Estimating the Carbon Footprint of BLOOM, a 176B Parameter Language Model
JOURNAL OF MACHINE LEARNING RESEARCH(2023)
摘要
Progress in machine learning (ML) comes with a cost to the environment, given that training ML models requires computational resources, energy and materials. In the present article, we aim to quantify the carbon footprint of BLOOM, a 176-billion parameter language model, across its life cycle. We estimate that BLOOM's final training emitted approximately 24.7 tonnes of CO2eq if we consider only the dynamic power consumption, and 50.5 tonnes if we account for all processes ranging from equipment manufacturing to energy-based operational consumption. We also carry out an empirical study to measure the energy requirements and carbon emissions of its deployment for inference via an API endpoint receiving user queries in real-time. We conclude with a discussion regarding the difficulty of precisely estimating the carbon footprint of ML models and future research directions that can contribute towards improving carbon emissions reporting.
更多查看译文
关键词
carbon footprint,language modeling,life cycle assessment,machine learning
AI 理解论文
溯源树
样例
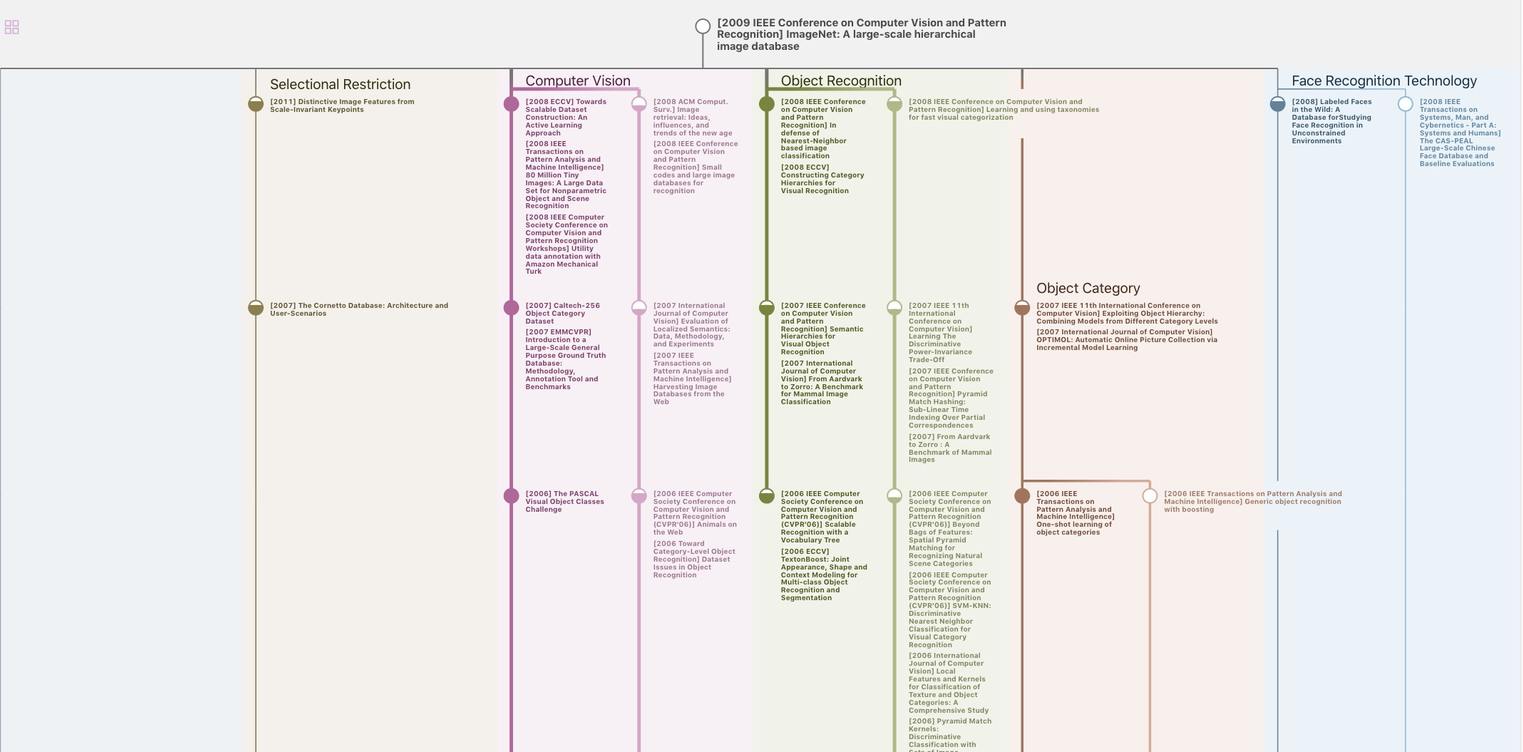
生成溯源树,研究论文发展脉络
Chat Paper
正在生成论文摘要