Cuboid-Net: A multi-branch convolutional neural network for joint space-time video super resolution
Iet Image Processing(2023)
摘要
The demand for high-resolution videos has been consistently rising across various domains, propelled by continuous advancements in societal. Nonetheless, limitations in imaging and economic factors often result in obtaining low-resolution images. The currently available space-time video super-resolution methods often fail to fully exploit the information existing within the spatio-temporal domain. To address this problem, the issue is tackled by conceptualizing the input low-resolution video as a cuboid structure. An innovative methodology called "Cuboid-Net", which incorporates a multi-branch convolutional neural network, is introduced. Cuboid-Net is designed to collectively enhance the spatial and temporal resolutions of videos, enabling the extraction of rich and meaningful information across both spatial and temporal dimensions. Specifically, the input video is taken as a cuboid to generate different directional slices as input for different branches of the network. The proposed network contains four modules, that is, a multi-branch-based hybrid feature extraction module, a multi-branch-based reconstruction module, a first-stage quality enhancement module, and a second-stage cross frame quality enhancement module for interpolated frames only. Experimental results demonstrate that the proposed method is not only effective for spatial and temporal super-resolution of video but also for spatial and angular super-resolution of light field. A multi-branch convolutional neural network (Cuboid-Net) for joint space-time video super resolution is proposed. Specifically, the input video is taken as a cuboid to generate different directional slices as input for different branches of the network.image
更多查看译文
关键词
image enhancement,image resolution,video signal processing
AI 理解论文
溯源树
样例
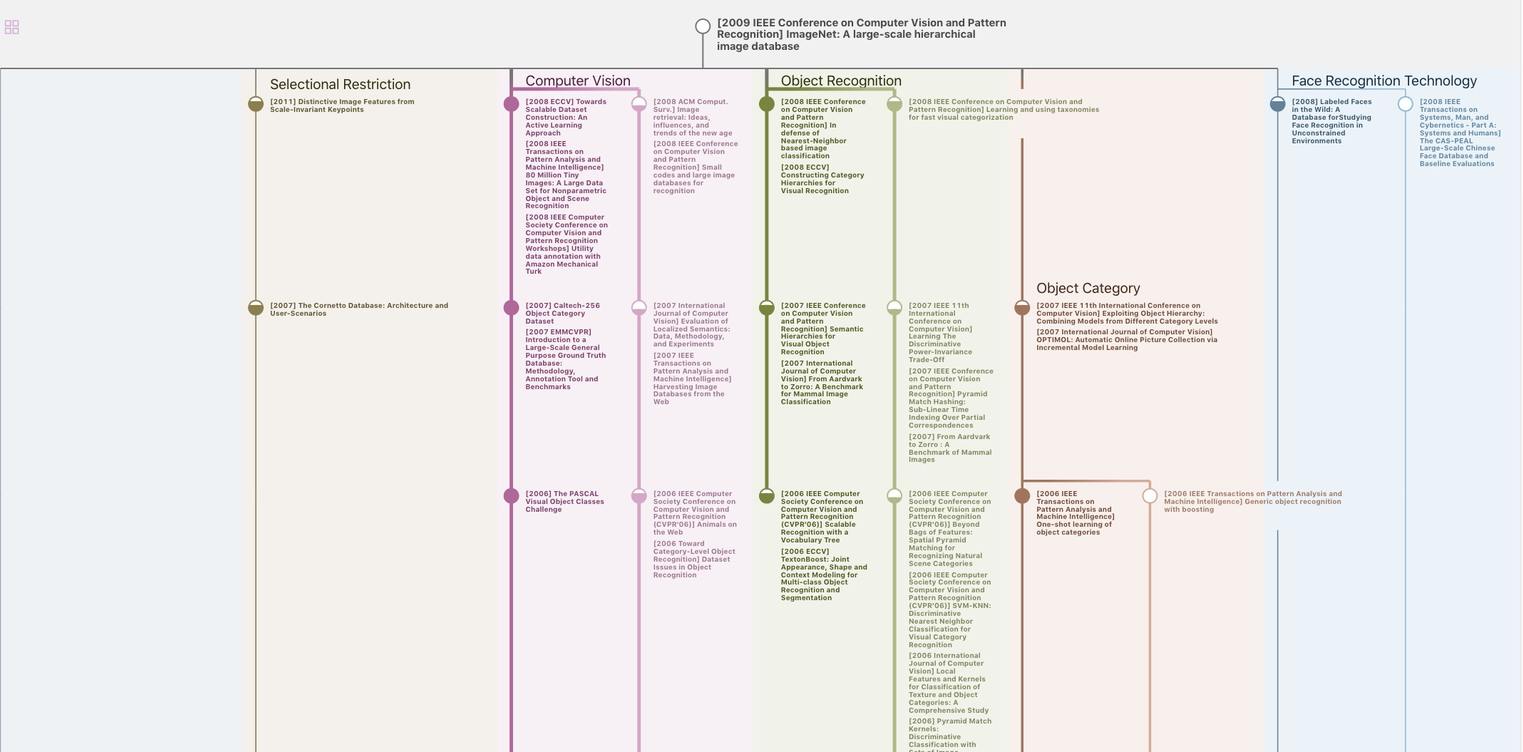
生成溯源树,研究论文发展脉络
Chat Paper
正在生成论文摘要