Soft-Output Signal Detection for Cetacean Vocalizations Using Spectral Entropy, K-Means Clustering and the Continuous Wavelet Transform
arxiv(2022)
摘要
Underwater acoustic monitoring systems record many hours of audio data for marine research, making fast and reliable non-causal signal detection paramount. Such detectors assist in reducing the amount of labor required for signal annotations, which often contain large portions devoid of signals. Cetacean vocalization detection based on spectral entropy is investigated as a means of vocalization discovery. Previous techniques using spectral entropy (SE) mostly consider time-frequency enhancement of the entropy measure, and utilize the STFT as its time-frequency (TF) decomposition. SE methods also requires the user to set a detection threshold manually, which call for knowledge of the produced entropy measures. This paper considers median filtering as a simple, effective way to provide temporal stabilization to the entropy measure, and considers the CWT as an alternative TF decomposition. K-means clustering is used to determine the threshold required to accurately separate the signal/no-signal entropy measures, resulting in a one-dimensional, two-class classification problem. The class means are used to perform pseudo-probabilistic soft class assignment, which is a useful metric in algorithmic development. The effect of median filtering, signal-to-noise ratio and the chosen TF decomposition are investigated. The proposed method shows a significant improvement in detection accuracy and specificity, while also providing a more interpretable detection threshold setting via soft class assignment.
更多查看译文
关键词
Cetacean vocalization,Spectral entropy,K -means,Signal detection,Soft classification,Continuous wavelet transform
AI 理解论文
溯源树
样例
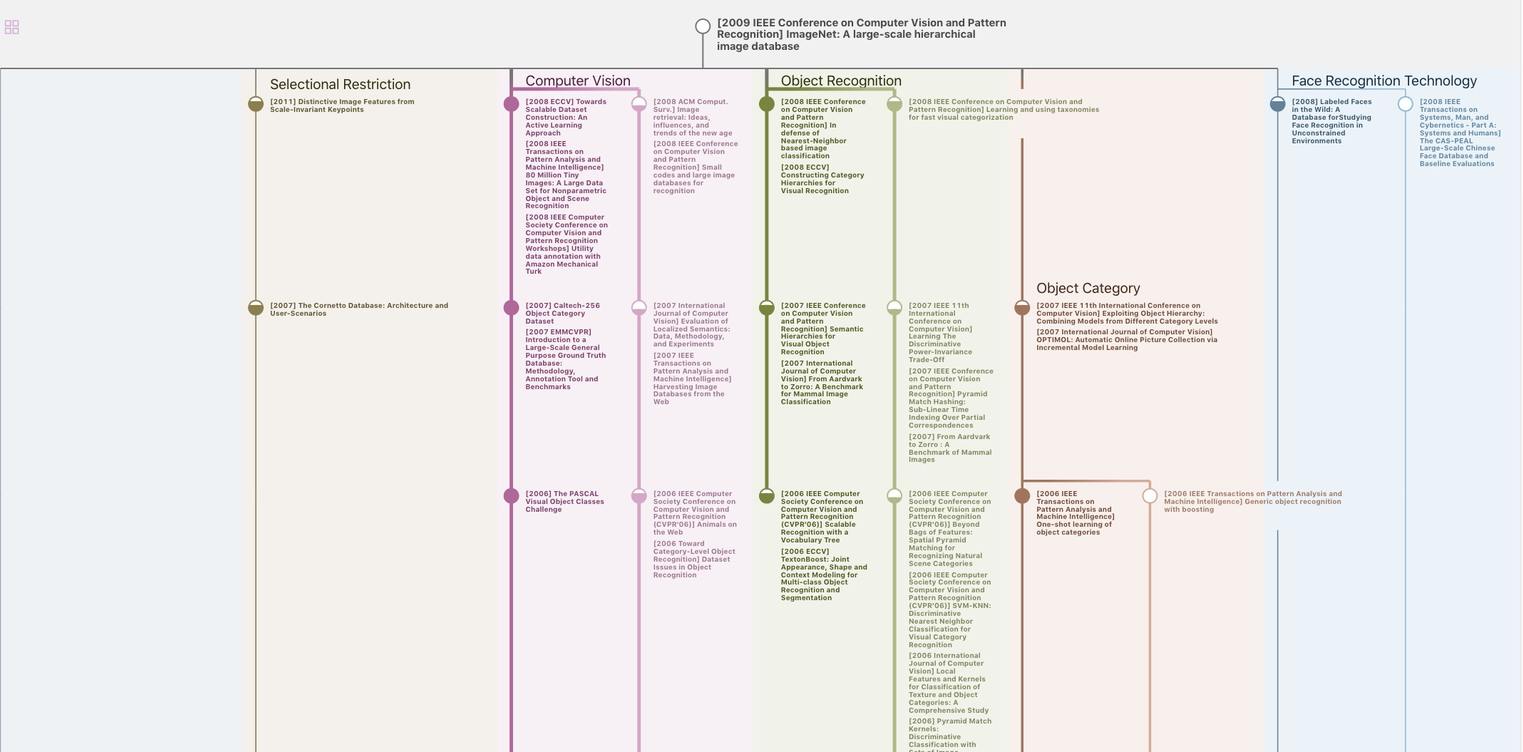
生成溯源树,研究论文发展脉络
Chat Paper
正在生成论文摘要