Active Learning for Individual Data via Minimal Stochastic Complexity
2022 58th Annual Allerton Conference on Communication, Control, and Computing (Allerton)(2022)
摘要
Modern machine learning systems require massive amounts of labeled training data in order to achieve high accuracy rates. Active learning uses feedback to label the most informative data points and significantly reduces the labeling effort. Many heuristics for selecting data points have been developed in recent years which are usually tailored to a specific task and a general unified framework is lacking. In this work, the individual data setting is considered and an active learning criterion is proposed. In this setting the features and labels, both in the training and the test, are specific individual, deterministic quantities. Motivated by connections between source coding and minimax learning, the proposed criterion attempts to find data points which minimize the average Predictive Normalized Maximum Likelihood (pNML) on the unlabeled test set. It is shown using a real data set that the proposed criterion performs better than other active learning criteria.
更多查看译文
关键词
deterministic quantities,source coding,minimax learning,unlabeled test set,data set,active learning criteria,minimal stochastic complexity,modern machine learning systems,labeled training data,high accuracy rates,informative data points,labeling effort,general unified framework,individual data setting,active learning criterion,specific individual quantities
AI 理解论文
溯源树
样例
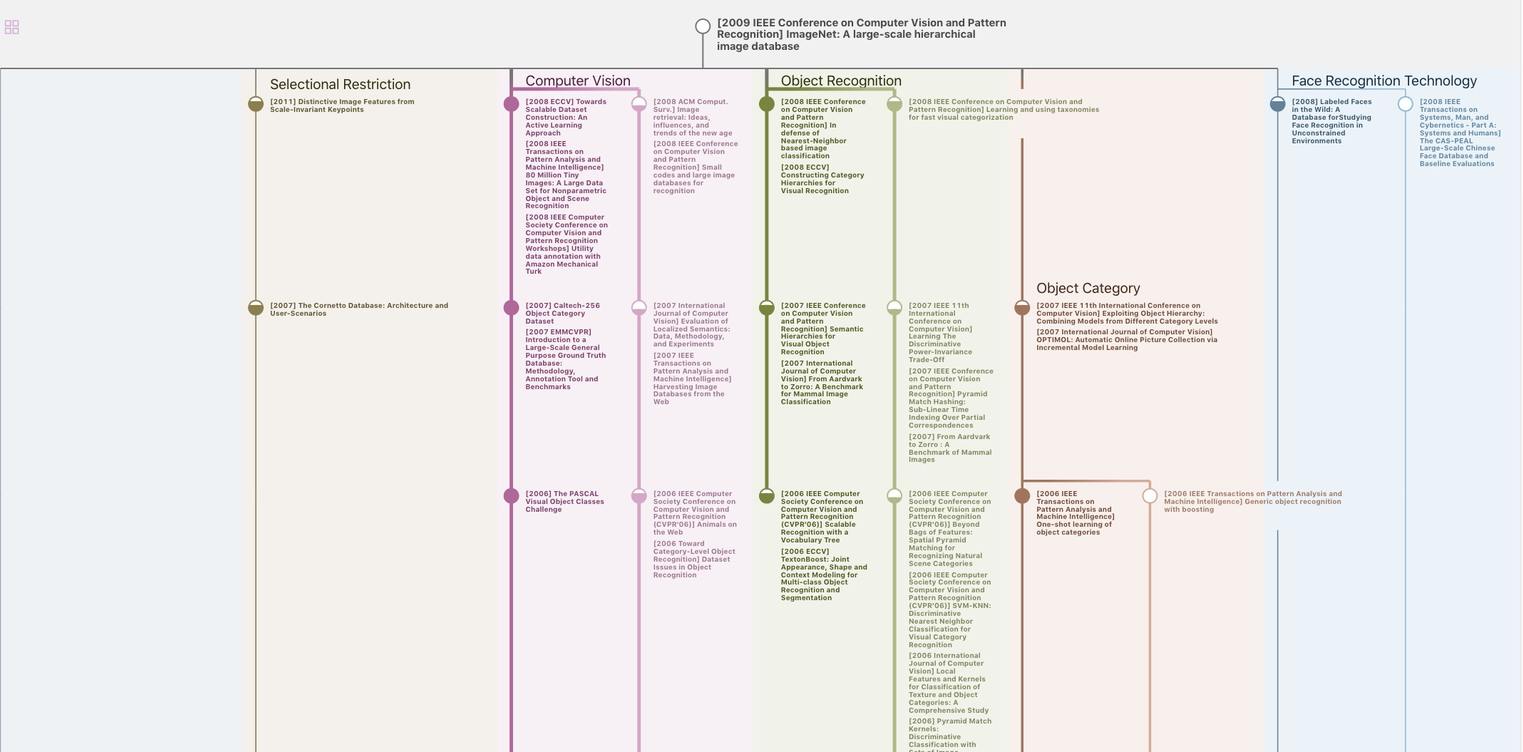
生成溯源树,研究论文发展脉络
Chat Paper
正在生成论文摘要