COVID-19 Detection Exploiting Self-Supervised Learning Representations of Respiratory Sounds
2022 IEEE-EMBS International Conference on Biomedical and Health Informatics (BHI)(2022)
摘要
In this work, we focus on the automatic detection of COVID-19 patients from the analysis of cough, breath, and speech samples. Our goal is to investigate the suitability of Self-Supervised Learning (SSL) representations extracted using Wav2Vec 2.0 for the task at hand. For this, in addition to the SSL representations, the models trained exploit the Low-Level Descriptors (LLD) of the eGeMAPS feature set, and Mel-spectrogram coefficients. The extracted representations are analysed using Convolutional Neural Networks (CNN) reinforced with contextual attention. Our experiments are performed using the data released as part of the Second Diagnosing COVID-19 using Acoustics (DiCOVA) Challenge, and we use the Area Under the Curve (AUC) as the evaluation metric. When using the CNNs without contextual attention, the multi-type model exploiting the SSL Wav2Vec 2.0 representations from the cough, breath, and speech sounds scores the highest AUC, 80.37 %. When reinforcing the embedded representations learnt with contextual attention, the AUC obtained using this same model slightly decreases to 80.01 %. The best performance on the test set is obtained with a multi-type model fusing the embedded representations extracted from the LLDs of the cough, breath, and speech samples and reinforced using contextual attention, scoring an AUC of 81.27 %.
更多查看译文
关键词
COVID-19 Detection,Respiratory Diagnosis,Paralinguistics,Self-Supervised Representations,Healthcare
AI 理解论文
溯源树
样例
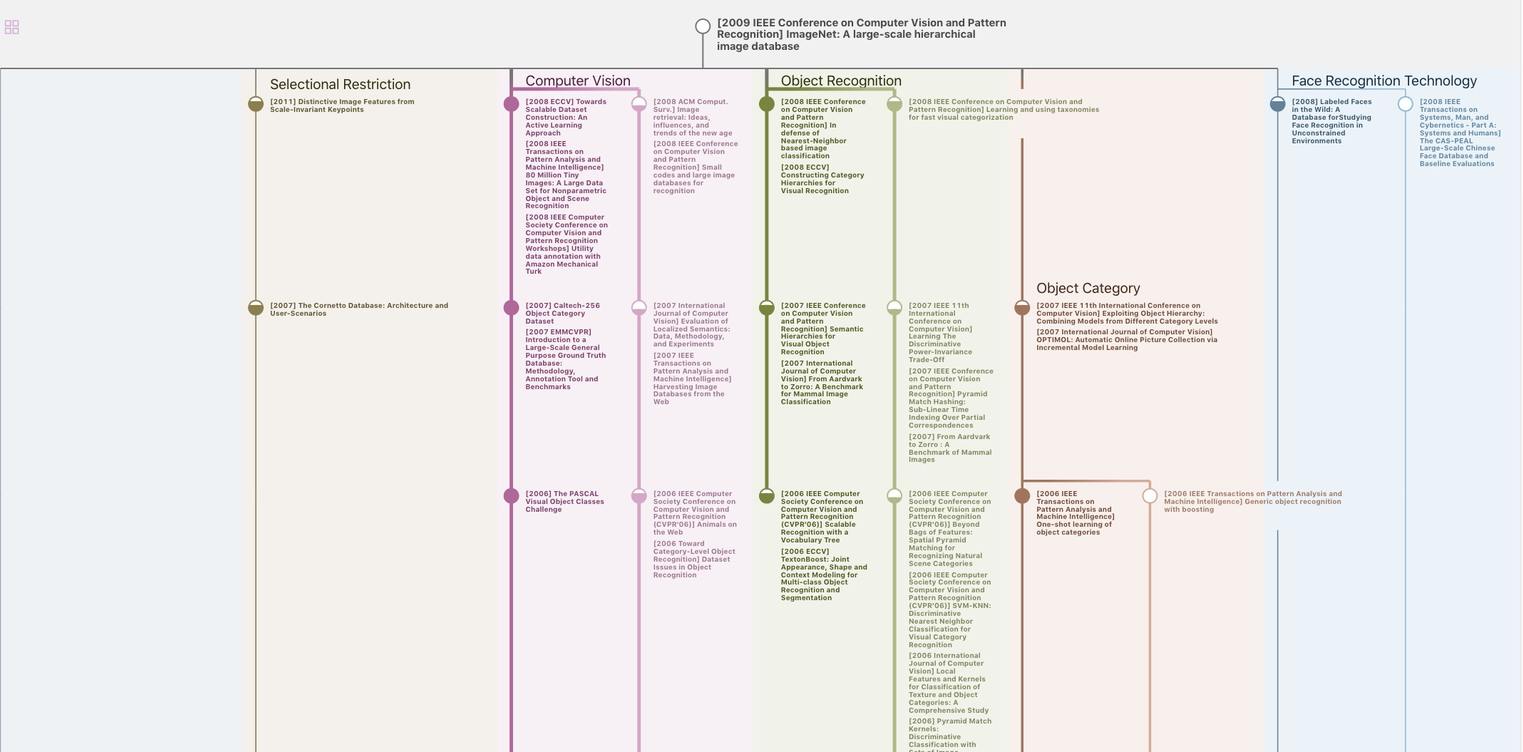
生成溯源树,研究论文发展脉络
Chat Paper
正在生成论文摘要