. Extensive experiments on two standard benchmark datasets validate the superiority of G2DA, yielding competitive performance against previous state-of-the-arts.
更多查看译文
关键词
xmlnsmml=http//wwww3org/1998/math/mathml,learning,geometry-guided,dual-alignment,rgb-infrared,re-identification
AI 理解论文
溯源树
样例
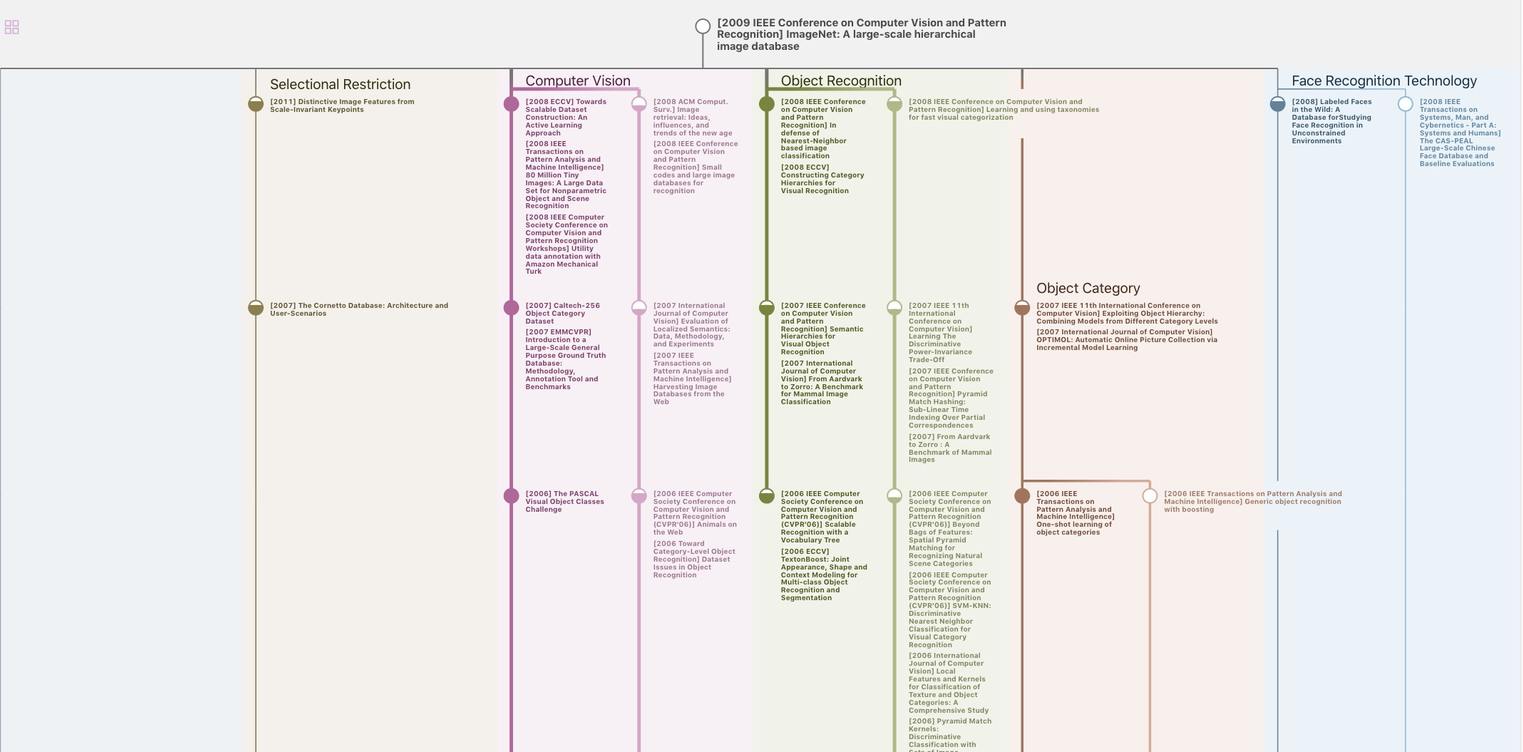
生成溯源树,研究论文发展脉络
Chat Paper
正在生成论文摘要